Is there a need for a Cybersecurity-Data Science Conceptual Framework??

Discussion-Thought Piece on the Future of Data Science Solutions in the Cybersecurity Battle Frontlines
The world is run by ones and zeroes…There’s a war out there…It’s about who controls the information (Kaplan, 2016, p. 31).
Regular intrusions into the critical United States (U.S.) state and federal Information Technology (IT) systems highlight the ever agile and highly impactful effects of cyber-threats worldwide (Allyn, 2019; Olson, 2012; Starr, 2015; Stoll, 2005). The 2015 Office of Personnel Management (OPM) security breach was one of the most extensive and damaging exfiltrations of U.S. government personnel data in history (Koerner, 2016; Naylor, 2016). Cyber-attacks have even adversely affected the supposedly highly protected networks of the Department of Defense (DOD). “For nearly a week, some 4,000-key military and civilian personnel working for the Joint Chiefs of Staff [had] lost access to their unclassified email after what is now believed to be an intrusion into the critical Pentagon server that handles that email network” (Kaplan, 2016; Starr, 2015). The ability to better detect and prevent these cyber-assaults by nefarious threats has still not improved (Allyn, 2019; Garamone, 2018; Stoll, 2005).
Cyber-thugs are also regularly hacking companies and agencies around the globe (Kaplan, 2016; Olson, 2012). Computer attacks frequently happen to even the most technically savvy companies such as Eurofins, a United Kingdom’s (U.K.) based company in 2019 (Devlin, 2019; Olson, 2012). It paid an undisclosed amount of money to hackers to regain access to their databases’ and records’ repositories; this information was vital to Britain’s primary criminal forensics support firm to the multitude of U.K.’s law enforcement departments (Devlin, 2019). Technically capable agencies and companies still fail against repeated cyber-assaults, and their ability to detect and stop their effects remain limited (Allyn, 2019; Olson, 2012; Garamone, 2018; Stoll, 2005).
Ezeife, Dong, and Aggarwal (2008) describe the frustrations of intrusion detection efforts within corporate and agency networks. The requirements to monitor threats and update threat data sources, lists, and reports are labor-intensive activities (Ezeife, Dong, & Aggarwal, 2008). These demands focus on detecting, and not preventing, a cyber-threat intrusion into a company’s or agencies’ IT environment, i.e., network. Ezeife et al. (2008) further describe the need to maintain threat signature databases, used to identify threats, as requiring “a lot of human involvement” (Ezeife et al., 2008, p. 98). The need for more capable automated solutions is critical to any future success against these threats.
Specifically, the U.S. Defense Industrial Base (DIB) provides contract goods and services to the DOD and faces the challenges of cyber-threats regularly (Garamone, 2018; Hensel, 2016). DIB companies must balance national security protection requirements with their financial stability, and the knowledge that they too are valuable targets to the enemy (Hensel, 2016). These businesses face growing federal regulatory demands to protect their stored critical DOD-provided information and comply with DOD cybersecurity, and federally-driven requirements are often cumbersome and laborious (Ezeife et al., 2008; National Institute of Standards and Technology [NIST], 2015; 2018). They must secure their sensitive networks and data from cyber-threat nation-state actors such as China, Russia, Iran, and North Korea seeking insight to DOD operations and intentions against them in the realm of cyberspace (Garamone, 2018; Hensel, 2016; Starks, 2019).
Internal versus External Data
Companies rely heavily upon internal security, system, and antivirus logs, i.e., internal data, to identify risks and threats within their IT infrastructure (Ezeife et al., 2008; Zuech, Khoshgoftaar, & Wald, 2015). Explicitly, there is a need to include external or heterogeneous data to supplement threat detection and prevention and to protect the DOD and the nation’s data better (Hensel, 2016; Nagrecha & Chawla, 2016). External or heterogeneous data exists outside the resident IT environment; it is exterior to the local computer network and can further enhance an organization’s situational awareness and ability to respond to threats (Galloppo & Previati, 2014; Hassani & Renaudin, 2018; Nagrecha & Chawla, 2016).
