SPOT: Why End-point Security is not good enough…
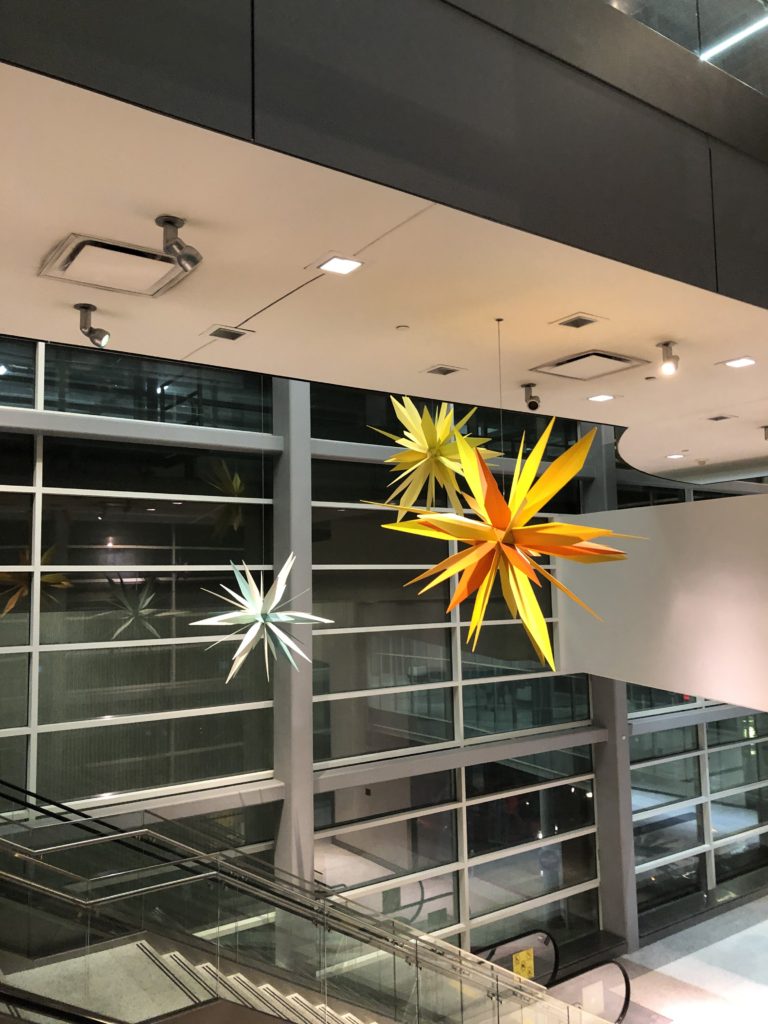
Threat Intelligence and Situational Awareness Beyond the Security Boundary
Columbus (2019a) identifies ten major cybersecurity firms that are applying AI-based solutions to enhance cybersecurity protections and highlights foundational concerns about private-sector cybersecurity intrusion system development and production. Columbus’ (2019a; 2019b) work supports the issue that these identified companies use end-point detection alone; this detection is based upon threat characteristics found within the businesses or agencies local IT architecture.
“There is a need to include external or heterogeneous data to supplement threat detection and prevention to protect the nation’s data better.”
End-points may include, for example, desktops, servers, and routers (Palo Alto, n.d.). An end-point processes both threat and non-threat information, details, and digital signatures for a determination of risk. This information is ultimately used to guide organizational leadership and cybersecurity staff members for follow-on actionable responses.
Companies rely heavily upon internal security, system, and antivirus logs, i.e., internal data, to identify risks and threats within their IT infrastructure (Ezeife et al., 2008; Zuech, Khoshgoftaar, & Wald, 2015). Explicitly, there is a need to include external or heterogeneous data to supplement threat detection and prevention to protect the nation’s data better (Hensel, 2016; Nagrecha & Chawla, 2016). External or heterogeneous data exists outside the resident IT environment; it is exterior to the local computer network. It can further enhance an organization’s situational awareness and ability to respond to threats (Galloppo & Previati, 2014; Hassani & Renaudin, 2018; Nagrecha & Chawla, 2016).
The addition of external data offers more effective protection by providing valuable intelligence into the IT environment beyond the confines of an organization’s localized network (Rodriguez & Da Cunha, 2018; Strand, Wangler, & Niklasson, 2004). This information may include, for example, government-sponsored threat indicator, honeypot, or honeynet data in machine-readable formats that are designed to capture the presence and actions of cyber-threats’ Tactics, Techniques, and Procedures (TTP) (Kumar & Verma, 2017; Ng, Pan, & Xiang, 2018; Spitzner, 2003; Zhan, Xu, M., & Xu, S., 2013).
