Why most SIEM Solutions are Failing us
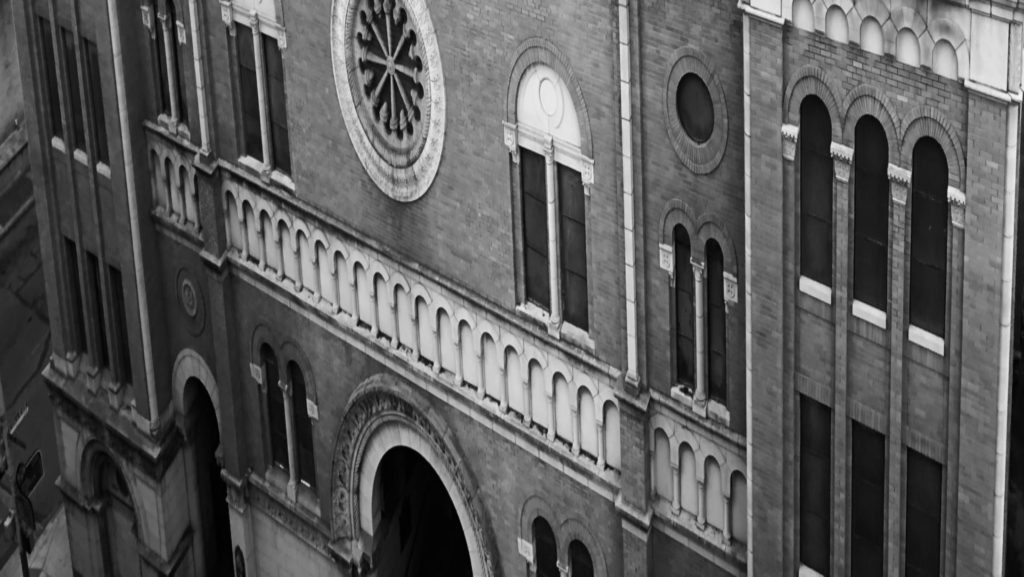
Security Information Event Managers (SIEM) and like Data Science-based automated solutions continue to fail against a broad and complex array of cyber-threats. This article is one of several that will focus on why we keep losing the cyber-war
Introduction
In fields of study that rely upon information and the use of predictive data analytics, there is a common theme for these venues failing to incorporate external or heterogeneous datasets to protect their respective networks and data (Nagrecha & Chawla, 2016; Pham, 2018; Weng, 2017; Zuech, Khoshgoftaar, & Wald, 2015). External data exists outside the local IT environment; it is exterior to the computer network; however, it can be leveraged to improve an organization’s situational awareness and ability to respond to cyber-threats more effectively (Galloppo & Previati, 2014; Hassani & Renaudin, 2018; Nagrecha & Chawla, 2016; Schroer, 2019; Weng, 2017).
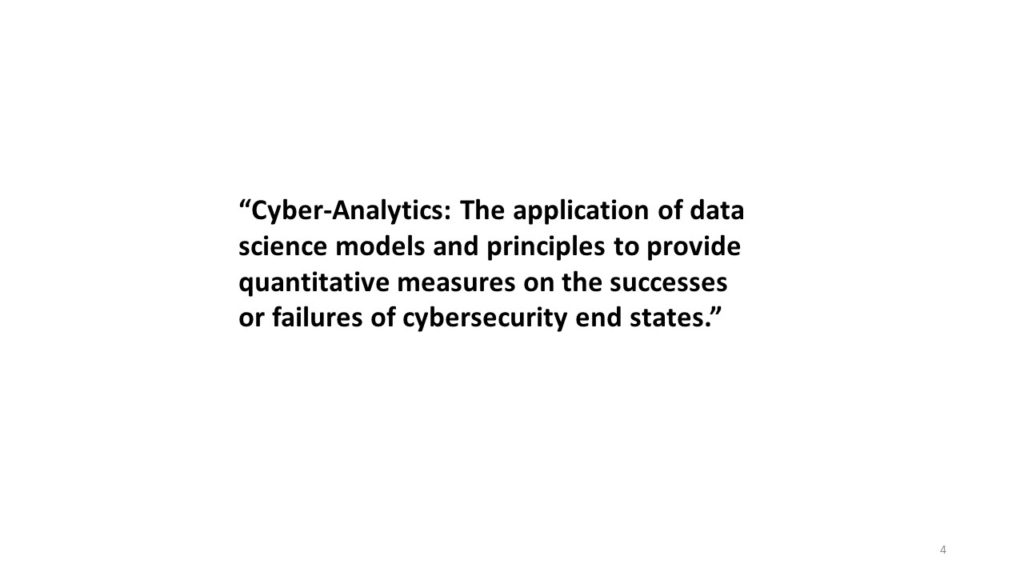
As Weng (2017) describes in his dissertation on the topic concerning better stock market predictions, he offers that the “use of external data sources along with traditional metrics leads to improve[d]…prediction performance” (Weng, 2017, p. ii.). The objective of this article is to highlight this shortcoming and suggest a practical path forward for organizations reliant on Predictive Analytics (PA) in the area of cybersecurity protections (Anitha & Patil, 2018; Lee, 2015; Oltramari & Kott, 2018).