Cybersecurity Weaknesses
The addition of external data offers more effective protection by providing valuable intelligence into the IT environment beyond the confines of an organization’s localized network (Rodriguez & Da Cunha, 2018). This information may include, for example, government indicators, honeypots, or honeynets in machine-readable formats that are designed to identify threat Tactics, Techniques, and Procedures (TTP) (Kumar & Verma, 2017; Ng, Pan, & Xiang, 2018; Spitzner, 2003; Zhan, Xu, M., & Xu, S., 2013). External data also includes associated attack statistics that can be analyzed and studied by current technical methods and means to determine the kind of threat and its associated attack (Kumar & Verma, 2017; Spitzner, 2003). In general, industry and governments have done an overall poor job in leveraging heterogeneous data sources and suffer from regular attacks by the myriad of cyber-threats (Starks, 2019; Starr, 2015; Zuech et al., 2015). Any solution to the cybersecurity challenge must be nimbler and automated to counter daily cyber-assaults from the global threat community (Zuech et al., 2015).
Additionally, organizations do not only have challenges at the data but the higher system-to-system level. “Compounding the [cybersecurity detection] problem further, existing IT security systems seldom integrate across a wide spectrum of an organizations’ information systems” (Zuech et al., 2015, p. 2). There are many avenues for cyber-threats to attack critical IT infrastructures (Allyn, 2019; Olson, 2012; Starks, 2019; Starr, 2015). This study focuses on the information or data level to confine its scope to just one portion of the cybersecurity challenge (Koerner, 2016; Naylor, 2016).
Data Science Acceptance
“Organizations are rapidly embracing data science to inform decision making,” and to protect its valuable virtual intellectual property and trade secrets (Nagrecha & Chawla, 2016, p. 1). “The shift from the conceptual to concrete use of machine learning is already underway” (Harvard Business Review [HBR], 2018, p. 1). Data science offers a powerful solution to addressing the problems of effective cybersecurity protection measures, and interest in this capability is continually expanding (see Figure 1).
Recently, the term cyber analytics emerged as the union between the challenges of cybersecurity and the leveraging of data science to solve the relentless trials of cybersecurity attacks (Djekic, 2019). There are many existing statistical and ever-growing data modeling and predictive capabilities arising from the field of data science (Loy, 2019; Silver, 2012). The growth of data science is directly attributable to the availability of Big Data sources and the associated accessibility of Artificial Intelligence (AI) and Machine Learning (ML) solutions (Fang, Xu, M., Xu, S., & Zhao, 2019; Wilner, 2018; Yu-Zhong, Zi-Gang, Xu, & Ying-Cheng, 2015). AI affords better decision-making and improves the overall effectiveness to observe and counter threats to an organization (Chimento, 2019; Fang et al., 2019; Gupta & Rani, 2018; Halladay, 2013; K & Shivakumar, 2014).
The Power of Predictive Analytics
Current capabilities of Artificial Intelligence (AI)/Machine Learning (ML) Predictive Analytics (PA), the availability of massive and insightful datasets, provide greater depth for a company or agency “to lower…costs…and improve [its] overall efficiencies (Nagrecha & Chawla, 2016, p. 1). Companies are accepting of data science methods and tools to “not only deliver value from their internal data but also to connect with external data sources to develop a more complete data profile [of the threat]” (Nagrecha & Chawla, 2016, p. 1). The merger of the problems of detecting and protecting organizational networks with the capabilities of data science offer the next evolution in the battle against threats in cyberspace.
Can Data Science truly help improve the fight in cyberspace?
