CRITIQUE: The Problems with the Scientific-based Risk Metrics Methodology
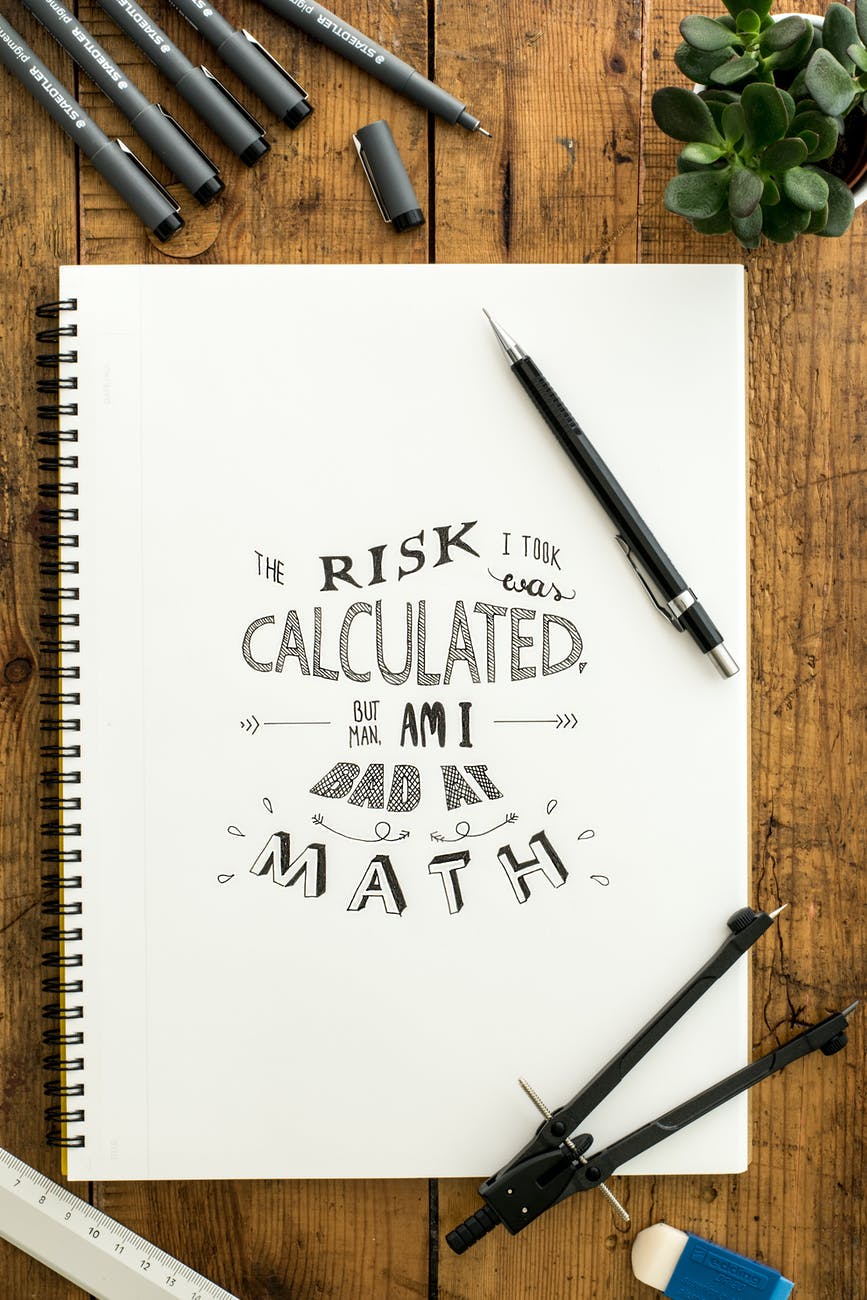
Photo by Lum3n on Pexels.com
Applying the Qualitative to Frame Quantitative Analysis
Abstract
A significant challenge facing businesses and agencies is how to focus limited resources and dollars against the relentless cybersecurity attacks against their networks and data. Watkins and Hurley (2015; 2016) propose a means to score and prioritize technology vulnerabilities using what they describe as Scientific-Based Risk Metrics. While portrayed as quantitative, their approach has several foundational subjective-based weaknesses, which diminish the overall objective of their solution. This critique investigates Watkins and Hurley’s quantitative and qualitative study efforts and highlights those flaws that dilute their innovative work. While their analysis identifies a well-defined and logical model, much of their supporting back-end analytics fail to reinforce a complete and more comprehensive scientific and statistical approach to prioritizing security vulnerabilities.
