Honeypot Data to Improve Threat Detection
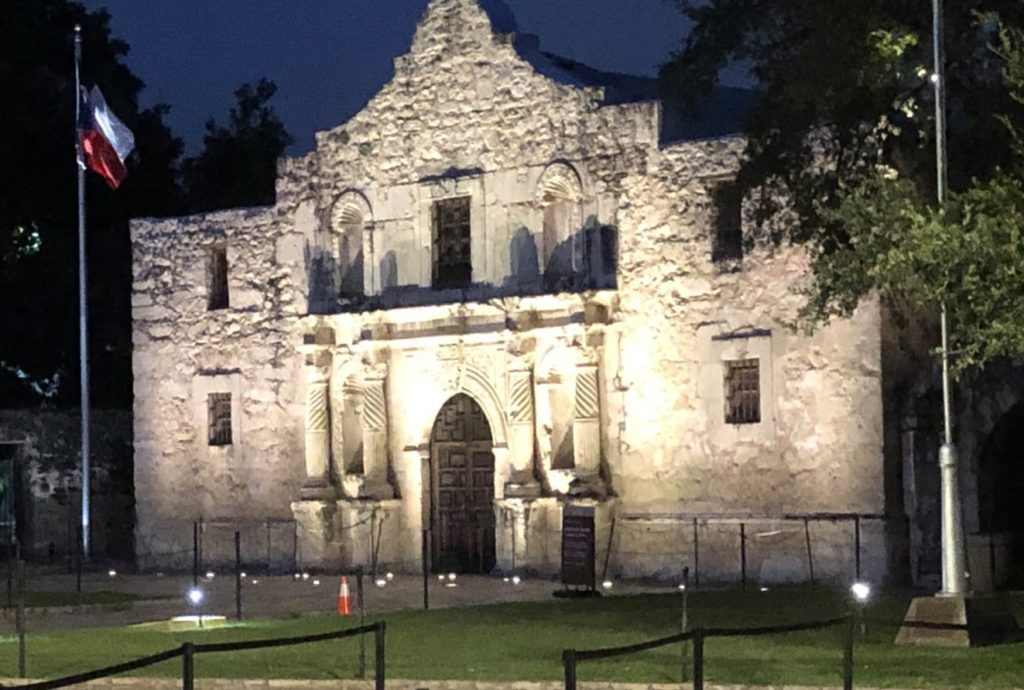
What are we still missing ?
Background
Ezeife, Dong, and Aggarwal (2008) describe the frustrations of intrusion detection of cyber-threats within corporate networks. The requirements to monitor threats and update threat data repositories, lists, and reports are labor-intensive activities (Ezeife, Dong, & Aggarwal, 2008). These demands are primarily focused on detecting, and not preventing, a cyber-threat intrusion into a company’s or agencies’ Information Technology (IT) environment, i.e., network. They further describe the need to maintain threat signature databases, used to identify threats, as requiring “a lot of human involvement” (Ezeife, Dong, & Aggarwal, 2008, p. 98).
The Defense Industrial Base (DIB) provides contract goods and services to the Department of Defense (DOD) (Hensel, 2016). These companies must balance national security protection requirements with their financial stability (Hensel, 2016). They too face growing demands to protect sensitive DOD provided information and secure their networks from cyber-threat nation-state actors such as China, Russia, and Iran (Starks, 2019).

Companies, to include those categorized as part of the United States (U.S.) DIB, rely heavily upon internal security, system, and antivirus logs, i.e., internal data, to identify risks and threats to a company as well as sensitive defense information (Zuech, Khoshgoftaar, & Wald, 2015). There is a need to include external data to supplement threat detection and prevention to protect the DOD and the nation’s data better (Hensel, 2016; Nagrecha & Chawla, 2016). External data exists outside the IT environment; it is exterior to the local computer network and can enhance an organization’s situational awareness and ability to respond to threats (Galloppo & Previati, 2014; Hassani & Renaudin, 2018; Nagrecha & Chawla, 2016).
External Datasets from active Honeypots could be further used to refine and improve the predictive analytics capability of
cyber-detection solutions.
The continued growth of data science and its ability to access large volumes of information have companies at all levels embracing these capabilities to better provide value and insight to organizational leadership and stakeholders alike (Nagrecha & Chawla, 2016). Data provides greater depth for a company or agency “to lower the costs, resulting in a higher overall Return on Investment” and improve overall efficiencies (Nagrecha & Chawla, 2016, p. 1). Companies are quickly accepting data science methods and tools to “not only deliver value from their internal data but also connect their internal data with external data sources to develop a more complete data profile [of the threat]” (Nagrecha & Chawla, 2016, p. 1).