-
State of Massachusetts’ Written Information Security Program (WISP) Template–with suggestions$49.99
-
__ “Security Authorization Development Package Model (SADP-M)” Template–NIST 800-171 Accreditation Packages (Bronze-Version)$299.99
-
Department of Defense (DOD) Controlled Defense Information (CDI) Marking, Handling and Storage Guide and Template$7.99
-
FREE: Bomb Threat Checklist$0.00
-
Make Your Own Neural Network$29.99
-
AUTHOR SIGNED: Writing an Effective Plan of Action & Milestones (POAM): Universal Version ~SECOND EDITION$17.99
-
AUTHOR SIGNED: NIST 800-171: System Security Plan (SSP) Template & Workbook (2019)$19.99
-
AUTHOR SIGNED: The Agile/Security Development Life Cycle (A/SDLC): Integrating Security Functionality into the SDLC ~SECOND EDITION (2019)$19.99
-
AUTHOR SIGNED: The Threat Hunt Process (THP) Roadmap: A Pathway for Advanced Cybersecurity Active Measures$29.99
Expansive References
Allyn, B. (2019, August 20). 22 Texas towns hit with ransomware attack in ‘new front’ of cyberassault. National Public Radio. Retrieved from https://www.npr.org/2019/08/20/752695554/23-texas-towns-hit-with-ransomware-attack-in-new-front-of-cyberassault
Alvarenga, A., & Tanev, G. (2017). A cybersecurity risk assessment framework that integrates value-sensitive design. Technology Innovation Management Review, 7(4), 32–43. Retrieved from https://franklin.captechu.edu:2074/docview/1963139581?accountid=44888
Anagnostopoulos, C. (2016).
Quality-optimized predictive analytics. Applied Intelligence, 45(4),
1034–1046. Retrieved from
http://franklin.captechu.edu:2123/10.1007/s10489-016-0807-x
Anitha, P., & Patil, M. M. (2018). A review of data analytics for supply chain management: A case study. International Journal of Information Engineering and Electronic Business, 10(5), 30–39. Retrieved from http://franklin.captechu.edu:2123/10.5815/ijieeb.2018.05.05
Chimento Jr, J. J. (2019). Toward an Understanding of Using High Entropic Digital Communication Techniques in Cybersecurity Decision Making (Doctoral dissertation). Retrieved from ProQuest Dissertations and Theses database. (UMI No. 13897847)
Chollet, F. (2018). Deep learning with Python. Shelter Island, NY: Manning publications.
Clarke, R. A., & Knake, R. K. (2014). Cyber war. New York, NY: Harper Collins.
Cooper, H. (2018). Reporting quantitative research in psychology: How to meet APA style journal article reporting standards (2nd ed.). Washington, DC: American Psychological Association.
Committee on National Security Systems (CNSS). (2015, April 6). CNSS glossary. CNSS. Retrieved from https://rmf.org/wp-content/uploads/2017/10/CNSSI-4009.pdf
Corrigan, J. (2019, September 4). Pentagon, NSA laying groundwork for AI-powered cyber defenses. Nextgov. Retrieved from https://www.nextgov.com/cybersecurity/2019/09/pentagon-nsa-laying-groundwork-ai-powered-cyber-defenses/159649/
Creswell, J. W., & Creswell, J. D. (2018). Research design: Qualitative, quantitative, and mixed methods approaches (5th ed.). Thousand Oaks, CA: Sage.
Department of Justice. (n.d.). Privacy act of 1974. DOJ. Retrieved from https://www.justice.gov/opcl/privacy-act-1974
Devlin, H. (2019, July 5). Hacked forensic firm pays ransom after malware attack. The Guardian. Retrieved from https://www.theguardian.com/science/2019/jul/05/eurofins-ransomware-attack-hacked-forensic-provider-pays-ransom
Digital.com. (n.d.). The deep web and dark web [Blog post]. Digital.com. Retrieved from https://digital.com/blog/deep-dark-web/
Djekic, M. (2019, July 5). Cyber security analytic purposes [Blog post]. Cyber Defense Magazine. Retrieved from https://www.cyberdefensemagazine.com/cyber-security-analytics-purposes/
Dutt, I., Paul, S., & Bandyopadyay, D. (2012). Security in all-optical network using artificial neural network. International Journal of Advanced Research in Computer Science, 3(2) Retrieved from https://franklin.captechu.edu:2074/docview/1443717924?accountid=44888
Elder, J. (2013, June). It is a mistake to…lack relevant data [White paper]. Charlottesville, VA: Elder Research.