Corporations, to include Defense Industrial Base (DIB) businesses, do not effectively use external datasets to supplement their IT network defenses against threats in cyberspace. (Nagrecha & Chawla, 2016; Zuech et al., 2015). The DIB provides contract goods and services to the Department of Defense (DOD); however, they must also balance national security protection of sensitive data while ensuring their economic stability (Hensel, 2016). These industries face growing demands to protect sensitive information and secure their networks from cyber-threat nation-state actors as well as cyber-terrorists and cyber-criminal groups (Starks, 2019; Starr, 2015).
The objective of this article is to identify a potential root-cause break-down in cybersecurity defense mechanisms such as SIEMs, smart firewalls, and Intrusion Detection Systems (IDS)
.
Background
Cybersecurity weaknesses. External data can be used to create more effective protection of IT networks (Rodriguez & Da Cunha, 2018). This information may include, for example, government indicators of threats in machine-readable formats or honeypots that are designed to identify threat Tactics, Techniques, and Procedures (TTP) to include the collection of associated attack statistics (Kumar & Verma, 2017). Industry has done an overall poor job in leveraging heterogeneous data sources and repositories and has suffered from regular attacks by cyber-threats (Zuech et al., 2015; Starks, 2019; Starr, 2015).
Organizations do not only have challenges at the data but higher-level system-to-system level. “Compounding the problem further, existing IT security systems seldom integrate across a wide spectrum of an organizations’ information systems” (Zuech et al., 2015). There are many avenues for cyber-threats to attack critical IT infrastructures (Starks, 2019; Starr, 2015). While this article only focuses at the data level, there are other “layers” that too must be better defended against cyber-attacks.
Data science acceptance and the Artificial Neural Network. “Organizations are rapidly embracing data science to inform decision making,” and to protect its valuable virtual intellectual property and trade secrets (Nagrecha & Chawla, 2016, p. 1). There are many statistical and newly burgeoning data modeling and predictive capabilities arising from the field of data science to include the use of Artificial Intelligence (AI) solutions (Wilner, 2018).
AI affords better decision-making and is designed to improve the overall effectiveness of an organization (Gupta & Rani, 2018; Halladay, 2013; K & Shivakumar, 2014). While not a perfect solution, the growth of data science’s Big Data progress and the leveraging of these large datasets offers many possible means to thwart the recurring attacks by cyber-threats (K & Shivakumar, 2014; Rodriguez & Da Cunha, 2018; Starks, 2019; Starr, 2015).
Problem Statements
General problem. The general problem is that current AI-based solutions use audit log generated data from end-point devices from within an IT environment. The exclusion of external data has been identified in academic studies as a gap in an organization’s ability to effectively apply PA within their respective private or public sector domains (Nagrecha & Chawla, 2016; Hu, Gnatyuk, V., Sydorenko, Odarchenko, & Gnatyuk, S., 2017; Zuech & Wald, 2015). Internal data only allows cyber-defenders to see what is occurring within their IT environment, and not what other attack types are occurring beyond its IT network security perimeters.
