TOP 7: “Cyber-Analytics” Journal Articles (2019)
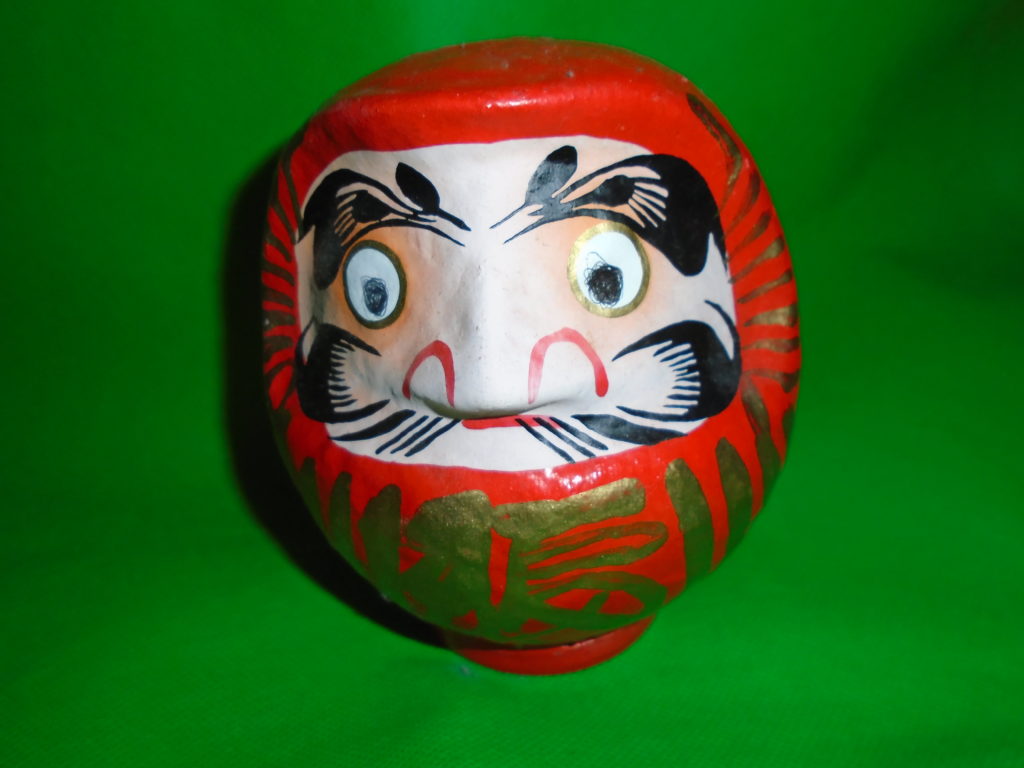
It’s time we measure how well we are really doing with Cybersecurity using Data Science
“Cyber-Analytics: The application of data science models and principles to provide quantitative measures on the successes or failures of cybersecurity end states.”
1
Anagnostopoulos, C. (2016). Quality-optimized predictive analytics. Applied Intelligence, 45(4), 1034-1046.
Anagnostopoulos (2016) offers a “quality-aware mechanism” and mathematical model for better managing contextual data before delivery to the targeted Information System (Anagnostopoulos, 2016, p. 1035). He particularly focuses his work around the sensory Internet of Things’ (IoT) devices such as fire-event monitoring and energy management solutions. He does this by introducing a delay in the pre-processing of the contextual data (“How hot is the fire?) with the associated meta-data (“What is the ambient temperature and humidity?) within the confines of the developed mathematical model.
His approach is intended to reduce any data bias such as outlier information real or not. This is done by applying a function, a typical math function of f(x), such as the use of linear regression to improve data quality—essentially delivering good data to the user. The author concludes with his mathematical efforts using “optimal stopping theory” (delay mechanism) to provide better and more actionable results with minimal biases (Anagnostopoulos, 2016, p. 1045).
COMMENT: What makes this article unique is that it parallels the human process, if so chosen, to ponder the available data, and consider innate biases to optimal decision-making.