European Union (EU). (n.d.). GDPR key changes. EU. Retrieved from https://eugdpr.org/the-regulation/
Ezeife, C. I., Dong, J., & Aggarwal, A. K. (2008). SensorWebIDS: A web mining intrusion detection system. International Journal of Web Information Systems, 4(1), 97–120. Retrieved from http://franklin.captechu.edu:2123/10.1108/17440080810865648
Fang, X., Xu, M., Xu, S., & Zhao, P. (2019). A deep learning framework for predicting cyber attacks rates. EURASIP Journal on Information Security, 2019(1), 1–11. Retrieved from http://franklin.captechu.edu:2123/10.1186/s13635-019-0090-6
Forcepoint. (n.d.). What is spoofing? Spoofing defined, explained, and explored [Blog post]. Forcepoint. https://www.forcepoint.com/cyber-edu/spoofing
Funke, D., & Benkleman, S. (2019, May 23). How Russia’s disinformation strategy is evolving. Poynter. Retrieved from https://www.poynter.org/fact-checking/2019/how-russias-disinformation-strategy-is-evolving/
Garamone, J. (2018, February 13). Cyber tops list of threats to U.S. director of national intelligence says. Defense.gov. Retrieved from https://www.defense.gov/Newsroom/News/Article/Article/1440838/cyber-tops-list-of-threats-to-us-director-of-national-intelligence-says/
Galloppo, G., & Previati, D. (2014). A review of methods for combining internal and external data. The Journal of Operational Risk, 9(4), 83–103. Retrieved from https://franklin.captechu.edu:2074/docview/1648312043?accountid=44888
Gupta, D. (2017, May 21). 25 must know terms & concepts for beginners in deep learning. Analytics Vidhya. Retrieved from https://www.analyticsvidhya.com/blog/2017/05/25-must-know-terms-concepts-for-beginners-in-deep-learning/
Gupta, D., & Rani,
R. (2018). A study of big data evolution and research challenges. Journal
of
Information Science, 1–19. Retrieved from https://doi.org/10.1177/0165551518789880
Halladay, S. D. (2013). Using predictive analytics to improve decisionmaking. The Journal of Equipment Lease Financing (Online), 31(2), 1–6. Retrieved from https://franklin.captechu.edu:2074/docview/1413251757?accountid=44888
Harvard Business Review (HBR). (2018). Artificial intelligence and machine learning driving tangible value for business [Briefing paper].
Hassani, B. K., & Renaudin, A. (2018). The cascade bayesian approach: Prior transformation for a controlled integration of internal data, external data and scenarios. Risks, 6(2), 1–17. Retrieved from http://franklin.captechu.edu:2123/10.3390/risks6020047
Hensel, N. (2016). The defense industry: Tradeoffs between fiscal constraints and national security challenges. Business Economics, 51(2), 111–122. Retrieved from http://franklin.captechu.edu:2123/10.1057/be.2016.16
Hu, Z., Gnatyuk, V., Sydorenko, V., Odarchenko, R., & Gnatyuk, S. (2017). Method for cyberincidents network-centric monitoring in critical information infrastructure. International Journal of Computer Network and Information Security, 9(6), 30. Retrieved from http://franklin.captechu.edu:2123/10.5815/ijcnis.2017.06.04
Hubbard, D., & Seiersen, R. (2016). How to measure anything in cybersecurity risk. Hoboken, NJ: John Wiley & Sons.
