Data Science Study Challenges
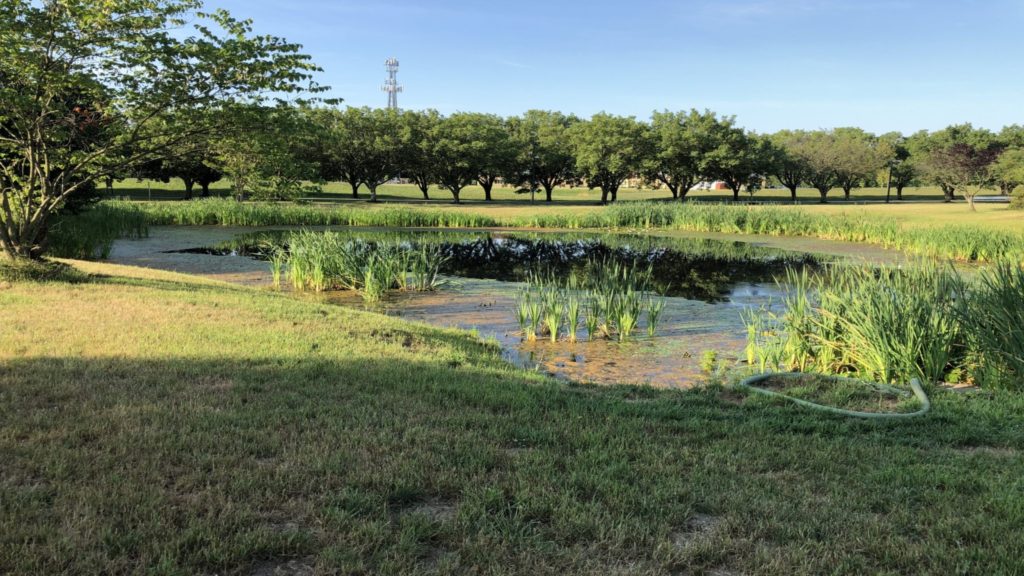
The Problems in Both Qualitative and Quantitative Research
The Real Data Science Challenges: Data Collection and Analysis
Data collection in both qualitative and quantitative research have their challenges. Annink (2016) suggests that within the qualitative, there have been efforts to “suppress” and to create “voids [that] affect the findings” (Annink, 2016, p. 1). Furthermore, in a study of approximately 600 Army enlisted personnel, researchers were concerned about the “complicated” and “sheer volume” of data within their military sample population (Young, Houston, Harris, Hoffman, & Wise, 1990, p. 301). However, data collection remains foundational to academic research, and even with their various problems, are critical to any study (Creswell & Creswell, 2018). There are vital steps required for both front-end data collection, and the back-end data analysis efforts, whether conducting a quantitative or qualitative undertaking.
Quantitative data collection can take the form of a survey design to gather information (Creswell & Creswell, 2018). The survey’s first step is to identify the purpose of the data collection in support of the study’s problem statement. Second, the researcher needs to inform the reader of their rationale for using a survey. Third, there is a need to indicate whether the study will require a single point in time dataset (cross-sectional study) or data collected over time (longitudinal study). Finally, the researcher identifies how the data collection survey will be administered. The survey may take the form of a written questionnaire, email questions, or online web-based service to collect quantifiable information (Creswell & Creswell, 2018).
The stages for qualitative data collection offer additional challenges. Creswell and Creswell (2018) state that this effort is about setting the “stage for discussion of issues” (Creswell & Creswell, 2018, p. 185). Additionally, Kivikko et al. (2007) discuss a resultant problem that occurs when the survey participants are aware “of the [study’s] purpose” (Kivikko et al., 2007, p. 321). Knowledge of a study’s purpose affects respondents to provide more general versus specific answers to questions sought (Kivikko et al., 2007). This issue has a more significant concern to the qualitative researcher and provides essential study preparation information. However, a defined set of collection steps are still necessary for useful data collection.
The stages for qualitative data collection begin with the identification of the respondents or materials required (Creswell & Creswell, 2018). Next, there is a requirement for “purposefully” selecting the needed information to shape the research approach (Creswell & Creswell, 2018, p. 185). The investigator needs to identify the methods for gathering a sound cross-section of volunteers, publications, or materials. Finally, the researcher identifies the data types or observations that provide a qualitative perspective. A common hurdle remains for the qualitative researcher in this process: the initial needed to identify and collect relevant information for beginning a successful study (Creswell & Creswell, 2018).