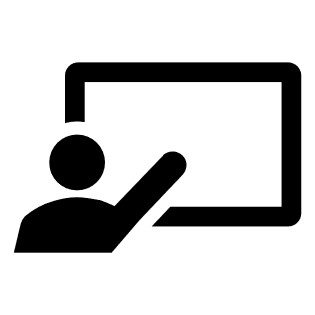
2
Anitha, P., & Patil, M. M. (2018). A review of data analytics for supply chain management: A case study. International Journal of Information Engineering and Electronic Business, 10(5), 30-39.
Anitha and Patil (2018) attempt to explain the interconnection between data science and Supply Chain Management (SCM). They describe this merger as “supply chain analytics” which is the application of data analysis to SCM issues to predict outcomes by considering the quality of the data (Anitha & Patil, 2018, p. 30). They further discuss the challenges of supply chain susceptibility alluding to its overall in adequately measuring SCM results such as component, supplier, and process complexities. They describe the three types of data analytics that include: 1) descriptive (answering the what happened), predictive (answering the when something will occur), and prescriptive analytics (answering the why something occurred) from the collected data.
The article provides multiple example techniques for the reader to become familiar. They conclude with the assertion from their study that linear regression provides predictive answers within SCM 80% closer to the available results and is therefore a useful tool to SCM overall (Anitha & Patil, 2018). (Also, see: K & Shivakumar, 2014).
COMMENT: Weaknesses in the article include not further clarifying the susceptibility issues, and merely describing the complexities as broad complexity topics, i.e., component complexities, supplier complexities, etc. (Anitha & Patil, 2018, p. 31). Otherwise, this remains our 2019 pick. 🙂
3
Hair, Joe F., Jr. (2007). Knowledge creation in marketing: The role of predictive analytics. European Business Review, 19(4), 303-315.
Hair (2007) discusses predictive analytics and how it is applied to knowledge creation in the area of marketing. He describes the importance of a knowledge-based economy, and how it relies upon information that must be actionable. Hair (2007) notes the historical accumulation of information that has been lost or discarded in the past, and now how it is seen as valuable for organizations, businesses, and researchers alike. He identifies that predictive analytics is not a revolutionary approach and dates to 1920s.
Hair (2007) further discusses the access to the volumes of data will increase the ability of businesses to better identify, understand and predict customer needs and market developments. He anticipates that in the future (remember, this was written over a decade ago) predictive analytics will rely on “mixed data,” i.e., structured, and unstructured data (Hair, 2007, p. 312). The author concludes with the essential nature of predictive analytics and the ongoing demand for more accurate and refined information to support marketing knowledge creation. (Hair, 2007).
COMMENT: The strength of this article is that it identified the perceptive concerns of the public about the corporations having access to their data, and potentially abusing that information for more than advertising purposes.
4
Halladay, S. D. (2013). Using predictive analytics to improve decisionmaking. The Journal of Equipment Lease Financing (Online), 31(2), 1-6.
Halladay (2013) explores the value of predictive analytics for the equipment leasing and the associated financial industry supporting this business segment. His view is much more pragmatic than academic. He sees predictive analytics as needed in the commercial sector for making better judgments. Additionally, he describes that data science allows for segmentation of customers which is its key strength; which customers are good return buyers, and which are likely to default on leases? Halladay (2013) highlights the importance of data science and such segmentation’s value to provide the highest return on investment to businesses.
He concludes with several observations that include: 1) industry is currently using predictive analytics in an ad hoc manner—yet, not well-established, and 2) the resultant information is not always actionable. He interestingly concludes with the essential nature of leadership’s acceptance and willingness to act upon the data. “Management has to not only preach it but also live it” (Halladay, 2013, p. 5).
COMMENT: Its strength is its introduction as a business case to the reader for a seemingly esoteric topic such as equipment leasing; it highlights the application beyond just the technical or scientific.
5
K, P. C., & Shivakumar, B. L. (2014). A review of trends and technologies in business analytics. International Journal of Advanced Research in Computer Science, 5(8), 225-229.