Jasim, Y. A. (2018). Improving intrusion detection systems using artificial neural networks. ADCAIJ: Advances in Distributed Computing and Artificial Intelligence Journal, 7(1), 49–65. Retrieved from http://franklin.captechu.edu:2123/10.14201/ADCAIJ2018714965
K, P. C., & Shivakumar, B. L. (2014). A review of trends and technologies in business analytics. International Journal of Advanced Research in Computer Science, 5(8), 225–229. Retrieved from https://franklin.captechu.edu:2074/docview/1658426584?accountid=44888
Kaplan, F. (2016). Dark territory: The secret history of cyber war. New York, NY: Simon & Schuster.
Kivikko, K., Mäkinen, A., Järventausta, P., Silvast, A., Heine, P., & Lehtonen, M. (2008). Comparison of reliability worth analysis methods: Data analysis and elimination methods. IET Proceedings. Generation, Transmission, and Distribution, 2(3), 321–329. Retrieved from https://franklin.captechu.edu:2074/docview/1627082453?accountid=44888
Koerner, B. (2016, October 23). Inside the cyberattack that shocked the US government. Wired. Retrieved from https://www.wired.com/2016/10/inside-cyberattack-shocked-us-government/
Lee, A. J. (2015). Predictive analytics: The new tool to combat fraud, waste and abuse. The Journal of Government Financial Management, 64(2), 12–16. Retrieved from https://franklin.captechu.edu:2074/docview/1711620017?accountid=44888
Loumiotis, I., Stamatiadi, T., Adamopoulou, E., Demestichas, K., & Sykas, E. (2013). Dynamic backhaul resource allocation in wireless networks using artificial neural networks. Electronics Letters, 49(8), 1–2. Retrieved from https://franklin.captechu.edu:2074/docview/1494555435?accountid=44888
Loy, J. (2019). Neural network projects with Python. Birmingham, UK: Packt.
Lyngaas, S. (2019, April 23). Someone is spoofing big bank IP addresses-possibly to embarrass security vendors. Cyberscoop. Retrieved from https://www.cyberscoop.com/spoofed-bank-ip-address-greynoise-andrew-morris-bank-of-america/
McGibony. (2015, June 30). Be a data detective [White paper]. Charlottesville, VA: Elder Research.
Nagrecha, S., & Chawla, N. V. (2016). Quantifying decision making for data science: From data acquisition to modeling. EPJ Data Science, 5(1), 1–16. Retrieved from doi:http://franklin.captechu.edu:2123/10.1140/epjds/s13688-016-0089-x
National Institute of Standards and Technology (NIST). (2015, January 22). Security and Privacy Controls for Federal Information Systems and Organizations. NIST. Retrieved from https://csrc.nist.gov/publications/detail/sp/800-53/rev-4/final
National Institute of Standards and Technology (NIST). (2018, June 7). Protecting Controlled Unclassified Information in Nonfederal Systems and Organizations. NIST. Retrieved from https://csrc.nist.gov/publications/detail/sp/800-171/rev-1/final
Naylor, B. (2016, June 6). One year after OPM data breach, what has the government learned? National Public Radio. Retrieved from https://www.npr.org/sections/alltechconsidered/2016/06/06/480968999/one-year-after-opm-data-breach-what-has-the-government-learned
Ng, C., Pan, L., Xiang, Y. (2018). Honeypot frameworks and their applications: A new framework. Singapore: Springer.
Novetta. (n.d.). Know your network: Arm your analysts [Blog post]. Novetta. Retrieved from https://www.novetta.com/products/novetta-cyber-analytics/
Olson, P. (2012). We are anonymous: Inside the hacker world of LulzSec, Anonymous, and the global cyber insurgency. New York, NY: Little, Brown, and Company.