The Differences of Two Data Analyses
Data analysis occurs on the back-end of the study. Cooper (2018) identifies that problems may arise because of missing, incomplete, or questionable data that contribute to adverse effects for the final analysis (Cooper, 2018). Poor quality information is problematic for the quantitative approach. Nevertheless, for example, automated tools such as R and Python for data extraction needs are especially helpful to resolve many of the problems from inadequate or incomplete data (Cooper, 2018).
The quantitative follows a general three-step process, but not all steps are required. Typically, the beginning phase will start with a descriptive analysis of the dependent and independent variables. This analysis uses the information of the variables to identify past data issues (Lee, 2015). Lee (2015) states that the descriptive is part of a “learning process [] initiated by incorporating past errors in the evaluation of incoming or new data and changes” (Lee, 2015, p. 16). The next step is prescriptive analysis, and it provides suggested directions or likely courses of action for the investigator (Lee, 2015). The final phase is characteristically the use of predictive analysis. It concentrates on determining future outcomes produced by the study; without understanding the “past, applied to the present, the future desire of actionable results would be meaningless and useless” (Russo, 2018, p. 7). While all steps are not required, the analytic objective should attempt to determine future occurrences using available mathematical and statistical models.
Like the quantitative, qualitative data analysis also requires a continual need to ensure accuracy of the information gathered and processed (Creswell & Creswell, 2018). Figure 9.2, Data Analysis in Qualitative Research, begins with the “textual or imagery data,” organizing it for analysis, reviewing the data, coding or applying automated tools to generate themes and descriptions, “interrelating themes/descriptions” based upon the study design, and providing a finished written interpretation of findings, i.e., final study (Creswell & Creswell, 2018, p. 194).
Conclusion
There are many issues faced by the researcher in conducting either a qualitative or quantitative study. They both rely upon a need to collect and thoroughly process critical data. The interpretation of the data through analysis supports a thorough and final academic report of findings. The challenge is not just the problem of collecting the data but also the ability to interpret the information to identify a path forward for future academic studies and efforts (Creswell & Creswell, 2018).
References
Annink, A. (2017). Using the research journal during qualitative data collection in a cross-cultural context. Entrepreneurship Research Journal, 7(1), 1–17. Retrieved from doi:http://franklin.captechu.edu:2123/10.1515/erj-2015-0063
Cooper, H. (2018). Reporting quantitative research in psychology: How to meet APA style journal article reporting standards (2nd ed.). Washington, DC: American Psychological Association.
Creswell, J. W., & Creswell, J. D. (2018). Research design: Qualitative, quantitative, and mixed methods approaches (5th ed.). Thousand Oaks, CA: Sage.
Kivikko, K., Mäkinen, A., Järventausta, P., Silvast, A., Heine, P., & Lehtonen, M. (2008). Comparison of reliability worth analysis methods: Data analysis and elimination methods. IET Proceedings. Generation, Transmission, and Distribution, 2(3), 321–329. Retrieved from https://franklin.captechu.edu:2074/docview/1627082453?accountid=44888
Lee, A. J. (2015). Predictive analytics: The new tool to combat fraud, waste and abuse. The Journal of Government Financial Management, 64(2), 12–16. Retrieved from https://search.proquest.com/docview/1711620017?accountid=44888
Russo, M. (2018). Disciplines paper: Predictive analytics: The potential role of process improvement. Unpublished manuscript.