K and Shivakumar (2014) describe data analytics as practices, skills, and technologies for the study of business information (and intelligence) designed to support corporate decision-making. The article discusses the insights, and intelligence produced from the Big Data sets collected and analyzed as part of the growing efforts in data science. The authors’ focus, based upon the articles business orientation, is upon actionable findings that can improve business decisions and increase profitability. They specifically discuss the three types of analytics: 1) descriptive, 2) predictive, and 3) prescriptive; neither of these is any more important and provide answers to real-world analytical questions based upon the customer’s needs.
Specific to predictive analytics, the authors classify it into six categories: data mining, pattern recognition and alerts, Monte-Carlo simulations, forecasting, root cause analysis, and predictive modeling; all aspects designed to improve managers ability to make better decisions. The three analytics types afford business intelligence operations to extract a complete picture insight into what has happened with an objective to determine what may happen in the future (K & Shivakumar, 2014). (Also, see: Anitha & Patil, 2018).
COMMENT: A key strength is Figure 3 (K & Shivakumar, 2014, 228), Understanding Analytics, provides a plain-English diagram of what the three branches of analytics can afford the business owner.
6
Lee, A. J., Ph.D. (2015). PREDICTIVE ANALYTICS: The new tool to combat fraud, waste and abuse. The Journal of Government Financial Management, 64(2), 12-16.
Lee (2015) calls for a systems approach for projects requiring data modeling especially those in the areas of fraud and abuse. He sees predictive analytics as a process that requires a series of methodologies to increase awareness and actionability. It requires a combination of statistical sampling, modeling, and estimation. In his Predictive Analysis System (PAS), he begins with 1) data collection and sampling, 2) model estimation—what are the likely results, 3) prediction and model evaluation—using more refined statistical analysis to produce better results, and 4) sampling and modeling adjustments—identify outlier data and extraneous bias.
Lee (2015) suggests that the application of such an approach will lead to greater effectiveness, efficiency, and transparency in the realm of fraud prediction. He concludes with the need that such processes are well-documented so the outputs can be understood at every level—from business analyst to data scientist (Lee, 2015).
COMMENT: The strength of this article is the introduction of PAS. While not novel to the area of data science, the article itself helps the beginner data scientist to understand the various actions that must occur with data collection, processing, and reporting.
7
Rodriguez, L., & Da Cunha, C. (2018). Impacts of big data analytics and absorptive capacity on sustainable supply chain innovation: A conceptual framework. LogForum, 14(2), 151-161.
Rodriguez and Da Cunha’s (2018) article discusses the interrelationship between Big Data and Predictive Analytics (BDPA), and its ability to affect sustainability. BDPA is “a holistic approach to manage, process and analyze data regarding high volume, variety, velocity, veracity, and value to create actionable insights…” (Rodriguez & Da Cunha, 2018, p. 152). Absorptive capacity is the organizational ability to gather the requisite data, and as the authors describe, create both value and wisdom. The authors offer a conceptual framework that links the ability of BDPA to support overall performance and innovation outputs.
The four elements include 1) innovation drivers—increased profitability, sustainability, etc., 2) new technologies—to include the predictive analytics of emerging data science tools and capabilities, 3) the relevance—that continues the awareness of profitability and social impacts, and 4) absorptive capacity—can the company accept, store, and process the data. They conclude that their framework positively connects sustainability, BDPA, and absorptive capacity (Rodriguez & Da Cunha, 2018).