Columbus (2019) identifies ten major cybersecurity firms that are applying AI-based solutions to enhance cybersecurity protections. Statistical analysis of Columbus’ (2019) article identifies that at least 70% are focused only on end-point interior detection using audit logs of their localized IT environments Internet traffic (Columbus, 2019).
End-point detection is based upon threat characteristics identified and processed within the local IT architecture; these solutions are only fixated on threats that have already penetrated the organizational network. Current commercial solutions are limited to internal network traffic and do not rely on any future or identified threats peripheral to the security perimeters (Columbus, 2019; Schroer, 2019).
Specific problem. The specific problem is that external or heterogeneous data have not been incorporated in current predictive cybersecurity analytic models to include the defense sector (Lee, 2015; Nagrecha & Chawla, 2016). Analogous studies of the medical and stock market arenas, respectively, identify this as a significant shortfall in PA (Pham, 2018; Weng, 2017). “When there are external data sources providing population-level information about the variables, it is desirable to use such information” (Pham, 2018, p. i.).
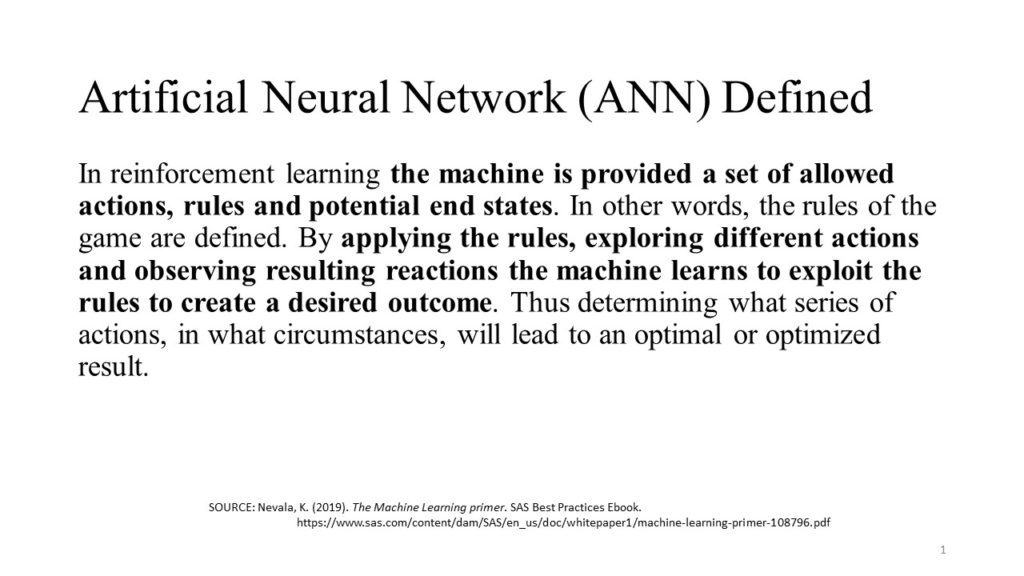
Path Forward
The suggested and anticipated solution lies with effective integration of both the internal and external data collected (Nagrecha & Chawla, 2016; Zuech et al., 2015). This root-cause failure recognition is a likely first-step avenue of approach for both the cybersecurity and data science to merge their capabilities in a concerted effort to protect their respective IT infrastructures.
Extensive Reference List
Anagnostopoulos, C.
(2016). Quality-optimized predictive analytics. Applied
Intelligence, 45(4), 1034–1046. Retrieved from
http://franklin.captechu.edu:2123/10.1007/s10489-016-0807-x
Anitha, P., & Patil, M. M. (2018). A review of data analytics for supply chain management: A case study. International Journal of Information Engineering and Electronic Business, 10(5), 30–39. Retrieved from http://franklin.captechu.edu:2123/10.5815/ijieeb.2018.05.05
Center for Development of Security Excellence (CDSE). (2017, May). Marking classified information: Job aid. Defense Security Service. Retrieved from https://www.cdse.edu/documents/cdse/Marking_Classified_Information.pdf
Center
for Innovation in Research and Teaching (CIRT). (n.d.). Quantitative
approaches. Grand Canyon University. Retrieved from https://cirt.gcu.edu/research/developmentresources/research_ready/quantresearch/
approaches
Chollet, F. (2018). Deep learning with Python. Shelter Island, NY: Manning publications.