Introduction
The problem. Commercial Artificial Intelligence (AI)-cybersecurity defense tools are primarily built to use and exploit internal data for threat detection (Columbus, 2019; Schroer, 2019). Current software and hardware solutions need to address this shortfall. Companies seldom rely upon the addition of external data such as data from active Honeypots (Nagrecha & Chawla, 2016). Overall, the lack of the use of external data identifies a gap in organizational cyber-defenses that could be closed through an applied, conceptual, framework to address this challenge.
Current studies. Forbes (Columbus, 2019) identifies ten major cybersecurity firms in 2019, applying AI solutions to enhance cybersecurity protections. Statistical analysis of the article identifies at least 70% percent are focused only on end-point detection (Columbus, 2019); these solutions are only attentive to threats that have already penetrated the corporate perimeter firewall or other local network defenses. Most commercial solutions are specifically focused on internal network traffic as a means to detect threat activity (Columbus, 2019).
Existing deficiencies. Daily, there are federal agencies and companies around the globe who are regularly hacked by cyber-thugs. Computer attacks frequently happen to even the most technically savvy companies such as Eurofins, a United Kingdom’s (U.K.) based company in 2019. It paid an undisclosed amount of money to hackers to regain access to their databases and records’ repositories; this information is vital to Britain’s primary criminal forensics support firm to the U.K.’s law enforcement departments (Devlin, 2019). Technically capable companies still fail against such cyber-assaults repeatedly.
Regular intrusions into critical U.S. federal systems highlight ever agile and highly impactful effects of cyber-threats worldwide. The 2015 Office of Personnel Management (OPM) was one of the most extensive and damaging exfiltrations of U.S. government personnel data in history (Koerner, 2016; Naylor, 2016). Also, interruptions have even impacted the supposedly highly protected networks of the DOD. “For nearly a week, some 4,000-key military and civilian personnel working for the Joint Chiefs of Staff [had] lost access to their unclassified email after what is now believed to be an intrusion into the critical Pentagon server that handles that email network” (Starr, 2015); the ability to better detect and prevent cyber-attacks has still not improved.
Problem
Commercial AI-based cyber-detection solutions are failing to integrate external data into their respective predictive analysis approaches (Columbus, 2019; Nagrecha & Chawla, 2016; Zuech et al., 2015). Any solution needs to be holistic.
The problem is not the lack of availability of data, but access to the right kinds of data and information critical to network defensive operations.
Purpose
The purpose of any study would be test the theory of the application of additional data, specifically Honeypot datasets, will provide a more exceptional ability to detect and prevent cyber-attacks better and sooner. Any study using operational external datasets and internal security logs to identify the measurable improvements with the addition of external datasets.
Theoretical and Conceptual Frameworks
The approach behind such a theoretical framework is best described by Nagrecha and Chawla’s (2016) suggestion of future work. They suggest that future researchers need to consider “the acquisition of external feature data occurs for all instances” to aid corporate decision-makers as a worthy effort since it provides value to an organization (Nagrecha & Chawla, 2016, p. 15).
Significance of a Study
The importance of such a study is the value of external Honeypot datasets provided in conjunction with internal data and its associated analysis (Nagrecha & Chawla, 2016). Additionally, the banking community has recognized that a wide range of available data is vital to ensuring financial institution growth and stability (Hassani & Renaudin, 2018). Hassani and Renaudin (2018) identify that “multiple sources [both internal and external] …increase the robustness, stability, and conservatism of the final capital evaluation [to make better decisions]” (Hassani & Renaudin, 2018, p. 2). Multiple sources, to include, e.g., Honeypot information, not only contributes to an understanding of the issues and risks beyond the localized data housed within a bank’s data storage servers but addresses the need for having value-based data to make informed organizational choices (Galloppo & Previati, 2014).
Conclusion
Halladay (2013) describes that data science allows for the ability to segregate customers that are either good or bad risks—a key data science strength (Halladay, 2013). Its capability in this study to categorize the good guys from the bad guys is an inherent strength of modern-day data analytic toolsets. Halladay (2013) highlights the importance of data science, and such segmentation value provides the highest return on investment to businesses (Halladay, 2013). He concludes with several observations that include: 1) industry is currently using predictive analytics in an ad hoc manner, and 2) the “resultant information must be actionable” (Halladay, 2013, p. 4). Data science offers excellent possibilities for the cybersecurity community to defend its precious IT systems and infrastructures better.
Finally, Honeypot data would better help to identify cyber-threats. It would accomplish this by employing expanded predictive analytics and insertion of a more comprehensive array of outside data (Gupta & Rani, 2018). Halladay (2013) explores the value of predictive analytics in the area of equipment leasing and the associated financial industry supporting this business segment; his view is much more pragmatic than academic. He sees predictive analytics as needed in the commercial sector for making similar judgments about risk—which also includes threats to corporations and agencies (Halladay, 2013).
