CO-ACT: An Ethics Model for Data Science Privacy

Balancing the Benefits of Data Science with Privacy
The implications of data science have yet to solidify within the academic communities’ position with the needs of its societal benefits and concerns for individual privacy (Beaton, 2016; McQuillan, 2018). There is no surprise that the use and exploitation of data have become more accepted to understand better the massive amounts of current-day troves of collected data. The ability to process this information to identify critical scientific and social insights has become more essential to agency and corporate leadership (Nagrecha & Chawla, 2016). Data science offers a powerful means to address society’s problems; however, it also poses an equal concern for the individual’s privacy rights in the 21st Century. Data science can also disrupt the “popular notions of autonomy, consent, self-determination, privacy, and selfhood,” as described by scholars studying the effects of data science (Beaton, 2016, p. 353).
Furthermore, a critical subset of data science is the concept and presence of Big Data. Big Data is “large datasets which require non-traditional scalable solutions for data acquisition, storage, management, analysis, and visualization, aiming to extract actionable insights having the potential to impact every aspect of human life” (Gupta & Rani, 2018, p. 4). Big Data affords the capabilities as well as the concerns for individual privacy (Ndukwe, Daniel, & Butson, 2018). Big Data provides the “opportunities [for] discovering knowledge increase with the [associated] risks of privacy violation” (Monreale, Rinzivillo, Pratesi, Giannotti, & Pedreschi, 2014, p. 1). Without the current capabilities of Big Data storage and the ability to use data science tools and mechanisms, the likelihood of the debate regarding data privacy and protection would be of relatively minimal concern to academia and the general public.
As Kayser, Nehrke, and Zubovic (2018) discuss, data science and especially Big Data are likely to create “potentially enormous business value” for the global community (Kayser, Nehrke, & Zubovic, 2018, p. 16). In particular, the utilitarian measure of the “greater good” for society impacts this discussion (Collins, 2017, p. 132). How can the worldwide community reduce the effects on people who could be harmed by the release, manipulation, or falsification of their data?
Ethical Dilemma
The dilemma posed by data science is about how to best balance the needs of society with those of the individual. It becomes a delicate act, as described by Spock in The Wrath of Khan. As Spock engages in an ethical and utilitarian predicament, he understands he has the most significant ability of all members aboard the starship to survive prolonged radiation exposure. Spock decides to save the ship with the likelihood of his death. He expresses that the “needs of the many outweigh the needs of the few” (Sallin (Producer) & Meyer (Director), 1982). His ethical position is rooted in classic Western ethical considerations to create the highest good for his society, i.e., the U.S.S. Enterprise (Paik, Lee, & Pak, 2019). The problem is also a consideration of whether the Western concept of utilitarianism is enough in the age of data science.
Additionally, is the demand for the application of Responsible Data Science achievable (Van der Aalst, Wil, Bichler, & Heinzl, 2017, p. 311)? Van der Aalst, Wil, Bichler, and Heinzl (2017) are champions of the need to address privacy within a defined thought model. Van der Aalst et al. (2017) recognize the immense availability of data and its potential abuse and suggest that the problem needs to be addressed through the lifecycle of data science-based research and analysis. They offer a construct that the author partially employs in developing a final ethical model to ensure accountable data science.
There is concern by the author about the academic community and its dismissiveness of the demands for privacy in data collection, handling, and dissemination (McQuillan, 2018). McQuillan (2018) points out that the “traditional notion of data protection is of little relevance when it comes to data science” (p. 259). Additionally, the tone offered by Beaton’s (2016) historical review of Lionel Trilling’s 20th Century work on data criticism attempts to divert discussion of “rehearsing debates over privacy and personal data property” as overly unique and insignificant to some within the academic community (Beaton, 2016, p. 359). Scholars appear to be straddling the privacy issue in favor of the extreme greater good argument to the society where individual rights are of little or no interest (Beaton, 2016; McQuillan, 2018).
Background
This article suggests one framework based on other academic efforts, as described by the work of van der Aalst et al. (2017). This Dutch team considers the privacy issue under Western philosophical views and attitudes about individuals’ data protection rights. Further, the author attempts to hybridize the ideas emerging from the Responsible Data Science community and offers one candidate model to formulate an ethical position (van der Aalst et al., 2017). The framework endeavors to be based more on rationality and logic versus emotionality, which is likely to introduce unintended biases in evaluating privacy, and whether an action is correct.