Statistical analysis of Columbus’ (2019a) article identifies that at best, 30% of the noted cyber-defense solution providers apply heterogeneous data to their intrusion detection-based solution—none openly describe the use of any defined data science solution or specific data science models (Columbus, 2019a).
This ontological analysis of Columbus’ (2019a) work further confirms this finding; a majority of the reviewed commercial solutions are limited to internal network traffic and do not use or emphasize external threat or intelligence data in their solutions (Columbus, 2019a; Schroer, 2019).
Ontological Survey and Analysis: Top 10 Cybersecurity Companies to watch in 2019
# Company Y|N? Language of use Language of non-use
(External Data)
1 | Absolute | N | “delivers the intelligence needed to ensure security.” |
“self-healing
endpoint security, always-connected visibility into their devices, data,
users, and applications.” “uncompromised endpoint persistence” | |
2 | Blackberry AI and Predictive Security | N | “uses AI and machine learning to protect the entire attack surface of an enterprise with automated threat prevention.” | ||
3 | Centrify | N | “By implementing least privilege access.” | ||
4 | Cloudflare | Y?a | “protects websites from a range of online, threats including spam”b | ||
5 | CrowdStrike | N |
“Applying
machine learning to endpoint detection” “real-time analysis of data from endpoint events” | ||
6 | Hunters.AI | N | “autonomous system that connects to multiple channels within [emphasis added] an organization” | ||
7 | Idaptive | N | “secures access to applications and endpoints by verifying every user” | ||
8 | Kount | Y? | “adaptive platform learns of new threats and continuously updates risk scores” c | ||
9 | MobileIron | N | |||
10 | Sumo Logic | Y? | “continuous intelligence from…data across the entire application lifecycle and stack”d |
aY?: denotes the provided open-source information is unclear about the use of heterogeneous data as integral to the commercial solution.
b Cloudflare: The assumption is the use of the available information from web sources supporting the identification is moderately related to external data use.
c Kount: While it is not clear how Kount’s system learns about new threats, it does indicate a potential use of external threat intelligence and data analytic methods.
d Sumo Logic: It is assumed that its declared access to threat intelligence extends beyond the local IT environment.
Selected References
Columbus, L. (2019a, June 16). Top 10 cybersecurity companies to watch in 2019. Forbes. Retrieved from https://www.forbes.com/sites/louiscolumbus/2019/06/16/top-10-cybersecurity-companies-to-watch-in-2019/#4b683b696022
Columbus, L. (2019b, May 27). 25 machine learning startups to watch in 2019. Forbes. Retrieved from https://www.forbes.com/sites/louiscolumbus/2019/05/27/25-machine-learning-startups-to-watch-in-2019/#181be6483c0b
Columbus, L. (2019c, January 23). Data scientist leads 50 best jobs in America for 2019 according to Glassdoor. Forbes. Retrieved from https://www.forbes.com/sites/louiscolumbus/2019/01/23/data-scientist-leads-50-best-jobs-in-america-for-2019-according-to-glassdoor/#457226e77474
Ezeife, C. I., Dong, J., & Aggarwal, A. K. (2008). SensorWebIDS: A web mining intrusion detection system. International Journal of Web Information Systems, 4(1), 97–120. Retrieved from http://franklin.captechu.edu:2123/10.1108/17440080810865648
Galloppo, G., & Previati, D. (2014). A review of methods for combining internal and external data. The Journal of Operational Risk, 9(4), 83–103. Retrieved from https://franklin.captechu.edu:2074/docview/1648312043?accountid=44888
Nagrecha, S., & Chawla, N. V. (2016). Quantifying decision making for data science: From data acquisition to modeling. EPJ Data Science, 5(1), 1–16. Retrieved from doi:http://franklin.captechu.edu:2123/10.1140/epjds/s13688-016-0089-x
Ng, C., Pan, L., Xiang, Y. (2018). Honeypot frameworks and their applications: A new framework. Singapore: Springer.
