The Illusion of Cybersecurity Quantification
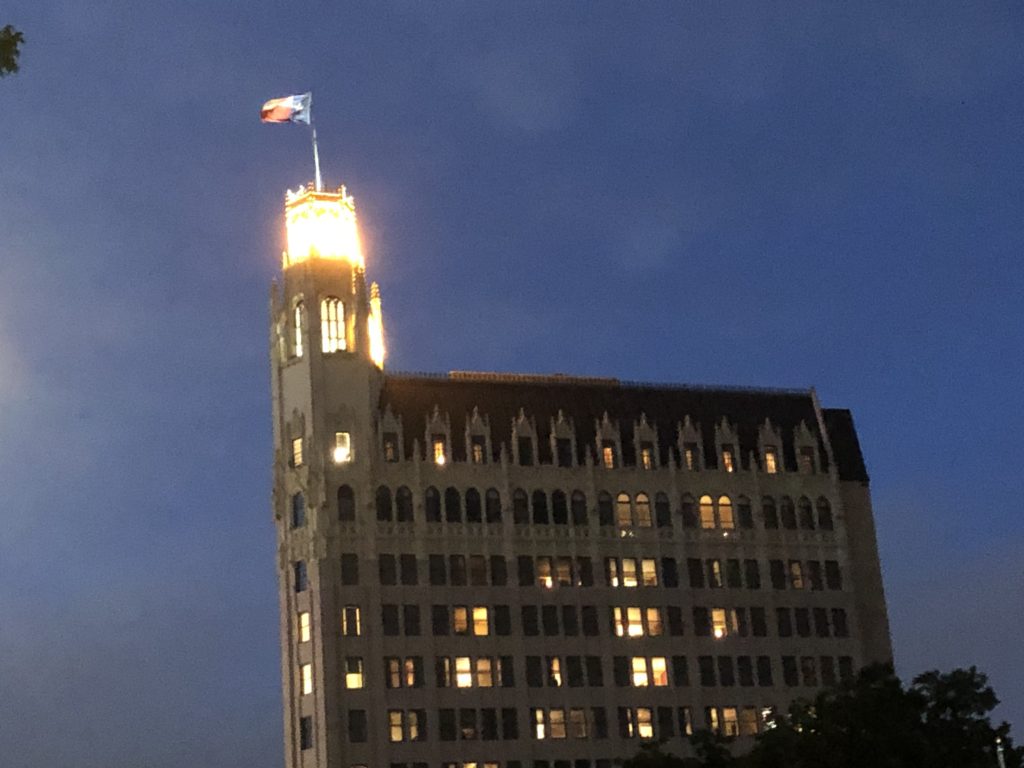
Where has measurability gone?
Approximately “48% [of organizations] attempt to quantify the damage a cyberattack could have” on their business (Lis & Mendell, 2019, p. 27). This estimate does not provide a complete picture of the damage beyond more than the cost of operational downtime. Other tangible and intangible costs of implementing available defensive measures such as those afforded by quantitative or even qualitative solutions are seldom considered (Lis & Mendell, 2019).
As Hubbard and Seiersen (2016) describe in their book, How to Measure Anything in Cybersecurity Risk, “the security readiness of [U.S.] federal systems and the nation’s overall security posture… taken a step back” (Waddell, 2015, as cited in Hubbard & Seiersen, 2016, p. 9). The cybersecurity community has, unfortunately, been engaged in quasi-quantitative measures to hide its lack of quantitative certainty (Hubbard & Seiersen, 2016).
Measurability is needed for senior corporate or agency management to understand and better resolve the challenges of cybersecurity attacks against their data and networks. A key example of existing quasi-quantitative assessment is the illusion of quantitative cybersecurity employing the purely qualitative (and long-accepted) Risk Matrix (RM) (Russo, 2018). The RM is a specific representation of one example by the cybersecurity community to consciously or unconsciously avoid a serious regimen to create valid quantification of the dangers and impacts of cyber-attacks (Hubbard & Seiersen, 2016).