Columbus, L. (2019, June 16). Top 10 cybersecurity companies to watch in 2019. Forbes. Retrieved from https://www.forbes.com/sites/louiscolumbus/2019/06/16/top-10-cybersecurity-companies-to-watch-in-2019/#4b683b696022
Cooper, H. (2018) Reporting quantitative research in psychology: How to meet APA style journal article reporting standards (2nd ed.). Washington, DC: American Psychological Association.
Creswell, J. W., & Creswell, J. D. (2018). Research design: Qualitative, quantitative, and mixed methods approaches (5th ed.). Thousand Oaks, CA: Sage.
Galloppo, G., & Previati, D. (2014). A review of methods for combining internal and external data. The Journal of Operational Risk, 9(4), 83–103. Retrieved from https://franklin.captechu.edu:2074/docview/1648312043?accountid=44888
Gupta, D., & Rani, R. (2018). A study of big data evolution and research challenges. Journal of Information Science, 1–19. Retrieved from https://doi.org/10.1177/0165551518789880
Halladay, S. D. (2013). Using predictive analytics to improve decisionmaking. The Journal of Equipment Lease Financing (Online), 31(2), 1–6. Retrieved from https://franklin.captechu.edu:2074/docview/1413251757?accountid=44888
Hassani, B. K., & Renaudin, A. (2018). The cascade bayesian approach: Prior transformation for a controlled integration of internal data, external data and scenarios. Risks, 6(2), 1–17. Retrieved from http://franklin.captechu.edu:2123/10.3390/risks6020047
Hensel, N. (2016). The defense industry: Tradeoffs between fiscal constraints and national security challenges. Business Economics, 51(2), 111–122. Retrieved from http://franklin.captechu.edu:2123/10.1057/be.2016.16
Hu, Z., Gnatyuk, V., Sydorenko, V., Odarchenko, R., & Gnatyuk, S. (2017). Method for cyberincidents network-centric monitoring in critical information infrastructure. International Journal of Computer Network and Information Security, 9(6), 30. Retrieved from http://franklin.captechu.edu:2123/10.5815/ijcnis.2017.06.04
Hubbard, D., & Seiersen, R. (2016). How to measure anything in cybersecurity risk. Hoboken, NJ: John Wiley & sons.
K, P. C., & Shivakumar, B. L. (2014). A review of trends and technologies in business analytics. International Journal of Advanced Research in Computer Science, 5(8), 225–229. Retrieved from https://franklin.captechu.edu:2074/docview/1658426584?accountid=44888
Koerner, B. (2016, October 23). Inside the cyberattack that shocked the US government. Wired. Retrieved from https://www.wired.com/2016/10/inside-cyberattack-shocked-us-government/
Kumar, P., & Verma, R. S. (2017). A review on recent advances & future trends of security in honeypot. International Journal of Advanced Research in Computer Science, 8(3). Retrieved from https://franklin.captechu.edu:2074/docview/1901458306?accountid=44888
Lee, A. J. (2015). Predictive analytics: The new tool to combat fraud, waste and abuse. The Journal of Government Financial Management, 64(2), 12–16. Retrieved from https://franklin.captechu.edu:2074/docview/1711620017?accountid=44888
Loy, J. (2019). Neural network projects with Python. Birmingham, UK: Packt.
Nagrecha, S., & Chawla, N. V. (2016). Quantifying decision making for data science: From data acquisition to modeling. EPJ Data Science, 5(1), 1–16. Retrieved from http://franklin.captechu.edu:2123/10.1140/epjds/s13688-016-0089-x
Naylor, B. (2016, June 6). One year after OPM data breach, what has the government learned? National Public Radio. Retrieved from https://www.npr.org/sections/alltechconsidered/2016/06/06/480968999/one-year-after-opm-data-breach-what-has-the-government-learned
Oltramari, A., & Kott, A. (2018). Towards a reconceptualisation of cyber risk: An empirical and ontological study. Journal of Information Warfare, 17(1), 4–73. Retrieved from https://franklin.captechu.edu:2074/docview/2059071274?accountid=44888
Paliwal, D., Vaya, D., Khandelwal, S. (2013). Mathematical analysis of problem statements: Artificial intelligence. International Journal of Advanced Research in Computer Science, 4(3). Retrieved from https://franklin.captechu.edu:2074/docview/1443744864?accountid=44888
Pham, T. M. (2018). Exploring strategies for incorporating population-level external information in multiple imputation of missing data (Doctoral dissertation). Retrieved from EBSCO Open Dissertations. http://search.ebscohost.com/login.aspx?direct=true&db=ddu&AN=788945D34A68B6CD&site=ehost-live
Rashid, T. (2016). Make your own neural network. Amazon Digital Services, LLC: Tariq Rashid.