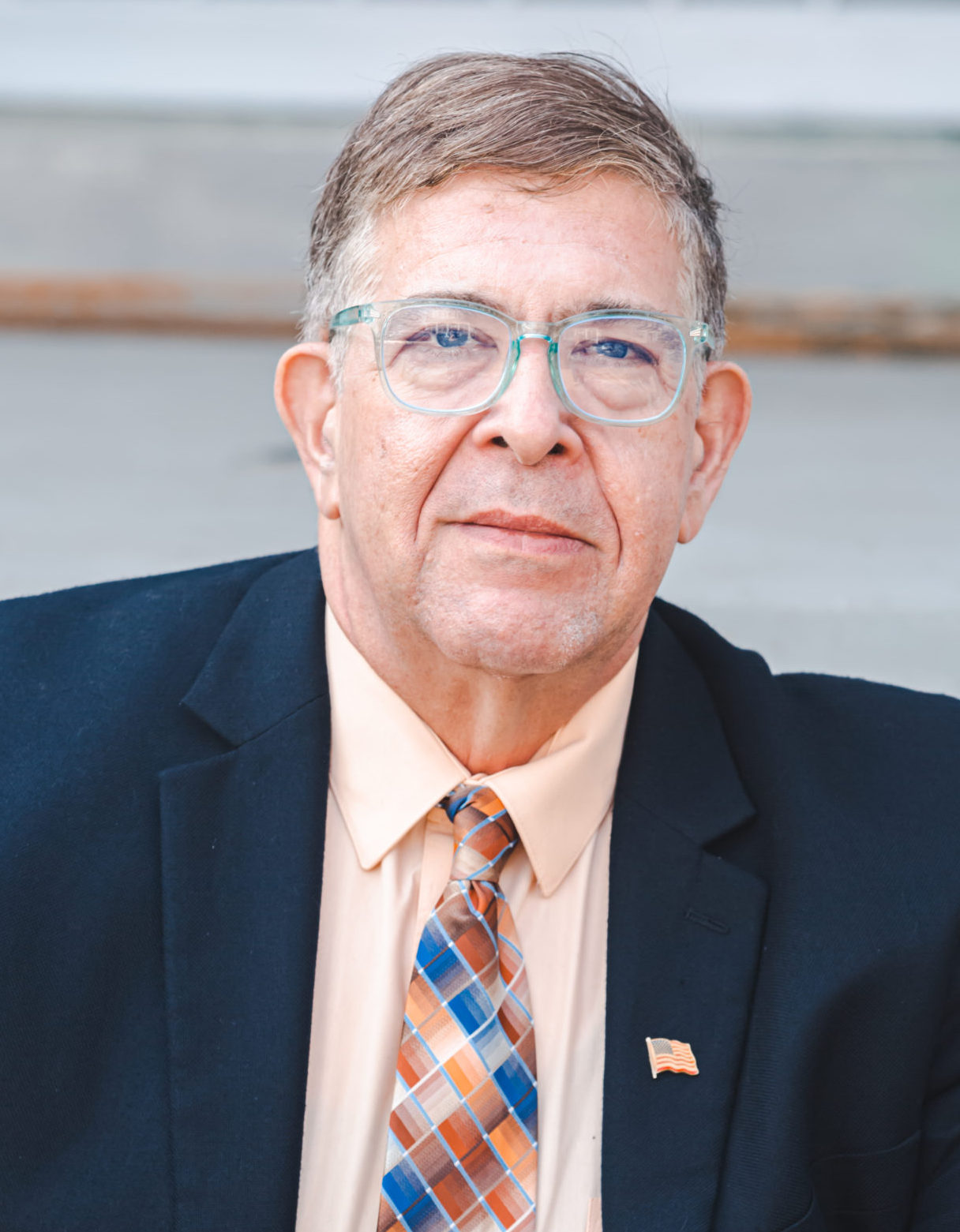
Dr. Russo is currently the Senior Data Scientist with Cybersenetinel AI in Washington, DC. He is a former Senior Information Security Engineer within the Department of Defense’s (DOD) F-35 Joint Strike Fighter program. He has an extensive background in cybersecurity and is an expert in the Risk Management Framework (RMF) and DOD Instruction 8510, which implement RMF throughout the DOD and the federal government. He holds a Certified Information Systems Security Professional (CISSP) certification and a CISSP in information security architecture (ISSAP). He has a 2017 Chief Information Security Officer (CISO) certification from the National Defense University, Washington, DC. Dr. Russo retired from the US Army Reserves in 2012 as a Senior Intelligence Officer.