Approximately “48% [of organizations] attempt to quantify the damage a cyberattack could have” on their business (Lis & Mendell, 2019, p. 27). This estimate does not provide a complete picture of the types and magnitudes of costs beyond more than the loss of operational downtime and the inconvenience to users to access their corporate data. Tangible and intangible costs of implementing defensive measures, such as those afforded by quantitative or qualitative solutions, are seldom fully employed or vetted for completeness and accuracy (Lis & Mendell, 2019). For example, Hubbard and Seiersen (2016) describe that subjective measures, such as the Risk Matrix, have been masquerading as supposed defined measured solutions where they are not. The cybersecurity community has, unfortunately, been engaged in quasi-quantitative analysis to hide its lack of quantitative certainty (Hubbard & Seiersen, 2016).
This review of two related quantitative analytic reports by the same authors, Cyber Maturity as Measured by Scientific-Based Risk Metrics (Watkins & Hurley, 2015) and The Next Generation of Scientific-Based Risk Metrics (Watkins & Hurley, 2016), offer a quantitative means to score and prioritize Information Technology (IT) security vulnerabilities.
This analysis agrees with their strategic objective to create a model to rank vulnerabilities; however, it highlights many tactical weaknesses in their supporting quasi-quantitative assumptions and considerations. This recognition raises doubt about the selected authors’ depth in terms of their overall scientific approach (Creswell & Creswell, 2018). While their Scientific-Based Risk Metrics (SBRM) methodology positively reflects upon the challenge of effective cybersecurity risk measures in creating a relative scoring application, their research also underscores a problem with the use of subjective foundational qualitative measures that distract from their overall work.
Measurability is needed for senior corporate or agency management to understand and better resolve the challenges of cybersecurity attacks against their data and networks (Association of International Certified Professional Accountants [AICPA], 2017). A key example of existing quasi-quantitative methods is the use of the qualitative (and long-accepted) Risk Matrix (RM) (Hubbard & Seiersen, 2016; Russo, 2018). The RM is a specific representation of one example from the cybersecurity community that contributes to creating invalid and subjective quantification of the dangers and costs of cyber-attacks (Hubbard & Seiersen, 2016).
The Illusion of Quantification
The RM is a historical decision-support tool used to describe the level of risk, most commonly in project and program management and, more recently, in the field of cybersecurity (Russo, 2018). RMs are used to capture a subjective graphical representation of the levels of risk that exist during the lifecycle of a system’s development and maintenance, see Figure 1. The RM is one of the pitfalls requiring attention. There is a need to focus on the realistic quantification of cybersecurity threats faced by organizations (Hubbard, 2020; Hubbard & Seiersen, 2016). Ultimately, this acknowledged standard for risk management has caused a false sense of security in the field of cybersecurity defense, and the cybersecurity community has not effectively employed and understood actual quantitative methodologies.

Figure 1. Risk Matrix. Adapted from “Risk Assessment Methodology,” by University of Melbourne, 2018, Risk Assessment Methodology, p. 2. Copyright 2018 by the University of Melbourne.
The significance of measurability. Hayden (2010) states that “established metrics programs…still struggle with understanding their security efforts” (p. 5). To understand and address a problem, it is necessary to apply metrics that afford a consistent quantification of that problem—either positively or negatively, to understand the impacts to the organization (Prusak, 2010). Companies create cybersecurity programs, but they have been nothing more than data collection efforts without little attempt to identify the real measured damages and costs of applying or not applying a solution (Hayden, 2010). Measurability affords the effective use of data and has demonstrated operational and security efficiencies for both the public and private sectors (Lee, 2015)
As Peter Drucker, the acclaimed author on the topic of modern business, stated, “what gets measured gets managed” (Prusak, 2010, para. 5). The achievement of any endeavor in cybersecurity protection is that there must be deliberate evidence to substantiate a claim of success, and it needs to be based upon comprehensive scientific approaches (Cooper, 2018; Hubbard & Seiersen, 2016). If there are defined measures of success and failure, it likely will benefit an organization’s viability to remain secure, productive, and profitable (Russo, 2018).