While it is presumed that the advent of data science and Artificial Intelligence (AI) is a relatively new phenomenon, some do not realize modern AI had its roots in the early 1950s (Schuchmann, 2019). AI has experienced several historical highs and lows described as Artificial Intelligence Winters and Summers, respectively. The beginnings of modern-day AI emerged during Alan Turing’s renowned test in 1950 to establish the criteria to demonstrate whether a machine can show human-like intelligent behavior (Nield, 2019; Schuchmann, 2019). The history of AI and data science has been volatile but has also resulted in evolutionary capabilities to support decision-making and predictive insights (Nagrecha & Chawla, 2016).
Also, data science does not just collect and process volumes of Big Data but “transforms” it into knowledge to create added wisdom (McQuillan, 2018, p. 254). The Data-Information-Knowledge-Wisdom (DIKW) “hierarchy…[is] where each layer adds certain attributes over and above the previous one” to advance human understanding in a complex world (Gu & Zhang, 2014, p. 283). This transformation is intended to improve people’s lives and society as well.
Data may also be transformed by unfriendly nation-state actors, for example, to create false data analytic products to conduct or enhance a propaganda campaign. For example, data manipulation may hurt leadership’s ability to make the right decisions using data science measurability and predictiveness capabilities (Hurley, 2016). These data alterations are highly specialized and likely could negatively affect a person or company to create a false appearance of facts.
Finally, there is an emerging recognition, especially by the social science community, that the potential of human biases may be introduced in the data collection and analysis portion of any academic study or research (McQuillan, 2018). McQuillan (2018) describes it as a “less obvious problem…[with]…the potential production of new forms of unrecognized prejudice” (p. 257). The argument is rational; data science algorithms and data introduced by humans may be consciously or even subconsciously created with embedded biases. The proposed framework attempts to reduce this issue but can never be entirely successful, for example, when individuals or countries pursue nefarious political vice science-based outcomes (Hurley, 2016).
The privacy question. For example, anonymizing or de-identifying an individual’s data cannot be accomplished effectively and thoroughly to ensure privacy (Monreale et al., 2014). Removing or anonymizing key data fields such as name, date of birth, or social security number will not solve the problem of obfuscating a person’s identity based on such approaches alone.
Another challenge of data privacy in a highly connected world still allows for technologies, including data science, to discern individuals based on other data sources and methodologies. An example includes behavioral techniques to identify individuals using keystroke analysis and pattern recognition (Leberknight & Recce, 2015). With such a vast present-day availability of data and models, ensuring privacy continues to be a demanding goal.
Ethical thought. The ethical considerations of Western utilitarianism are based on the individual and the maximization of benefit for the most substantial parts of a society or population (Collins, 2017). Sheskin, Chevallier, Adachi, Berniūnas, Castelain, Hulín, … Baumard (2018) discuss that many cultures adhere to a collectivist approach in their ethical decision-making process, vice the Western philosophy of individualism and classic democratic principles (p. 220). This article’s framework will focus on individual rights, vice those espoused by Sheskin et al. (2018), that may create potential harm to a person.
A Data Science Ethical Decision Framework
Monreale et al. (2014) articulate a need for a framework to address the tradeoffs between the benefits of data science and privacy. This article leverages the work of van der Aalst et al. (2017) and their “Fairness, Accuracy[A], Confidentiality[C], and Transparency[T]” (FACT) model with one deletion and two explicit additions (p. 311). This framework is designed to provide a collection of considerations and approaches to increase the value of its outcomes.
The framework suggested adds critical thinking (C) and open-mindedness (O) at the periphery to create a complete and sensible model. Critical thinking is best described by logic devoid of emotions with a preference for measures or metrics. It requires that data be handled appropriately to avoid unintended privacy violations or prejudice of outcomes. Secondly, open-mindedness is added to allow for the free exchange of ideas among the parties involved in handling and processing data or information; this should include quality control and oversight to enforce institutional standards of morals, ethics, and laws. The author has designated this structure as the CO-ACT model (based upon the first letter of the five identified components) to create an ethical position on the topic of this article, see Figure 1.