The RM is a decision-support tool used to describe the level of risk most commonly in the area of project and program management, and more recently, in the field of cybersecurity. RMs are used to capture a qualitative graphical representation of the levels of risk that exist during the development of a system; “its representation is simplistic, but its implications are far-reaching” (Russo, 2018, p. 3). The RM is one of the long-present pitfalls demanding immediate attention and focus on the realistic quantification of cybersecurity dangers faced by organizations (Hubbard & Seiersen, 2016; Russo, 2018).
The RM requires users to apply subjective labels to a typical 5×5 matrix; Figure 2 provides an example of an RM where the likelihood or probability is on the vertical and consequence or impact are across the horizontal axes (University of Melbourne, 2018). Users “[d]evelop probability and consequence scales by allocating thresholds across the WBS [Work Breakdown Structure],” but there are no substantive back-end methodologies or mathematics to derive any quantified risk value scores (Under Secretary of Defense for Acquisition, Technology, and Logistics [USD-ATL], 2006, p. 14).

Risk matrix (University of Melbourne, 2018)
Hayden (2010) and Hubbard and Seiersen’s (2016) main issue with the RM is that it has proven to be an overwhelming disappointment to the field of risk management. “They [RMs] are a failure…. They do not work” (Hubbard & Seiersen, 2016, p. 14). Both organizational cybersecurity and agency leaders need to know the actual state of its cybersecurity posture and not just relative and independent measures (or guesses) by its IT staff alone.
The RM was never designed to be a quantitative risk measure (Project Management Skills, 2010); however, it has been used to imply the quantification of qualitative expressions such as likely or minor risks to the network or its data (Hubbard & Seiersen, 2016). Practitioners of risk management and the recipients of RM reporting have assumed it is founded upon reliable analyses and statistics; they have unfortunately been deceived by this quasi-measure of certainty (Hubbard & Seiersen, 2016). In Hubbard’s (2009b) seminal publication, The Failure of Risk Management, he states that the RM is part of “various scoring schemes instead of quantitative risk methods…. all of them [to include RMs], without exception, are borderline or worthless” (p. 122). The significance of this study is to define a path to inject quantified analyses and outputs that can be applied by the cyber-defenders as well as the organizational leaders resourcing their efforts.
The significance of measurability. Hayden (2010) states that “established metrics programs…still, struggle with understanding their security efforts” (p. 5). Any problem needs to be measured (Prusak, 2010). Companies create cybersecurity programs, but they have been nothing more than data collection efforts without any attempt to identify the real measured damages and costs of applying or not applying a defined solution (Hayden, 2010). Measurability affords the effective use of data and has demonstrated operational and security efficiencies for both the public and private sectors (Lee, 2015)
The worth of an actual value of improvement for example data science solutions affords a company’s or agency’s leadership a potential ability to monetize the impacts of cybersecurity intrusions and damages better; this has been a research objective from the cybersecurity community for the past 20 years (Jahan, 2017; Jasim, 2018; Radziwill & Benton, 2017).