Oltramari, A., & Kott, A. (2018). Towards a reconceptualisation of cyber risk: An empirical and ontological study. Journal of Information Warfare, 17(1), 4–73. Retrieved from https://franklin.captechu.edu:2074/docview/2059071274?accountid=44888
Orgera, S. (2019, August 5). How to use TOR browser for anonymous web browsing. Lifewire. Retrieved from https://www.lifewire.com/tor-browser-tutorial-4103599
Paliwal, D., Vaya, D., Khandelwal, S. (2013). Mathematical analysis of problem statements: Artificial intelligence. International Journal of Advanced Research in Computer Science, 4(3) Retrieved from https://franklin.captechu.edu:2074/docview/1443744864?accountid=44888
Palo Alto. (n.d.). What is an endpoint [Blog post]? Palo Alto. Retrieved from https://www.paloaltonetworks.com/cyberpedia/what-is-an-endpoint
Pham, T. M. (2018). Exploring strategies for incorporating population-level external information in multiple imputation of missing data (Doctoral dissertation). Retrieved from EBSCO Open Dissertations. http://search.ebscohost.com/login.aspx?direct=true&db=ddu&AN=788945D34A68B6CD&site=ehost-live
Rashid, T. (2016). Make your own neural network. Amazon Digital Services, LLC: Tariq Rashid.
Rodriguez, L., & Da Cunha, C. (2018). Impacts of big data analytics and absorptive capacity on sustainable supply chain innovation: A conceptual framework. LogForum, 14(2), 151–161. Retrieved from doi:http://franklin.captechu.edu:2123/10.17270/J.LOG.267
RSA. (2016, February 5). The role of TOR in cybercrime [Blog post]. RSA. Retrieved from https://www.rsa.com/en-us/blog/2016-02/role-tor-cybercrime
Schroer, A. (2019, April 10). 25 Companies merging AI and cybersecurity to keep us safe and sound. Built-In. Retrieved from https://builtin.com/artificial-intelligence/artificial-intelligence-cybersecurity
Shaikh, F. (2016, October 3). Deep learning guide: Introduction to implementing neural networks using TensorFlow in Python. Analytics Vidhya. Retrieved from https://www.analyticsvidhya.com/blog/2016/10/an-introduction-to-implementing-neural-networks-using-tensorflow/
Siegel, E. (2016). Predictive analytics: The power to predict who will click, buy, lie, or die. Hoboken, NJ: John Wiley & Sons.
Silver, N. (2012). The signal and the noise: Why so many predictions fail–but some don’t. New York, NY: Penguin.
Singh, A. V., Juyal, V., & Saggar, R. (2017). Trust based intelligent routing algorithm for delay tolerant network using artificial neural network. Wireless Networks, 23(3), 693–702. Retrieved from http://franklin.captechu.edu:2123/10.1007/s11276-015-1166-y
Smith, A. (2019, July 4). 7 fundamental steps to complete a data project [Blog post]. Dataiku. Retrieved from https://blog.dataiku.com/2019/07/04/fundamental-steps-data-project-success
Spitzner, L. (2003). Honeypots: tracking hackers. Boston, MA: Addison-Wesley.
Starks, T. (2019, July 9). Cyber incidents were expensive in 2018. Politico. Retrieved from https://www.politico.com/newsletters/morning-cybersecurity/2019/07/09/cyber-incidents-were-expensive-in-2018-675243
Starr, B. (2015, July 31). Military still dealing with cyberattack ‘mess.’ CNN. Retrieved from https://www.cnn.com/2015/07/31/politics/defense-department-computer-intrusion-email-server/index.html
Strand, M., Wangler, B., & Niklasson, M. (2004). External data incorporation into data warehouses: an exploratory study of identification and usage practices in banking organizations. In Proceedings of the CAiSE Forum at the 16th International Conference on Advanced Information Systems Engineering (CAiSE’04) (pp. 103–112).
Sukamolson, S. (2007). Fundamentals of quantitative research. Language Institute Chulalongkorn University, 1, 2-3.
Johns, A. (n.d.). What is internal data? – Definition & sources [Blog post]. Study.com. Retrieved from https://study.com/academy/lesson/what-is-internal-data-definition-sources.html
Taylor, M. (2017). Neural network math: A visual introduction for beginners. Vancouver, Canada: Blue Windmill Media.
Tetlock, P. E., & Gardner, D. (2015). Superforecasting: The art and science of prediction. New York, NY: Crown Publishers.