The value and capabilities of data science tools cannot be ignored in a continuous battle with global cyber-threats for the foreseeable future.
References
Columbus, L. (2019, June 16). Top 10 cybersecurity companies to watch in 2019. Forbes. Retrieved from https://www.forbes.com/sites/louiscolumbus/2019/06/16/top-10-cybersecurity-companies-to-watch-in-2019/#4b683b696022
Devlin, H. (2019, July 5). Hacked forensic firm pays ransom after malware attack. The Guardian. Retrieved from https://www.theguardian.com/science/2019/jul/05/eurofins-ransomware-attack-hacked-forensic-provider-pays-ransom
Ezeife, C. I., Dong, J., & Aggarwal, A. K. (2008). SensorWebIDS: A web mining intrusion detection system. International Journal of Web Information Systems, 4(1), 97–120. Retrieved from doi:http://franklin.captechu.edu:2123/10.1108/17440080810865648
Galloppo, G., & Previati, D. (2014). A review of methods for combining internal and external data. The Journal of Operational Risk, 9(4), 83–103. Retrieved from https://franklin.captechu.edu:2074/docview/1648312043?accountid=44888
Gupta, D., & Rani, R. (2018). A study of big data evolution and research challenges. Journal of Information Science, 1–19. Retrieved from https://doi.org/10.1177/0165551518789880
Halladay, S. D. (2013). Using predictive analytics to improve decisionmaking. The Journal of Equipment Lease Financing (Online), 31(2), 1–6. Retrieved from https://franklin.captechu.edu:2074/docview/1413251757?accountid=44888
Hassani, B. K., & Renaudin, A. (2018). The cascade bayesian approach: Prior transformation for a controlled integration of internal data, external data and scenarios. Risks, 6(2), 1–17. Retrieved from doi:http://franklin.captechu.edu:2123/10.3390/risks6020047
Hensel, N. (2016). The defense industry: Tradeoffs between fiscal constraints and national security challenges. Business Economics, 51(2), 111–122. doi:http://franklin.captechu.edu:2123/10.1057/be.2016.16
Koerner, B. (2016, October 23). Inside the cyberattack that shocked the US government. Wired. Retrieved from https://www.wired.com/2016/10/inside-cyberattack-shocked-us-government/
Lyngaas, S. (2019, April 23). Someone is spoofing big bank IP addresses-possibly to embarrass security vendors. Cyberscoop. Retrieved from https://www.cyberscoop.com/spoofed-bank-ip-address-greynoise-andrew-morris-bank-of-america/
Nagrecha, S., & Chawla, N. V. (2016). Quantifying decision making for data science: From data acquisition to modeling. EPJ Data Science, 5(1), 1–16. Retrieved from doi:http://franklin.captechu.edu:2123/10.1140/epjds/s13688-016-0089-x