Figure 1
The CO-ACT Ethical Position Model

Note. Adapted from source: van der Aalst, Wil M, P., Bichler, M., & Heinzl, A. (2017). Responsible data science. Business & Information Systems Engineering, 59(5), 311–313. doi:http://franklin.captechu.edu:2123/10.1007/s12599-017-0487-z
Furthermore, the deletion of fairness is necessary and contradicts many mainstream academics that, too describe it in terms of personal “judgment” and emotionality (Schweitzer & Gibson, 2008, p. 287). The concept of fairness is not a reasoned term. What may be considered reasonable to one segment of society may not be adequate for another. A resolution of the term fairness is a matter of individual desire and perspective and not objective reality, such as market forces or choosing another profession that will meet the desired aspiration.
The proposed CO-ACT ethical concept uses van der Aalst et al. (2017) work, addressing the first three standards of accuracy, confidentiality, and transparency. These three fundamental elements should be respected first in any experiment, initiative, or endeavor using data of United States (U.S.) persons as defined under federal law—this will also include corporate entities and their intellectual property and trade secrets. Accuracy should ensure that the information is complete and not manipulated (Hurley, 2016). The element of confidentiality needs to be the objective for everyone within the data processing effort to employ proper encryption and controlled release to only those team members with a valid need to know. Finally, transparency allows only authorized personnel to reconstruct the original data analysis to test and reproduce the same results to ensure data validity. In the era of data science, these components need to be at the forefront of determining the proper use of private information and provide the converse minimization of data exposure to others, not authorizing the use of personally identifiable data.
Personnel involved with the data need to consider all aspects of the negligent or intentional release of information. This is where critical-thinking considerations need to be part of any issue or action using personal information. Reviewing data analysis requires constant questioning of the data during the study and ensures research efforts are free from bias that may skew the results.
Finally, outcomes may not be as expected by the hypotheses or assumed direction. However, once factors of biases are eliminated, the results need to be supported based on the scientific outcomes of the data employed. The final analytic product should capture any caveats or confidence concerns to offer dissenting opinions. Ultimately, the CO-ACT model provides a holistic mental tool to avoid unintended logic pitfalls, reduce uncertainty, and offer increased individual-based privacy protections.
Framework’s Pros. The CO-ACT structure offers a means for scholars, scientists, and academics to apply reason to balancing societal and individual privacy needs and requirements. The model allows for defining a position based on several critical factors designed to avoid implicit or explicit prejudices. The standard provides a start-point with likely future changes based upon situations and experiences; it will only be as good as the rigors applied to the five elements of the CO-ACT framework.
Framework’s Cons. Any problem, including data science and privacy, needs to be measurable (Prusak, 2010). CO-ACT is a qualitative model and lacks measurability to support the author’s position that CO-ACT needs to be fully defined; it needs to be a quantitative approach. There are issues with moving to a quantitative model, as Hayden (2010) suggested. He states that “established metrics programs… struggle with understanding their … efforts” (p. 5). Companies create data science-based programs, but they have been more data collection efforts with little effort to identify the real measured problems or risks of applying a defined solution (Hayden, 2010). Measurability affords the effective use of data and its protection to create an ethical approach to handling and safeguarding information.
Values, Laws, Rules, Policies, and Procedures Application
The long-standing commitment to the Privacy Act of 1974 (Department of Justice [DOJ], 2020), and the Health Insurance Portability and Accountability Act (HIPAA), respectively, by U.S. federal and state governments, suggest that privacy is not a minor topic of interest under federal law. As with any new technologies, considerations of data defense and security in the data science age pose new concerns. As Joo, Kim, and Kim (2017) highlight, there is still a “good deal of legal uncertainty [that] exists regarding privacy regulations [as applied to data science and Big Data]” (Joo et al., 2017, p. 400). The law is consistently lagging in many areas, including privacy protections.
The values, rules, and laws as applied to data science violated may not be fully captured in prior laws and regulations; however, privacy is an ongoing opportunity for success and abuse. An example of recent abuse within the current U.S. rules governing confidentiality includes the United States (U.S.) government’s Analysis, Dissemination, Visualization, Insight, and Semantic Enhancement (ADVISE) program. This program allowed the unauthorized collection of U.S. individuals’ personally identifiable information and data. The program was later terminated in 2007 by Congress (D’Andre, 2007; Gu & Zhang, 2014).