Thurber, M. (2018, April 6). What is data wrangling and why does it take so long [Blog post]. Elder Research. Retrieved from https://www.elderresearch.com/blog/what-is-data-wrangling
Trullen, J., & Bartunek, J. M. (2007). What a design approach offers to organization development. The Journal of Applied Behavioral Science, 43(1), 23–40. Retrieved from https://franklin.captechu.edu:2074/docview/236352484?accountid=44888
U.S. Health and Human Services (HHS). (n.d.). Health information privacy. HHS. Retrieved from https://www.hhs.gov/hipaa/index.html
Udemy. (n.d.). Machine learning: Build neural networks in 77 lines of code. Retrieved from https://www.udemy.com/machine-learning-build-a-neural-network-in-77-lines-of-code/learn/lecture/13179726#overview
Walsh, K. (n.d.). Audit log best practices for information security [Blog post]. Reciprocity. Retrieved from https://reciprocitylabs.com/audit-log-best-practices-for-information-security/
Walters, T. (n.d.). Incorporating external data into the data warehouse. SAS. Retrieved from https://support.sas.com/resources/papers/proceedings/proceedings/sugi22/DATAWARE/PAPER116.PDF
Warwick, K. (2010). Cultured neural networks. Proceedings of the Institution of Mechanical Engineers, Part I: Journal of Systems and Control Engineering, 224(2), 109–111. Retrieved from https://doi.org/10.1243/09596518JSCE916
Watkins, L. A., & Hurley, J. S. (2015). Cyber maturity as measured by scientific-based risk metrics. Journal of Information Warfare, 14(3), 57–65. Retrieved from https://franklin.captechu.edu:2074/docview/1967314091?accountid=44888
Weng, B. (2017). Application of machine learning techniques for stock market prediction (Doctoral dissertation). Retrieved from EBSCO Open Dissertations. http://search.ebscohost.com/login.aspx?direct=true&db=ddu&AN=DE0B8B4C2E217AE3&site=ehost-live
Wilner, A. S. (2018). Cybersecurity and its discontents: Artificial intelligence, the Internet of Things, and digital misinformation. International Journal, 73(2), 308–316. Retrieved from https://doi.org/10.1177/0020702018782496
Young, W. Y., Houston, J. S., Harris, J. H., Hoffman, R. G., & Wise, L. L. (1990). Large-scale predictor validation in project A: Data collection procedures and data base preparation. Personnel Psychology, 43(2), 301–311. Retrieved from https://franklin.captechu.edu:2074/docview/220131995?accountid=44888
Yu-Zhong, C., Zi-Gang Huang, Xu, S., & Ying-Cheng, L. (2015). Spatiotemporal patterns and predictability of cyberattacks. PLoS One, 10(5). Retrieved from https://franklin.captechu.edu:2074/docview/1685181563?accountid=44888
Zhan, Z., Xu, M., & Xu, S. (2013). Characterizing honeypot-captured cyber attacks: Statistical framework and case study. IEEE Transactions on Information Forensics and Security, 8(11), pp.1775–1789.