Young, W. Y., Houston, J. S., Harris, J. H., Hoffman, R. G., & Wise, L. L. (1990). Large-scale predictor validation in project A: Data collection procedures and data base preparation. Personnel Psychology, 43(2), 301–311. Retrieved from https://franklin.captechu.edu:2074/docview/220131995?accountid=44888
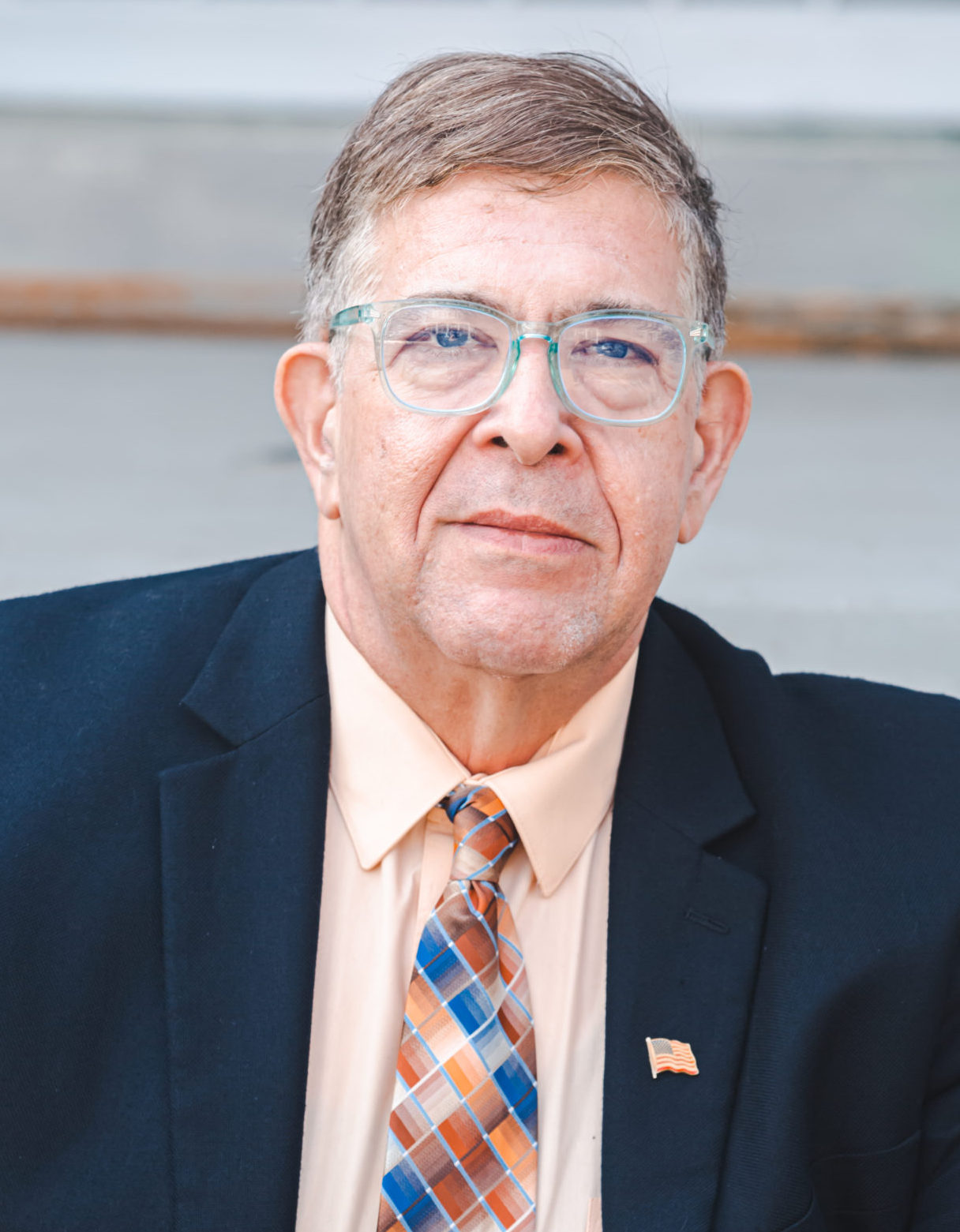
Dr. Russo is currently the Senior Data Scientist with Cybersenetinel AI in Washington, DC. He is a former Senior Information Security Engineer within the Department of Defense’s (DOD) F-35 Joint Strike Fighter program. He has an extensive background in cybersecurity and is an expert in the Risk Management Framework (RMF) and DOD Instruction 8510, which implement RMF throughout the DOD and the federal government. He holds a Certified Information Systems Security Professional (CISSP) certification and a CISSP in information security architecture (ISSAP). He has a 2017 Chief Information Security Officer (CISO) certification from the National Defense University, Washington, DC. Dr. Russo retired from the US Army Reserves in 2012 as a Senior Intelligence Officer.