TOP 5: The Main Implications of ML to Help Deter Cyber-threats
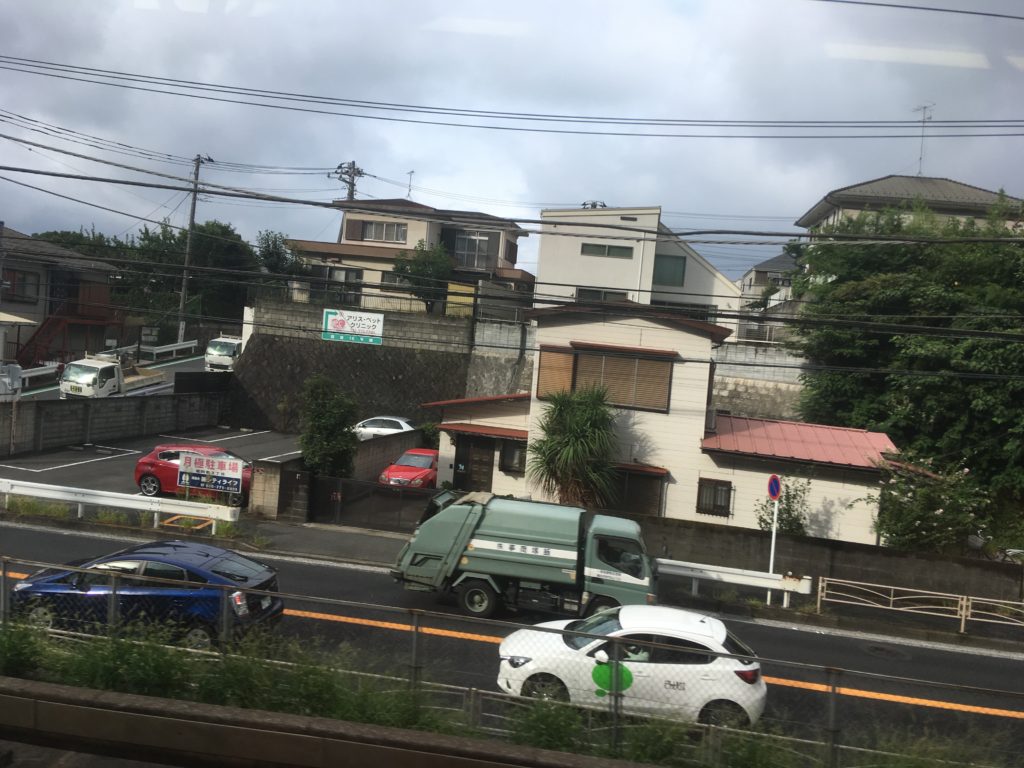
SUSCEPTIBILITY OF DATA INTERNAL AND EXTERNAL TO THE DATA ANALYTIC EFFORT…INSIDE AND OUTSIDE “THE WIRE”
Data poisoning, also known as adversarial poisoning or data injection attacks, involves injecting malicious or corrupted data into a machine learning model’s training data to disrupt or manipulate the model’s performance. This attack can have severe implications for the future application of machine learning tools. It can lead to incorrect or biased decision-making, compromise the confidentiality and integrity of data, and undermine trust in machine learning systems. Here are five main implications of data poisoning for the future of machine learning:
- Incorrect or biased decision-making: One of the main goals of machine learning is to make accurate and reliable predictions or decisions based on data. However, suppose a machine learning model has been poisoned with malicious data. In that case, it may make incorrect or biased decisions, leading to negative consequences such as financial losses or harm to individuals. For example, a machine learning model used for credit risk assessment may make biased decisions based on poisoned data, leading to discrimination against certain groups of people.
- Compromised confidentiality and integrity of data: Data poisoning attacks can also compromise the confidentiality and integrity of data, as the injected malicious data can spread throughout the machine learning model and affect the accuracy of its predictions or decisions. This can lead to the leakage of sensitive information or data manipulation for nefarious purposes.
- Undermining trust in machine learning systems: Data poisoning attacks can also undermine trust in machine learning systems, leading to incorrect or biased decisions that can have serious consequences. This can result in a lack of confidence in the reliability and accuracy of machine learning models, which can hinder their adoption and use in various fields.
- Need for improved security measures: To mitigate the risks of data poisoning attacks, it is essential to implement robust security measures to protect machine learning models and data. This may include measures such as data sanitization, data quality checks, and secure training environments.
- Increased awareness and education: Data poisoning is a complex and evolving threat that requires increased awareness and education to help deter and stop these attacks. This includes educating developers and users about the risks and implications of data poisoning and the measures that can be taken to prevent or mitigate these attacks.