Fixing Trust Among the Triad
Watkins and Hurley (2015; 2016) confusingly attempt to resolve the security trust between the citizenry, the defense complex, and the intelligence community to create needed confidence between these significant players regarding effective cybersecurity measures. They try to juxtapose that their solution will resolve trust, at least in part. They assert that if their outputs are transparent and explain to all the triad members the state of operational risk, their method will fix the trust between these parties.
Specifically, this discussion is out of place and neglects to identify other vital individuals, groups, and organizations. They fail to address, for example, the global community and extended businesses feeding the supply chains that must understand and abide by their proposed solution. Qualitatively, this discussion, in both papers, appears to add nothing to the positive study direction of scoring computer vulnerabilities and risks. The authors should have remained focused on the practical significance of their work. Their solution forms the beginnings of better prioritization capabilities that would allow organizations to focus limited resources against their cybersecurity weaknesses, see Figure 2.
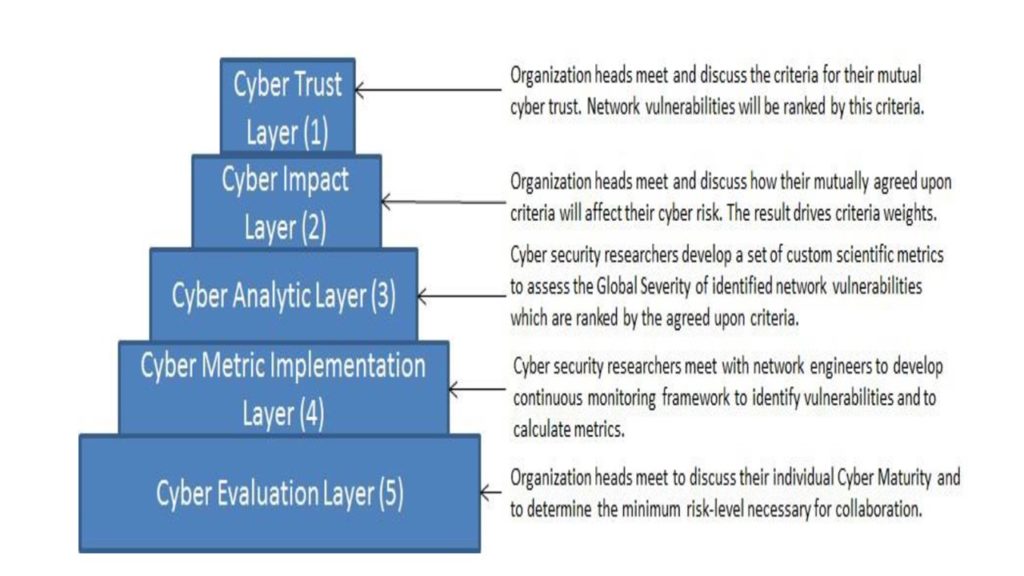
Figure 2. Watkins and Hurley proposed a five-layer Analytical Hierarchy Process (AHP) cyber maturity model. Adapted from “Cyber Maturity as Measured by Scientific-Based Metrics,” by LA Watkins & JS Hurley, 2015, Journal of Information Warfare, p. 59. Copyright 2015 by Journal of Information Warfare.
The authors also concentrate on new vulnerability assessments specific to network security alone. While their position is only partially correct, they fail to recognize the perspective of federal standards mandated for the Department of Defense (DOD) and Intelligence Community (IC) for networks and systems in 2014 (Department of Defense [DOD], 2014). The Risk Management Framework (RMF) became mandatory for the DOD, and most other federal agencies were already obliged to abide by the National Institute of Standards and Technology (NIST) guidance specific to identifying vulnerabilities through security control selection and oversight (National Institute of Standards and Technology [NIST], 2014; 2018). The authors did not recognize that even in 2015 and 2016, respectively, the standards were already directed by the federal government to ensure elements of trust and security. Such topic areas as reciprocity of system-to-system interconnection and continuous monitoring of IT environments were defined; matters of trust were already in progress at the time of publishing their works and should have been known by both authors (NIST, 2014; 2018).