Naylor, B. (2016, June 6). One year after OPM data breach, what has the government learned? Retrieved from National Public Radio: https://www.npr.org/sections/alltechconsidered/2016/06/06/480968999/one-year-after-opm-data-breach-what-has-the-government-learned
Rodriguez, L., & Da Cunha, C. (2018). Impacts of big data analytics and absorptive capacity on sustainable supply chain innovation: A conceptual framework. LogForum, 14(2), 151–161. Retrieved from doi:http://franklin.captechu.edu:2123/10.17270/J.LOG.267
Schroer, A. (2019, April 10). 25 Companies merging AI and cybersecurity to keep us safe and sound. Built-In. Retrieved from https://builtin.com/artificial-intelligence/artificial-intelligence-cybersecurity
Shaikh, F. (2016, October 3). Deep learning guide: Introduction to implementing neural networks using TensorFlow in Python. Analytics Vidhya. Retrieved from https://www.analyticsvidhya.com/blog/2016/10/an-introduction-to-implementing-neural-networks-using-tensorflow/
Starks, T. (2019, July 9). Cyber incidents were expensive in 2018. Politico. Retrieved from https://www.politico.com/newsletters/morning-cybersecurity/2019/07/09/cyber-incidents-were-expensive-in-2018-675243
Starr, B. (2015, July 31). Military still dealing with cyberattack ‘mess.’ CNN. Retrieved from https://www.cnn.com/2015/07/31/politics/defense-department-computer-intrusion-email-server/index.html
Warwick, K. (2010). Cultured neural networks. Proceedings of the Institution of Mechanical Engineers, Part I: Journal of Systems and Control Engineering, 224(2), 109–111. Retrieved from https://doi.org/10.1243/09596518JSCE916
Zuech, R., Khoshgoftaar, T. M., & Wald, R. (2015). Intrusion detection and big heterogeneous data: A survey. Journal of Big Data, 2(1), 1–41. Retrieved from doi:http://franklin.captechu.edu:2123/10.1186/s40537-015-0013-4
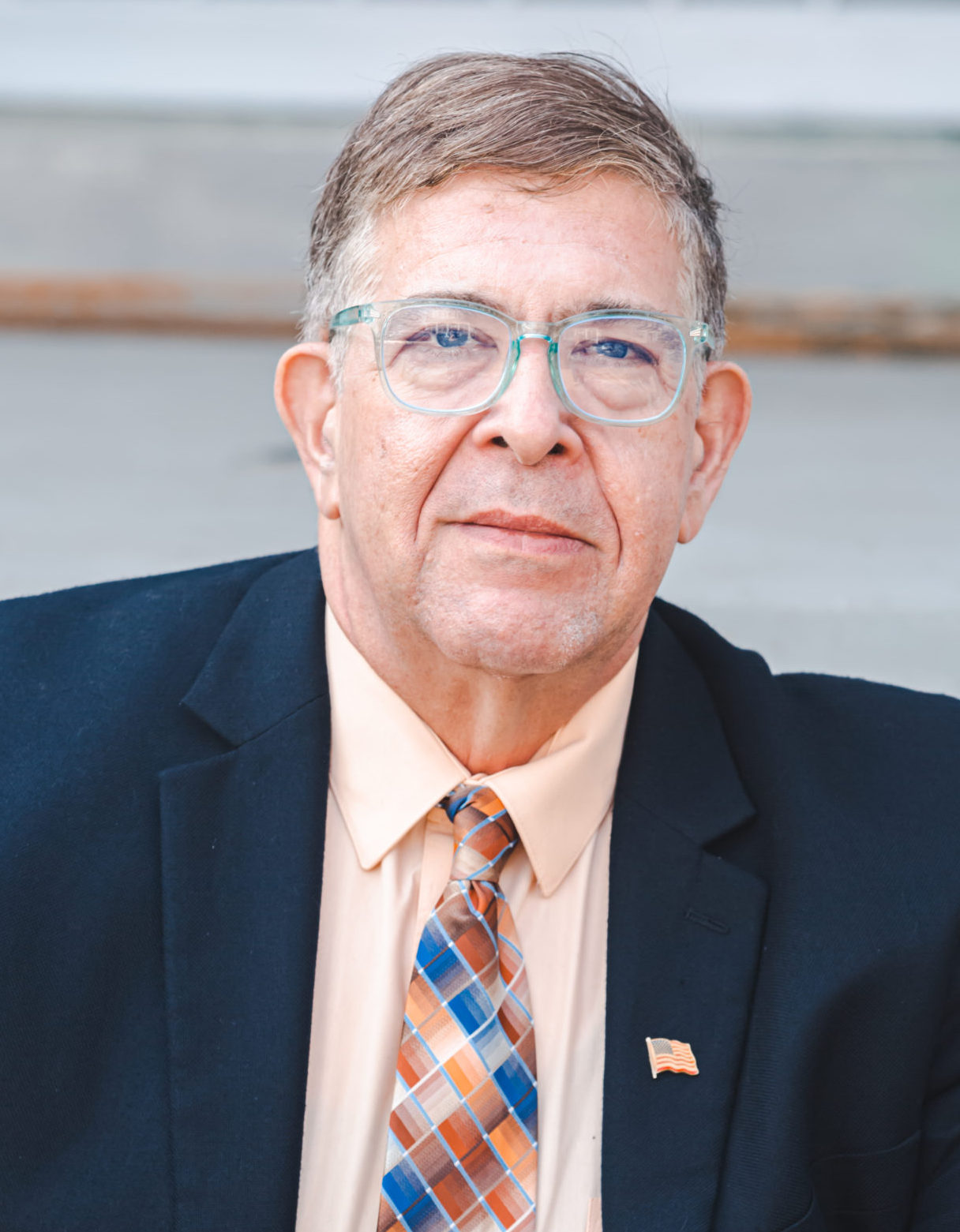
Dr. Russo is currently the Senior Data Scientist with Cybersenetinel AI in Washington, DC. He is a former Senior Information Security Engineer within the Department of Defense’s (DOD) F-35 Joint Strike Fighter program. He has an extensive background in cybersecurity and is an expert in the Risk Management Framework (RMF) and DOD Instruction 8510, which implement RMF throughout the DOD and the federal government. He holds a Certified Information Systems Security Professional (CISSP) certification and a CISSP in information security architecture (ISSAP). He has a 2017 Chief Information Security Officer (CISO) certification from the National Defense University, Washington, DC. Dr. Russo retired from the US Army Reserves in 2012 as a Senior Intelligence Officer.