Palo Alto. (n.d.). What is an endpoint [Blog post]? Palo Alto. Retrieved from https://www.paloaltonetworks.com/cyberpedia/what-is-an-endpoint
Rodriguez, L., & Da Cunha, C. (2018). Impacts of big data analytics and absorptive capacity on sustainable supply chain innovation: A conceptual framework. LogForum, 14(2), 151–161. Retrieved from doi:http://franklin.captechu.edu:2123/10.17270/J.LOG.267
Schroer, A. (2019, April 10). 25 Companies merging AI and cybersecurity to keep us safe and sound. Built-In. Retrieved from https://builtin.com/artificial-intelligence/artificial-intelligence-cybersecurity
Zhan, Z., Xu, M., & Xu, S. (2013). Characterizing honeypot-captured cyber attacks: Statistical framework and case study. IEEE Transactions on Information Forensics and Security, 8(11), pp.1775–1789. doi: 10.1109/TIFS.2013.2279800
Zuech, R., Khoshgoftaar, T. M., & Wald, R. (2015). Intrusion detection and big heterogeneous data: A survey. Journal of Big Data, 2(1), 1–41. Retrieved from http://franklin.captechu.edu:2123/10.1186/s40537-015-0013-4
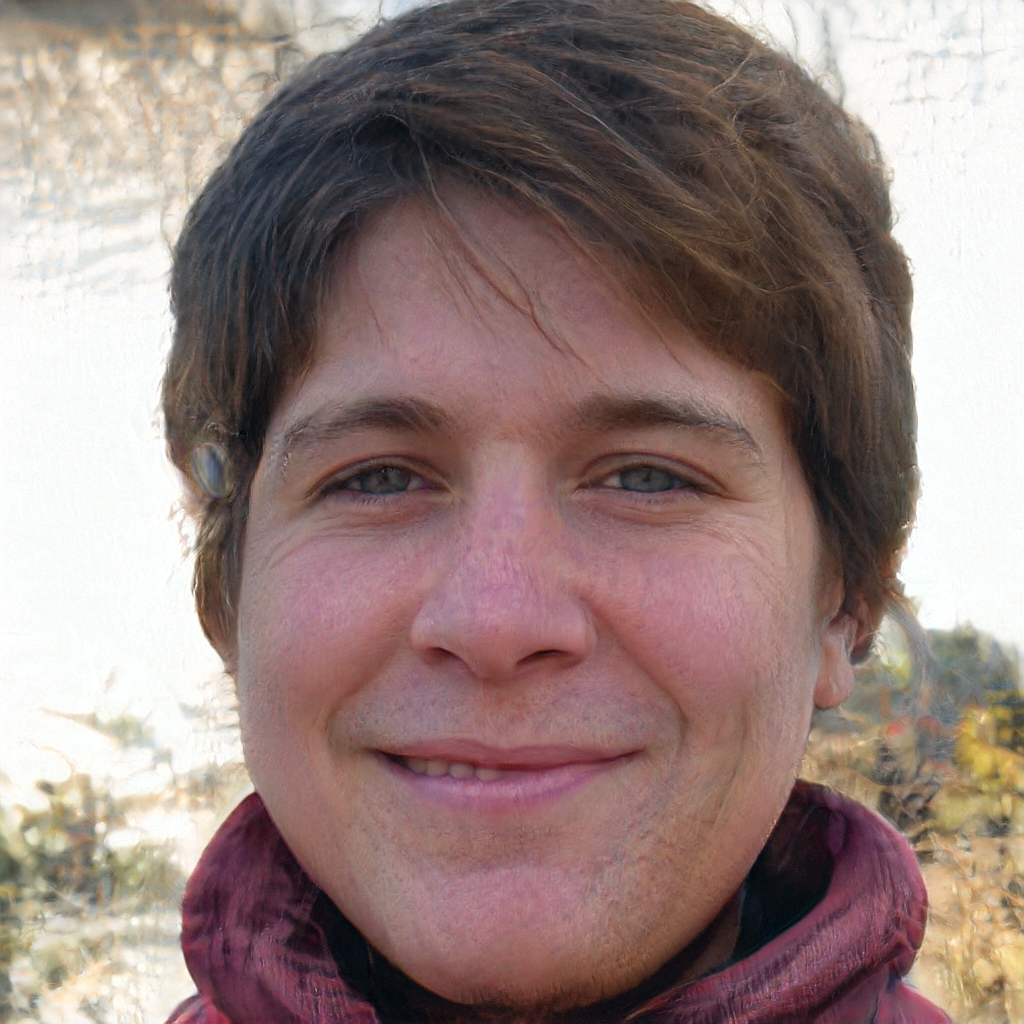
Ms. Columbus has worked in the Intelligence Community (IC) for over 20 years. She retired from the US Air Force in 2014 after working as a Senior Advisor providing authoritative advice on all aspects of Cyberspace operations, force structure and organizational concepts. She oversaw strategic support activities to enable the right mix of cyber capabilities for future operations.