Supervised Learning
Quantitative Analysis
One of the most glaring problems with the 2015 work is the singular self-selection by one of the authors as a Subject Matter Expert (SME), which may be inferred as a form of unconscious bias. Presumably, Watkins designates himself as the only SME that creates and establishes the relative factors of the computations for their solution (Watkins & Hurley, 2015). He describes that there is comparative importance of the availability of a vulnerability exploit is twice as important as the impact on the network and that the availability of the exploit is seven times more important than the age or existence of a specific exploit. Such presumptions were only based upon “the researchers’ experience in the field [of cybersecurity]” (p. 61). This approach is, at best, qualitative in assigning personal values and introduces incompleteness where there is little scientific basis. It was not until the 2016 work that the authors stated these assumptions could be applied to their measured solution. They never address the additional use of an expanded number of SMEs to provide greater back-end depth to their solution (Watkins & Hurley, 2015).
Additionally, Watkins and Hurley (2015; 2016) use the Analytical Hierarchy Process (AHP) methodology created by Saaty (1980). The AHP is considered a framework to separate varied tiers for relative scoring to create a semi-quantified solution, see Figure 2. The AHP is widely utilized throughout the academic community and affords a mechanism to score relative strengths by creating a defined approach to better categorize the layers of effort.
Kaušpadienė, Ramanauskaitė, and Čenys (2019), for example, selected AHP to help structure their decision-making process in creating a condensed version of a cybersecurity framework for both small and medium businesses. Their study explicitly establishes AHP because it enables users “to combine a consensus of expert groups” (p. 985). Kaušpadienė et al. (2019) attempt to ensure their supporting qualitative values from multiple SMEs rely upon a broader sample of experts and vice one. Further, suggesting that Watkins and Hurley (2015; 2016) have introduced a level of bias to their study, which is unrelated to the standard application of the AHP methodology (Saaty, 1980).
While Watkins and Hurley’s (2015; 2016) approach may be considered a good beginning, it needs better affirmation that there are more SMEs and introduces even more scientific consistency. Hubbard and Seiersen (2016) present the concept of a calibrated expert trained, tested, and certified to make quantitative predictions through the application and recognition of expected errors. Identifying errors by the SME helps to create value assessments of cybersecurity attack cost impacts within a defined 95% Confidence Interval (CI). Hubbard and Seiersen (2016) approach this problem far more scientifically. They offer the concept and the process to train calibrated SMEs from a statistical position grounded in a scientific approach.
Additionally, Hubbard and Seiersen (2016) suggest that human experts can be trained through a defined evaluative process to include a recognition of CI. Hubbard’s Applied Information Economics (AIE) programs offer businesses and agencies where risk management is critical an applied approach to have training calibrated SMEs to better ascertain the enumerated dangers and risks to cybersecurity infrastructures (Hubbard Decision Research [HDR], 2020). The approach trains experts to assess whether they are underconfident, overconfident, or have biases that prevent them from supporting quantitative analysis; in data science terms, these would be trained experts supporting supervised learning efforts.
Relative Scoring and Geometric Means
While there are several concerns about the principal authors’ base preparation for their work, they offer a valuable framework for businesses and agencies to employ against threat vulnerabilities. Smith’s (1988) study provides an interesting similarity with Watkins and Hurley’s (2015; 2016) work in his attempt to characterize computer performance to a singular value. Smith (1988) argues that a benchmarking amount is needed to assess varied computer performance levels, much like diverse threat vulnerabilities. In this discussion, consolidating values to create a particular measure is where the principal authors’ study offers significant benefit and practicality. The parallels can be extended to vulnerability identification to a singular aggregated value that could be applied to determine the relative threats among different vulnerabilities.