Zuech, R., Khoshgoftaar, T. M., & Wald, R. (2015). Intrusion detection and big heterogeneous data: A survey. Journal of Big Data, 2(1), 1–41. Retrieved from http://franklin.captechu.edu:2123/10.1186/s40537-015-0013-4
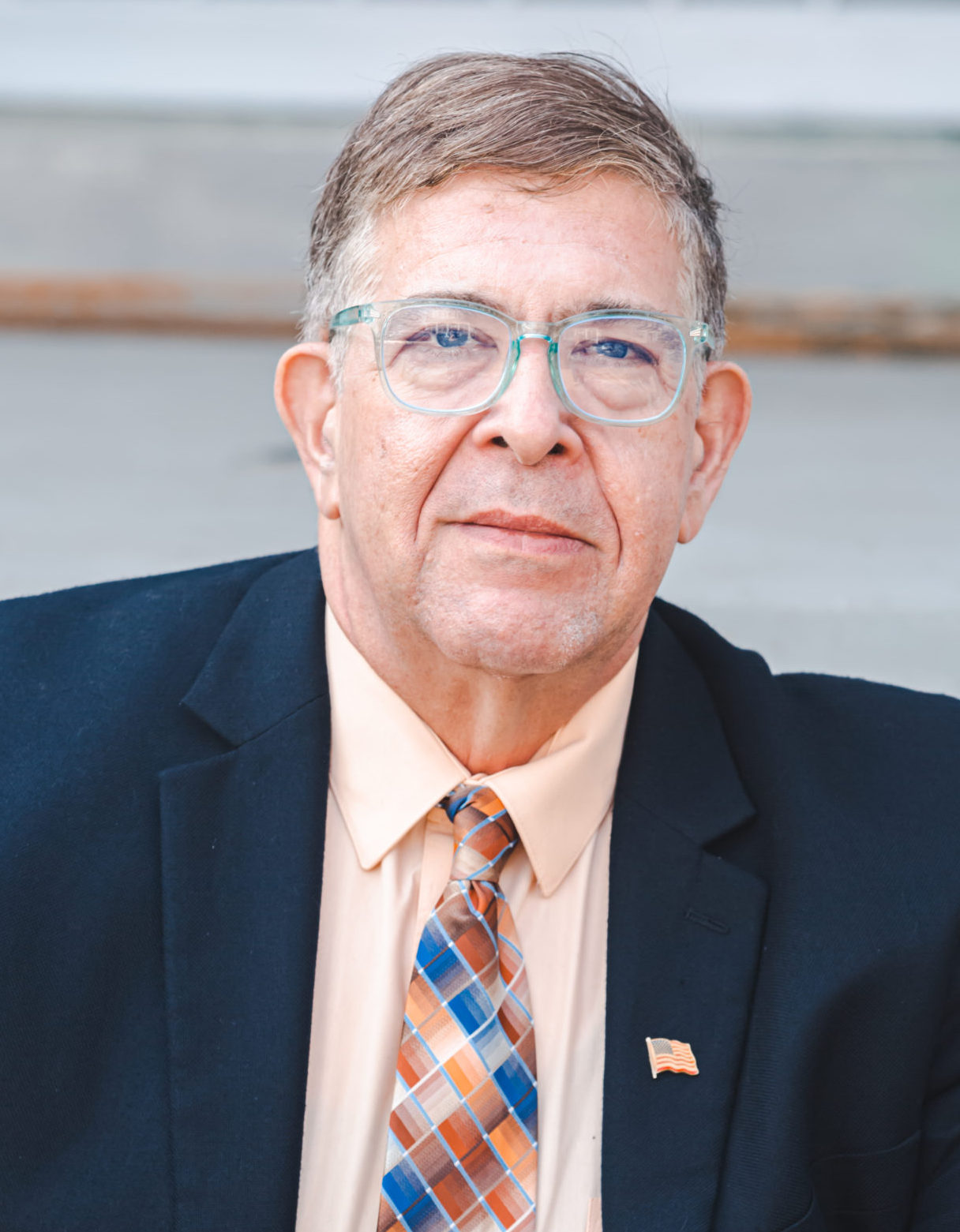
Dr. Russo is currently the Senior Data Scientist with Cybersenetinel AI in Washington, DC. He is a former Senior Information Security Engineer within the Department of Defense’s (DOD) F-35 Joint Strike Fighter program. He has an extensive background in cybersecurity and is an expert in the Risk Management Framework (RMF) and DOD Instruction 8510, which implement RMF throughout the DOD and the federal government. He holds a Certified Information Systems Security Professional (CISSP) certification and a CISSP in information security architecture (ISSAP). He has a 2017 Chief Information Security Officer (CISO) certification from the National Defense University, Washington, DC. Dr. Russo retired from the US Army Reserves in 2012 as a Senior Intelligence Officer.