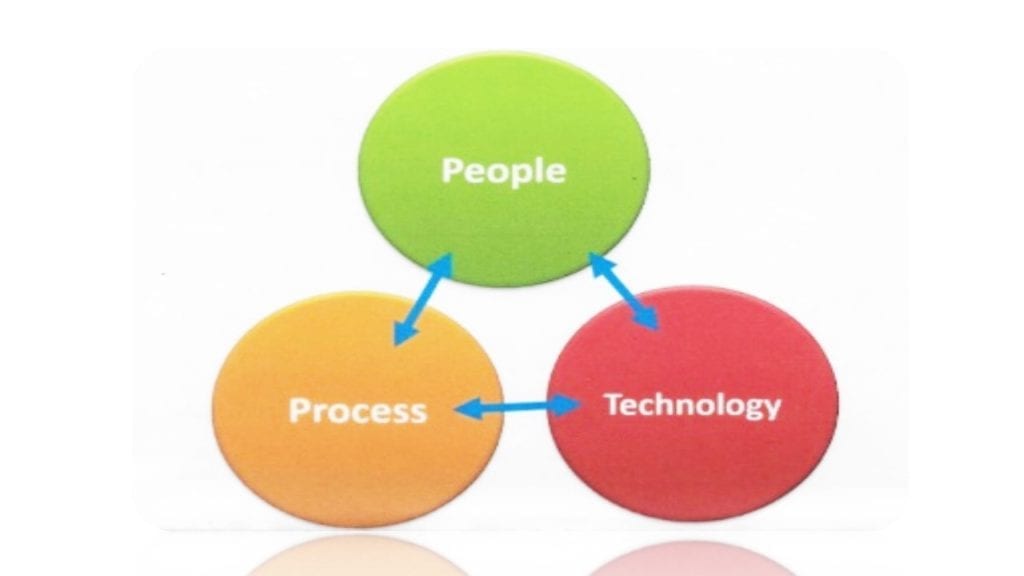
As Peter Drucker, the acclaimed author on the topic of modern business, stated, “what gets measured gets managed” (Prusak, 2010, para. 5). If there are defined measures of success and failure, it benefits an organization’s viability to remain secure, productive as well as profitable.
Selected References
Hubbard, D. (2009a, February 11). I am concerned about the CI, median and normal distribution [Blog post]. Hubbard Decision Research. Retrieved from https://hubbardresearch.com/i-am-concerned-about-the-ci-median-and-normal-distribution/
Hubbard, D. (2009b). The failure of risk management: Why it’s broken and how to fix it. Hoboken, NJ: John Wiley & Sons.
Hubbard, D., & Seiersen, R. (2016). How to measure anything in cybersecurity risk. Hoboken, NJ: John Wiley & Sons. Jahan, A., & Alam, M. A. (2017). Intrusion detection systems based on artificial intelligence. International Journal of Advanced Research in Computer Science, 8(5).
Lee, A. J. (2015). Predictive analytics: The new tool to combat fraud, waste and abuse. The Journal of Government Financial Management, 64(2), 12–16. Retrieved from https://franklin.captechu.edu:2074/docview/1711620017?accountid=44888
Lis, P., & Mendel, J. (2019). Cyberattacks on critical infrastructure: An economic perspective 1. Economics and Business Review, 5(2), 24–47. Retrieved from doi:http://franklin.captechu.edu:2123/10.18559/ebr.2019.2.2
Prusak, L. (2010, October 7). What can’t be measured. Harvard Business Review. Retrieved from https://hbr.org/2010/10/what-cant-be-measured
Radziwill, N. M., & Benton, M. C. (2017). Cybersecurity cost of quality: Managing the costs of cybersecurity risk management. ArXiv. Retrieved from https://arxiv.org/ftp/arxiv/papers/1707/1707.02653.pdf
Russo, M. (2019). Critiques paper: Cybersecurity and data science join forces. Unpublished manuscript.
Russo, M. (2018). The Risk Reporting Matrix is a Threat to Advancing the Principle of Risk Management. Unpublished manuscript.