Smith (1988) and Watkins and Hurley (2015; 2016) use geometric means in their respective studies. Historically, geometric means have been recognized for providing consistency in like research and are beneficial in aggregating a representative score to determine relative levels of performance or threat, respectively (Smith, 1988). “Geometric means has been advocated for use with performance numbers that are normalized,” and it is such standardization that also occurs in Watkins and Hurley’s (2015; 2016) Scientific-Based Risk Metrics solution (p. 1204).
Watkins and Hurley (2015; 2016) employ geometric vice arithmetic means. They use the resultant value to create a ratio between the factors sought, such as exploit availability or age of exploit. In geometric means calculations, instead of adding the values and then dividing by the number of values provided, geometric means multiplies the values and subsequently applies the root function based upon the numbers by the product of those values, see Figure 3.
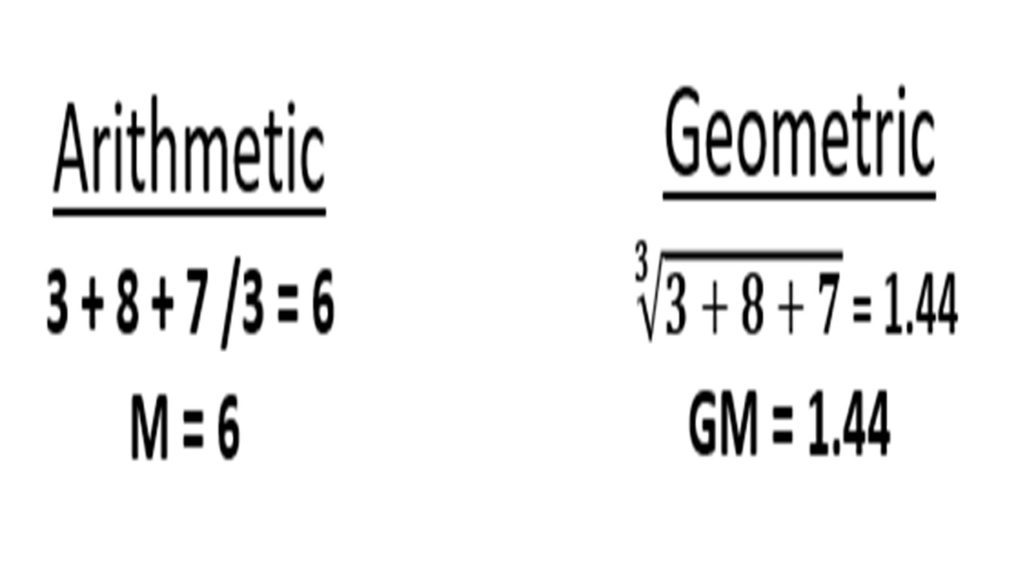
Figure 3. Arithmetic versus geometric means computations.
However, Smith’s (1988) work indicates that geometric means should only be applied after the aggregation of all the data and not before. This finding suggests a potential flaw in the primary authors’ application of their solution; however, it does not appear critical at this point in the data-processing phase. The geometric mean is then used to create a ratio of comparative importance for this baselining effort. The output is a final relative scoring or criteria ranking of the three selected factors, as found in Figure 4.

Figure 4. Pairwise comparison of scientific-based risk scoring initialization criteria. Adapted from “Cyber Maturity as Measured by Scientific-Based Risk Metrics,” by LA Watkins & JS Hurley, 2015, Journal of Information Warfare, 2015, p. 62. Copyright 2015 by Journal of Information Warfare.
Scientific-Based Risk Metrics Methodology
Watkins and Hurley (2015; 2016) use the criteria ranking, or weighting, to apply a qualitative determination of high (1.0), medium (0.5), or low risk (0.1) to their computations. These calculations are then used to a Symantec Threat database for the Common Vulnerability Scoring System (CVSS) scoring, see Figure 5. The output is a unit-less weight that can be related to other vulnerability scores to prioritize mitigation efforts and focus organizational resources to address the weaknesses en masse.
Unfortunately, this portion of the solution also injects an element of human subjectivity. Using simplified ratings such as high, medium, or low, raises more questions about what the categories of exploit availability, impact, and exploit age mean to organizational leadership. What are the other missing criteria? What does impact mean? While innovative, the method does not create a genuinely quantitative result for a diverse cyber-defense community.