Data poisoning is a severe threat to the future application of machine learning tools. It can lead to incorrect or biased decision-making, compromise the confidentiality and integrity of data, and undermine trust in machine learning systems. To address this threat, it is essential to implement robust security measures and increase awareness and education about data poisoning and its potential consequences.
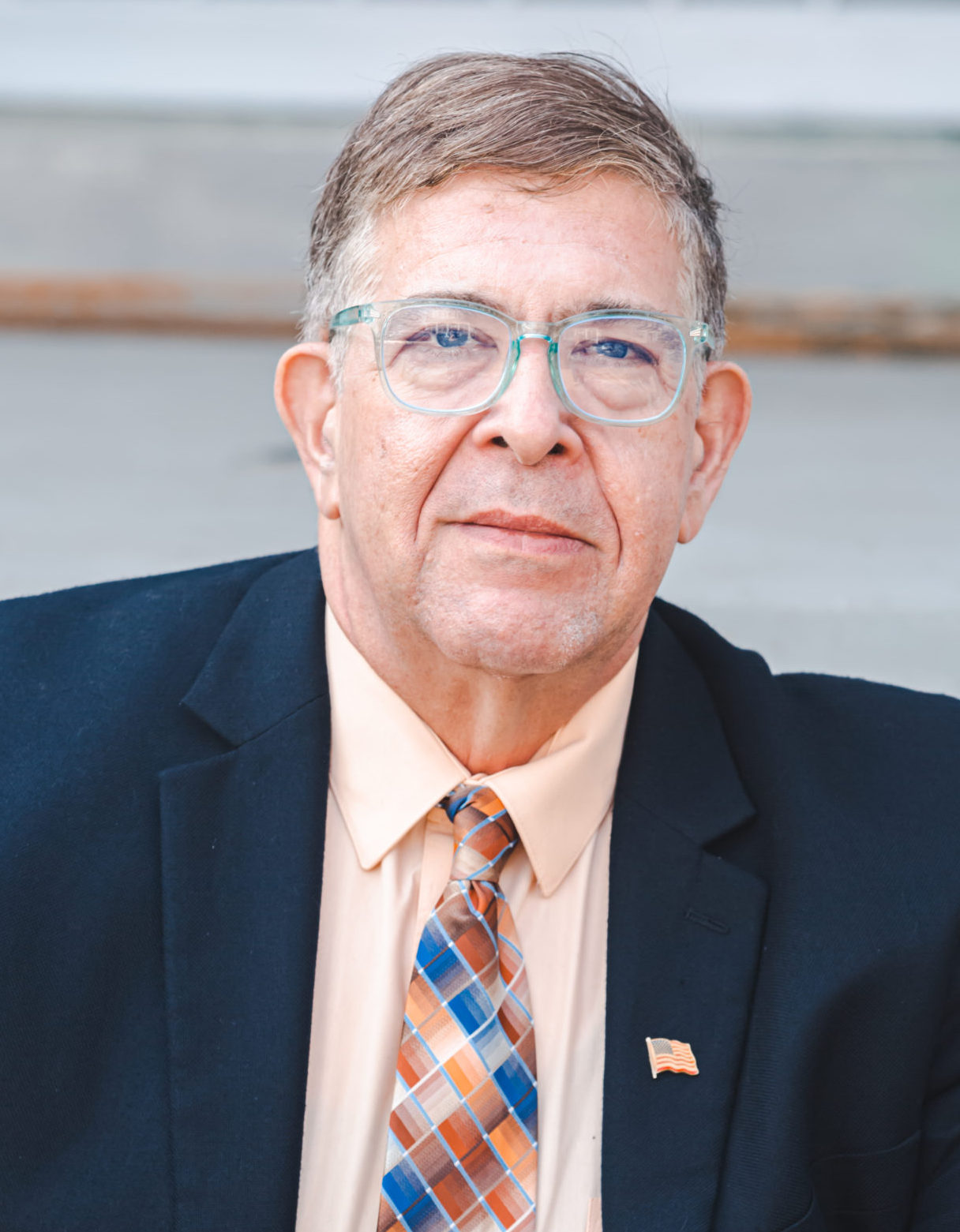
Dr. Russo is currently the Senior Data Scientist with Cybersenetinel AI in Washington, DC. He is a former Senior Information Security Engineer within the Department of Defense’s (DOD) F-35 Joint Strike Fighter program. He has an extensive background in cybersecurity and is an expert in the Risk Management Framework (RMF) and DOD Instruction 8510, which implement RMF throughout the DOD and the federal government. He holds a Certified Information Systems Security Professional (CISSP) certification and a CISSP in information security architecture (ISSAP). He has a 2017 Chief Information Security Officer (CISO) certification from the National Defense University, Washington, DC. Dr. Russo retired from the US Army Reserves in 2012 as a Senior Intelligence Officer.