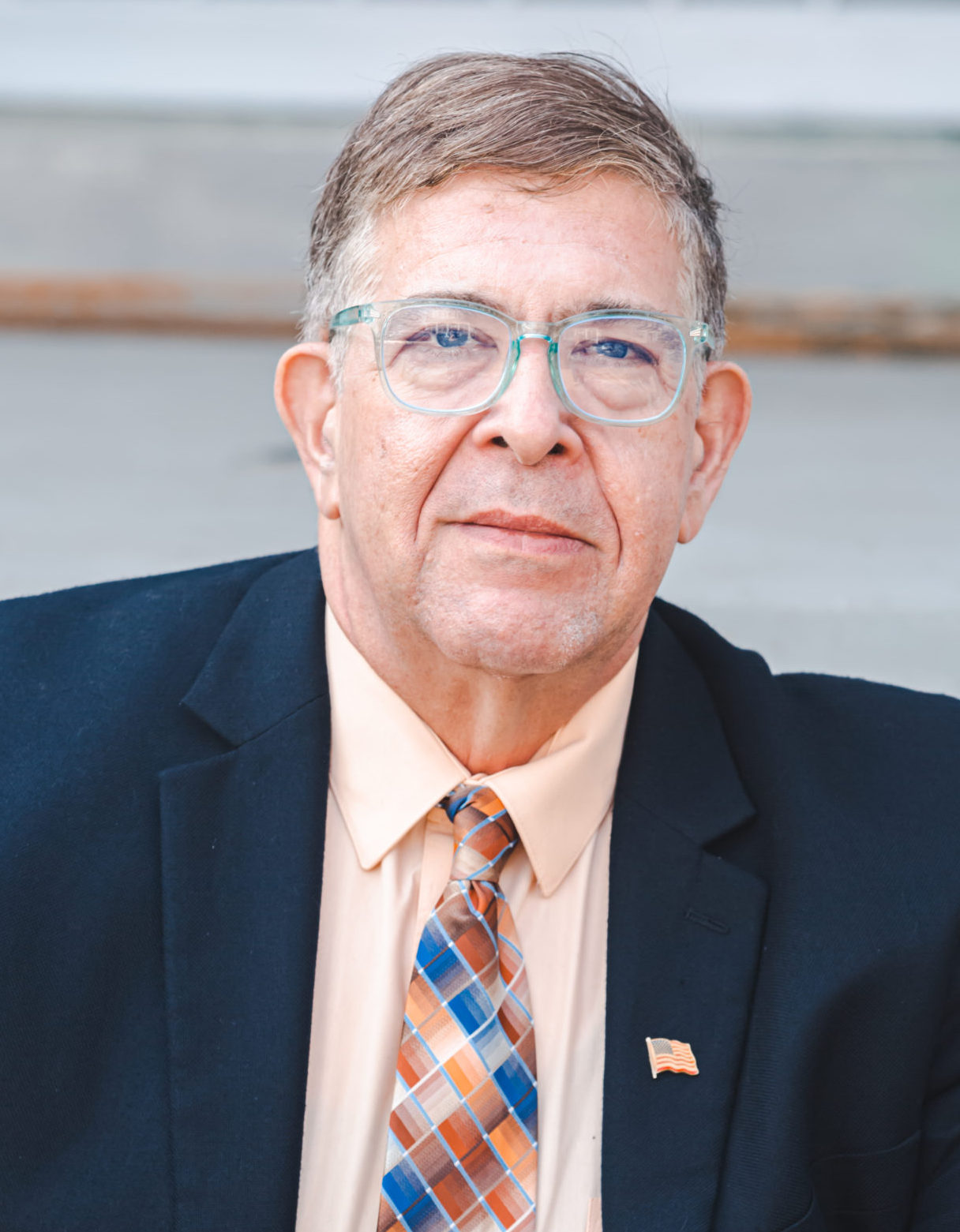
Dr. Russo is currently the Senior Data Scientist with Cybersenetinel AI in Washington, DC. He is a former Senior Information Security Engineer within the Department of Defense’s (DOD) F-35 Joint Strike Fighter program. He has an extensive background in cybersecurity and is an expert in the Risk Management Framework (RMF) and DOD Instruction 8510, which implement RMF throughout the DOD and the federal government. He holds a Certified Information Systems Security Professional (CISSP) certification and a CISSP in information security architecture (ISSAP). He has a 2017 Chief Information Security Officer (CISO) certification from the National Defense University, Washington, DC. Dr. Russo retired from the US Army Reserves in 2012 as a Senior Intelligence Officer.