Subsequently, as a result of Edward Snowden’s release of classified information in 2009 from the National Security Agency (NSA), it was discovered “another similar project [to ADVISE existed]” (Gu & Zhang, 2014, p. 287). The identified NSA PRISM [code name] program collected “even more wide and deep information [about U.S. citizens]” (p. 287). The PRISM program is another example where the federal government violated individual rights even with the many laws focused on personal privacy (Gu & Zhang, 2014). Also, this violated privacy rights under the Fourth Amendment of the Bill of Rights (U.S. Const. amend. IV) and demonstrates this issue goes beyond corporate or academic studies collecting and exploiting privacy-based data.
Conclusion
Any ethical position should be grounded based on an individualized and defined model. The challenges posed by the expansive growth of the field of data science offer great promise and potential dangers. Monreale et al. (2014) strongly favor that privacy is incorporated not just in ideas but within the information technology solutions “from the very start” (p. 3). Ethical decision-making needs to continually address privacy throughout the life of a data science research effort where personal data is employed.
As Hurley (2016) interestingly highlights, there are other dangers concerning using or misusing personal data. He suggests that data is susceptible to manipulation not just of the backend analysis but the data collection and processing by nefarious persons or countries. The exploitation of data to seek or distort for the desired outcome poses a grave challenge to ongoing ethical considerations of privacy and data security (Gu & Zhang, 2014; Hurley, 2016). The transparency element of the CO-ACT model offers a vital factor in remaining consistent and scientifically accurate in preventing these kinds of abuse.
Finally, the primacy of the individual needs to be reinforced in applying an ethical stance by everyone, including academics. The apparent quibbling about privacy affords no benefit in Western philosophic ethics (Beaton, 2016; Sheskin et al., 2018). “Issues of privacy and ethical concerns have often prevented researchers from accessing data for research purposes” (Ndukwe et al., 2018, p. 15). The author expects that the entirety of the academic community would be leading the charge for greater privacy protections, and, unfortunately, that is not wholly true. The author supports the Dutch initiative of Responsible Data Science, where a framework is essential to promoting societal and technological advances with a specific emphasis on individual privacy and security (van der Aalst, 2017).
References
Beaton, B. (2016). How to respond to data science: Early data criticism by lionel trilling. Information & Culture, 51(3), 352–372. Retrieved from doi:http://franklin.captechu.edu:2123/10.7560/IC51303
Collins, D. (2017). Business ethics: best practices for designing and managing ethical organizations. Thousand Oaks, CA: Sage Publications.
D’Andre, H. (2007, September 10). DHS scraps ADVISE data-mining software. Electronic Frontier Foundation. Retrieved from https://www.eff.org/deeplinks/2007/09/dhs-scraps-advise-data-mining-software
Gu, J., & Zhang, L. (2014). Some comments on big data and data science. Annals of Data Science, 1(3-4), 283–291. doi:http://franklin.captechu.edu:2123/10.1007/s40745-014-0021-9
Gupta, D., & Rani, R. (2018). A study of big data evolution and research challenges. Journal of
Information Science, 1–19. Retrieved from https://doi.org/10.1177/0165551518789880
Hayden, L. (2010). IT security metrics: A practical framework for measuring security & protecting data. New York, NY: McGraw Hill.