Rodriguez, L., & Da Cunha, C. (2018). Impacts of big data analytics and absorptive capacity on sustainable supply chain innovation: A conceptual framework. LogForum, 14(2), 151–161. Retrieved from http://franklin.captechu.edu:2123/10.17270/J.LOG.267
Schroer, A. (2019, April 10). 25 Companies merging AI and cybersecurity to keep us safe and sound. Built-In. Retrieved from https://builtin.com/artificial-intelligence/artificial-intelligence-cybersecurity
Shaikh, F. (2016, October 3). Deep learning guide: Introduction to implementing neural networks using TensorFlow in Python. Analytics Vidhya. Retrieved from https://www.analyticsvidhya.com/blog/2016/10/an-introduction-to-implementing-neural-networks-using-tensorflow/
Starks, T. (2019, July 9). Cyber incidents were expensive in 2018. Politico. Retrieved from https://www.politico.com/newsletters/morning-cybersecurity/2019/07/09/cyber-incidents-were-expensive-in-2018-675243
Starr, B. (2015, July 31). Military still dealing with cyberattack ‘mess.’ CNN. Retrieved from https://www.cnn.com/2015/07/31/politics/defense-department-computer-intrusion-email-server/index.html
Taylor, M. (2017). Neural network math: A visual introduction for beginners. Vancouver, Canada: Blue Windmill Media.
Udemy. (n.d.). Machine learning: Build neural networks in 77 lines of code. Retrieved from https://www.udemy.com/machine-learning-build-a-neural-network-in-77-lines-of-code/learn/lecture/13179726#overview
Walsh, K. (n.d.). Audit log best practices for information security [Blog post]. Reciprocity. Retrieved from https://reciprocitylabs.com/audit-log-best-practices-for-information-security/
Warwick, K. (2010). Cultured neural networks. Proceedings of the Institution of Mechanical Engineers, Part I: Journal of Systems and Control Engineering, 224(2), 109–111. Retrieved from https://doi.org/10.1243/09596518JSCE916