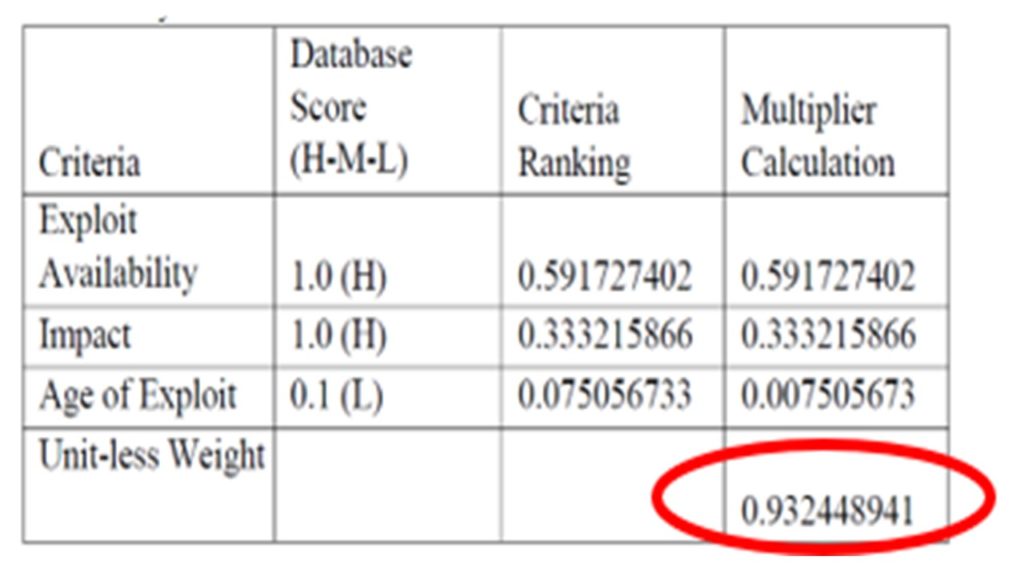
Figure 5. Unit-less weight scoring for the vulnerability (CVE-2014-6271). Adapted from “Cyber Maturity as Measured by Scientific-Based Risk Metrics,” by LA Watkins & JS Hurley, 2015, Journal of Information Warfare, 2015, p. 62. Copyright 2015 by Journal of Information Warfare.
Recommendation
The main recommendation for the approach’s improvement is to consider at all levels the assumptions that form the basis of their quantitative solution effort. Making quantitative assertions based on subjectivity reduces the overall purpose of Watkins and Hurley’s (2015; 2016) crucial effort. A researcher must apply rigor at all stages of a quantitative study to ensure completeness, reliability, and validity (Abbott, 2014; Adams & Lawrence, 2019; Creswell & Creswell, 2018).
Conclusion
The illusion of measurability persists throughout the cybersecurity community, posing a threat to practical methods of success against cyber threats (Hubbard & Seiersen, 2016; Under Secretary of Defense for Acquisition, Technology, and Logistics [USD-ATL], 2006). An organization’s ability to honestly know its security status is arbitrary at best. Measurability of the relative dangers of wide-ranging cybersecurity vulnerabilities must be substantiated by the complete quantitative foundations to support the overall objective—focusing on limited resources. Watkins and Hurley’s (2015; 2016) approach needs to have a comprehensive and coherent quantified basis to mitigate and eliminate the pains of global cyber threats.
References
Abbott, D. (2014). Applied predictive analytics: Principles and techniques for the professional data analyst. Indianapolis, IN: John Wiley & Sons.
Adams, K. A., & Lawrence, E. K. (2019). Research methods, statistics, and applications
(2nd ed.). Thousand Oaks, CA: Sage Publications.
Association of International Certified Professional Accountants. (2017, April 15). Description criteria for management’s description of the entity’s cybersecurity risk management program. Retrieved from https://www.aicpa.org/InterestAreas/FRC/AssuranceAdvisoryServices/DownloadableDocuments/Cybersecurity/Description-Criteria.pdf
Cooper, H. (2018). Reporting quantitative research in psychology: How to meet APA style journal article reporting standards. Washington, DC: American Psychological Association.