Hurley, J. S. (2018). Enabling successful artificial intelligence implementation in the department of defense. Journal of Information Warfare, 17(2), 65–82. Retrieved from https://franklin.captechu.edu:2074/docview/2137387163/363CACE28F7D48CBPQ/1?accountid=44888
Joo, S., Kim, S., & Kim, Y. (2017). An exploratory study of health scientists’ data reuse behaviors. Aslib Journal of Information Management, 69(4), 389–407. doi:http://franklin.captechu.edu:2123/10.1108/AJIM-12-2016-0201
Kayser, V., Nehrke, B., & Zubovic, D. (2018). Data science as an innovation challenge: From big data to value proposition. Technology Innovation Management Review, 8(3), 16–25. Retrieved from https://franklin.captechu.edu:2074/docview/2036405696?accountid=44888
Leberknight, C. S., & Recce, M. L. (2015). The application of keystroke analysis for physical security: A field experiment. Journal of Information Privacy & Security, 11(4), 211–227. Retrieved from doi:http://franklin.captechu.edu:2123/10.1080/15536548.2015.1105599
McQuillan, D. (2018). Data science as machinic neoplatonism. Philosophy & Technology, 31(2), 253–272. Retrieved from doi:http://franklin.captechu.edu:2123/10.1007/s13347-017-0273-3
Monreale, A., Rinzivillo, S., Pratesi, F., Giannotti, F., & Pedreschi, D. (2014). Privacy-by-design in big data analytics and social mining. EPJ Data Science, 3(1), 1–26. Retrieved from doi:http://franklin.captechu.edu:2123/10.1140/epjds/s13688-014-0010-4
Nagrecha, S., & Chawla, N. V. (2016). Quantifying decision making for data science: From data acquisition to modeling. EPJ Data Science, 5(1), 1–16. Retrieved from doi:http://franklin.captechu.edu:2123/10.1140/epjds/s13688-016-0089-x
Ndukwe, I. G., Daniel, B. K., & Butson, R. J. (2018). Data science approach for simulating educational data: Towards the development of teaching outcome model (TOM). Big Data and Cognitive Computing, 2(3). Retrieved from doi:http://franklin.captechu.edu:2123/10.3390/bdcc2030024
Nield, T. (2019, February 7). Is another AI winter coming? Hackernoon. Retrieved from https://hackernoon.com/is-another-ai-winter-coming-ac552669e58c
Paik, Y., Lee, J. M., & Pak, Y. S. (2019). Convergence in international business ethics? A comparative study of ethical philosophies, thinking style, and ethical decision-making between US and korean managers. Journal of Business Ethics, 156(3), 839–855. Retrieved from doi: http://franklin.captechu.edu:2123/10.1007/s10551-017-3629-9
Prusak, L. (2010, October 7). What can’t be measured. Harvard Business Review. Retrieved from https://hbr.org/2010/10/what-cant-be-measured
Sallin, R. (Producer) & Meyer, N. (Director). (1982). Star trek II: The wrath of khan [Motion picture]. United States: Paramount Pictures.
Schuchmann, S. (2019, May 12). History of the first AI winter. Toward Data Science. Retrieved from https://towardsdatascience.com/history-of-the-first-ai-winter-6f8c2186f80b
Schweitzer, M. E., & Gibson, D. E. (2008). Fairness, feelings, and ethical decision- making: Consequences of violating community standards of fairness. Journal of Business Ethics, 77(3), 287–301. Retrieved from doi:http://franklin.captechu.edu:2123/10.1007/s10551-007-9350-3
Sheskin, M., Chevallier, C., Adachi, K., Berniūnas, R., Castelain, T., Hulín, M., … Baumard, N. (2018). The needs of the many do not outweigh the needs of the few: the limits of individual sacrifice across diverse cultures. Journal of cognition and culture, 18(1–2), 205–223. Retrieved from https://www.researchgate.net/publication/324803905_The_Needs_of_the_Many_Do_Not_Outweigh_the_Needs_of_the_Few_The_Limits_of_Individual_Sacrifice_across_Diverse_Cultures/link/5aebaf99a6fdcc8508b6defd/download
U.S. Const. amend. IV.