Watkins, L. A., & Hurley, J. S. (2015). Cyber maturity as measured by scientific-based risk metrics. Journal of Information Warfare, 14(3), 57–65. Retrieved from https://franklin.captechu.edu:2074/docview/1967314091?accountid=44888
Weng, B. (2017). Application of machine learning techniques for stock market prediction (Doctoral dissertation). Retrieved from EBSCO Open Dissertations. http://search.ebscohost.com/login.aspx?direct=true&db=ddu&AN=DE0B8B4C2E217AE3&site=ehost-live
Wilner, A. S. (2018). Cybersecurity and its discontents: Artificial intelligence, the Internet of Things, and digital misinformation. International Journal, 73(2), 308–316. Retrieved from https://doi.org/10.1177/0020702018782496
Zuech, R., Khoshgoftaar, T. M., & Wald, R. (2015). Intrusion detection and big heterogeneous data: A survey. Journal of Big Data, 2(1), 1–41. Retrieved from http://franklin.captechu.edu:2123/10.1186/s40537-015-0013-4
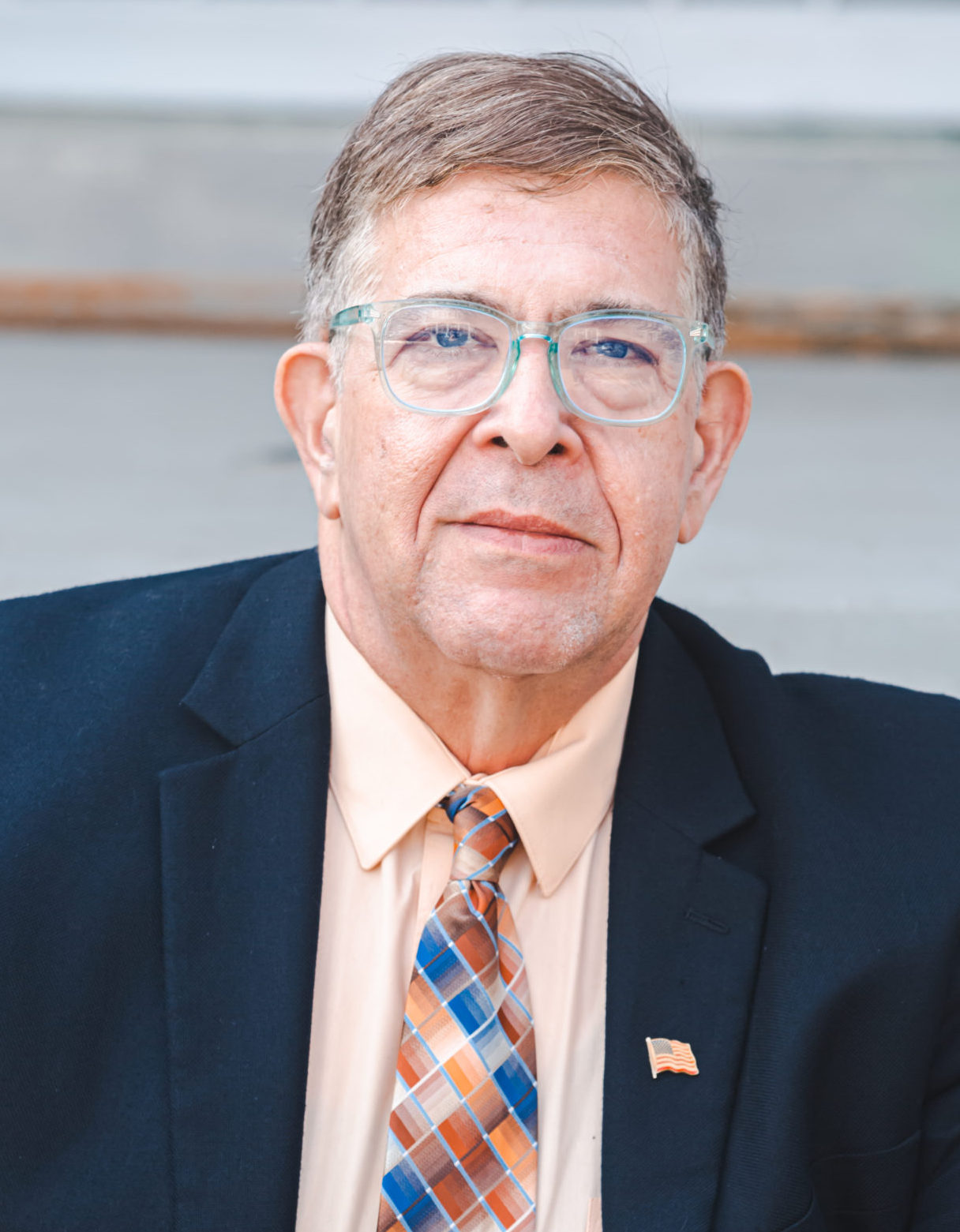
Dr. Russo is currently the Senior Data Scientist with Cybersenetinel AI in Washington, DC. He is a former Senior Information Security Engineer within the Department of Defense’s (DOD) F-35 Joint Strike Fighter program. He has an extensive background in cybersecurity and is an expert in the Risk Management Framework (RMF) and DOD Instruction 8510, which implement RMF throughout the DOD and the federal government. He holds a Certified Information Systems Security Professional (CISSP) certification and a CISSP in information security architecture (ISSAP). He has a 2017 Chief Information Security Officer (CISO) certification from the National Defense University, Washington, DC. Dr. Russo retired from the US Army Reserves in 2012 as a Senior Intelligence Officer.