Creswell, J. W., & Creswell, J. D. (2018). Research design: Qualitative, quantitative, and mixed methods approaches (5th ed.). Thousand Oaks, CA: Sage.
Department of Defense. (2014, March 12). Risk management framework (RMF) for dod information technology (IT) (DOD Instruction 8510.01). Retrieved from https://www.esd.whs.mil/Portals/54/Documents/DD/issuances/dodi/851001p.pdf?ver=2019-02-26-101520-300
Hayden, L. (2010). IT security metrics: A practical framework for measuring security & protecting data. New York, NY: McGraw Hill.
Hubbard Decision Research. (2020). Applied Information Economics. Retrieved from https://hubbardresearch.com/about/applied-information-economics/
Hubbard, D. (2020). The failure of risk management: Why it’s broken and how to fix it (2nd ed.). Hoboken, NJ: John Wiley & Sons.
Hubbard, D., & Seiersen, R. (2016). How to measure anything in cybersecurity risk. Hoboken, NJ: John Wiley & Sons.
Kaušpadienė, L., Ramanauskaitė, S., & Čenys, A. (2019). Information security management framework suitability estimation for small and medium enterprise. Technological and Economic Development of Economy, 25(5), 979–997. doi:http://franklin.captechu.edu:2123/10.3846/tede.2019.10298
Lee, A. J. (2015). Predictive analytics: The new tool to combat fraud, waste and abuse. The Journal of Government Financial Management, 64(2), 12–16. Retrieved from https://franklin.captechu.edu:2074/docview/1711620017?accountid=44888
Lis, P., & Mendel, J. (2019). Cyberattacks on critical infrastructure: An economic perspective 1. Economics and Business Review, 5(2), 24–47. Retrieved from doi:http://franklin.captechu.edu:2123/10.18559/ebr.2019.2.2
National Institute of Standards and Technology. (2014; 2018). Risk management framework for information systems and organizations, revision 2 (NIST 800-37). Retrieved from https://nvlpubs.nist.gov/nistpubs/SpecialPublications/NIST.SP.800-37r2.pdf
Prusak, L. (2010, October 7). What can’t be measured. Harvard Business Review. Retrieved from https://hbr.org/2010/10/what-cant-be-measured
Russo, M. (2018). The Risk Reporting Matrix is a Threat to Advancing the Principle of Risk Management. Unpublished manuscript.