U.S. Department of Justice. (2020, January 15). Privacy act of 1974. Retrieved from https://www.justice.gov/opcl/privacy-act-1974
van der Aalst, Wil M, P., Bichler, M., & Heinzl, A. (2017). Responsible data science. Business & Information Systems Engineering, 59(5), 311–313. Retrieved from doi:http://franklin.captechu.edu:2123/10.1007/s12599-017-0487-z
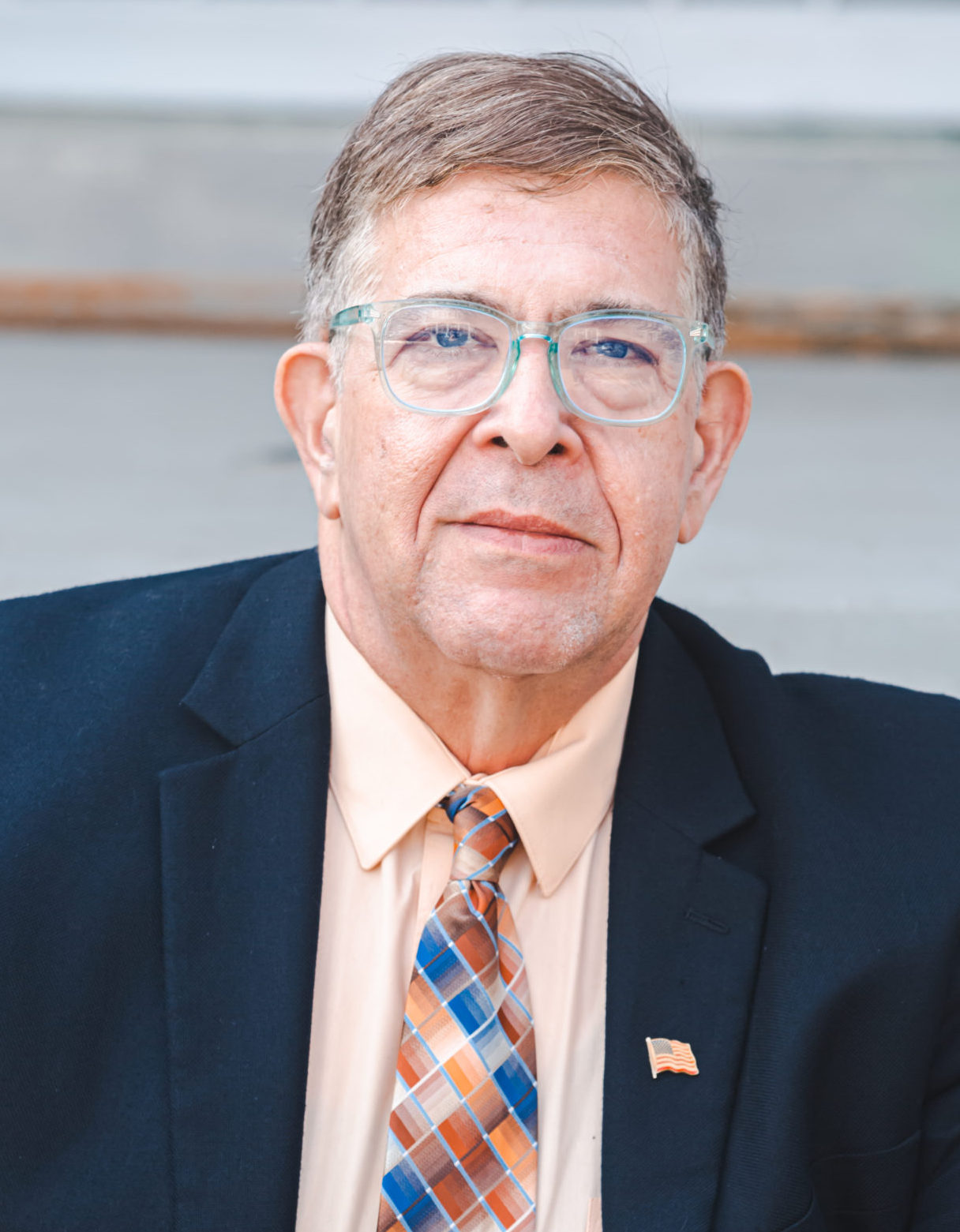
Dr. Russo is currently the Senior Data Scientist with Cybersenetinel AI in Washington, DC. He is a former Senior Information Security Engineer within the Department of Defense’s (DOD) F-35 Joint Strike Fighter program. He has an extensive background in cybersecurity and is an expert in the Risk Management Framework (RMF) and DOD Instruction 8510, which implement RMF throughout the DOD and the federal government. He holds a Certified Information Systems Security Professional (CISSP) certification and a CISSP in information security architecture (ISSAP). He has a 2017 Chief Information Security Officer (CISO) certification from the National Defense University, Washington, DC. Dr. Russo retired from the US Army Reserves in 2012 as a Senior Intelligence Officer.