Saaty, T. L. (1980). The analytic hierarchy process: Planning, priority setting, resources allocation. New York, NY: McGraw.
Smith, J. (1988). Characterizing computer performance with a single number. University of Washington. Retrieved from http://courses.cs.washington.edu/courses/cse471/01au/p1202-smith-ps
Under Secretary of Defense for Acquisition, Technology, and Logistics. (2006, August). Risk management guide for DOD acquisition. Retrieved from https://www.acq.osd.mil/damir/documents/DAES_2006_RISK_GUIDE.pdf
University of Melbourne. (2018, May). Risk assessment methodology. Retrieved from https://safety.unimelb.edu.au/__data/assets/pdf_file/0007/1716712/health-and-safety-risk-assessment-methodology.pdf
Watkins, L. A., & Hurley, J. S. (2015). Cyber maturity as measured by scientific-based risk metrics. Journal of Information Warfare, 14(3), 57–65. Retrieved from https://www.researchgate.net/publication/283421461_Cyber_Maturity_as_Measured_by_Scientific-Based_Risk_Metrics
Watkins, L., & Hurley, J. S. (2016). The Next Generation of Scientific-Based Risk Metrics: Measuring Cyber Maturity. International Journal of Cyber Warfare and Terrorism (IJCWT), 6(3), 43–52. Retrieved from https://www.researchgate.net/publication/305416838_The_Next_Generation_of_Scientific-Based_Risk_Metrics_Measuring_Cyber_Maturity
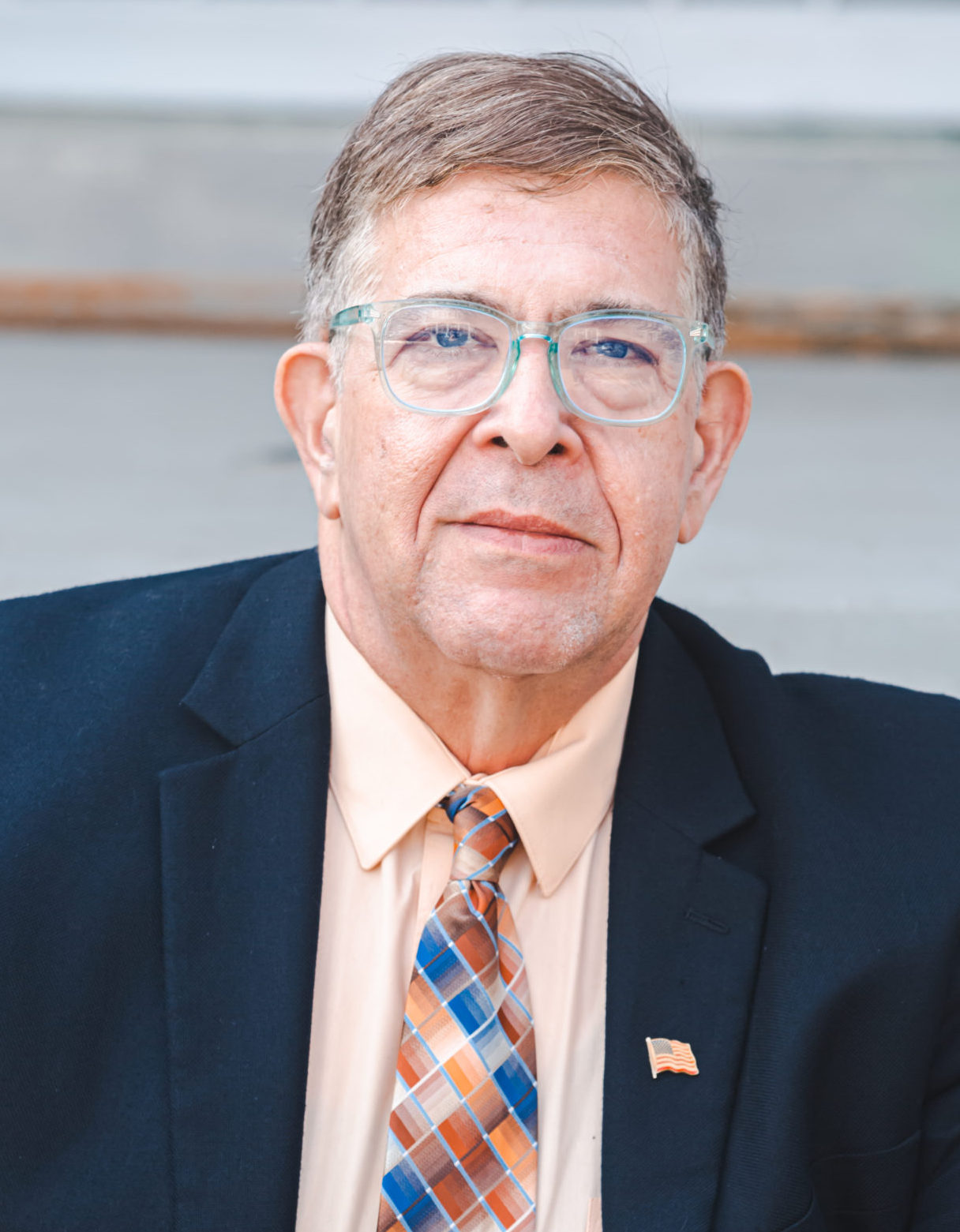
Dr. Russo is currently the Senior Data Scientist with Cybersenetinel AI in Washington, DC. He is a former Senior Information Security Engineer within the Department of Defense’s (DOD) F-35 Joint Strike Fighter program. He has an extensive background in cybersecurity and is an expert in the Risk Management Framework (RMF) and DOD Instruction 8510, which implement RMF throughout the DOD and the federal government. He holds a Certified Information Systems Security Professional (CISSP) certification and a CISSP in information security architecture (ISSAP). He has a 2017 Chief Information Security Officer (CISO) certification from the National Defense University, Washington, DC. Dr. Russo retired from the US Army Reserves in 2012 as a Senior Intelligence Officer.