What’s Next for Better Cyber Predictive Analytics?

How can Predictive Analytics (PA) move forward to improve our ability to better anticipate problems and threats?
The use of data has led to increases in efficiency and effectiveness for both the public and private sectors (Lee, 2015). Cybersecurity Predictive Analytics (CPA) is about the technology, mathematics, and models to make better forecasts. It is further about how to better predict human behavior that will help companies make improved and profitable decisions (Siegel, 2013). The challenge is how to make advances in CPA to provide overall improved predictions.
The arithmetic has been worked for centuries within the understanding and improvements of mathematics and statistics to aid man’s ability to use the available data. With the vast expansion of the data, we can now infer and better predict outcomes due to the Big Data “revolution.” Further, this has supported increased improvement in the models and algorithms that have given us an ability to measure the innate uncertainty and variability of humans. This is the “technology” component that has played a greater part in man’s development. This has become even more impactful since the beginning of the Industrial Revolution.
Even further back in time, man has been enamored with the desire to foretell the future with oracles, wise men, and spiritual leaders that had a supposed connection with the divine. However, it was not until the early 20th Century that the “people” component was recognized as a contributor to improving the secular and rational improvements in CPA. In 1906, Sir Francis Galton conducted an experiment where he had several hundred individuals attempt to determine the final weight of a slaughtered cow. The result was greater accuracy from the collective crowd. With remarkable precision, the average weight guessed by the participants was 1,197 pounds, and in fact, the actual weight was 1,198 pounds (Tetlock & Gardner, 2016). It is this type of “crowd sourcing” effect that demonstrates the informed and knowledgeable average of all guesses (or, predictions) culminated into a final and near accurate answer—they were only one pound off.
The only factor that has yet to be fully introduced is the component of improved process. The solution to a major evolution in CPA may have always been hiding “in plain sight.” While process is presumed part of the two prior components, the final part may be found in the process that binds these two together. The classic People-Process-Technology (PPT) Triad may always have been in front of us, but we may have just missed this next dimension to improving the ability to make better forecasts. Sometimes the simplest answer may in fact be the correct answer or at least the next best path to pursue.
The People-Process-Technology Triad
The PPT Triad has a less than defined origin (Bravo, 2013); however, it has proven valuable in creating holistic solutions to other modern-day problems. In cybersecurity, for example, it is used as a methodology to solve incomplete security controls. It provides a means to address controls where a technical solution may not eliminate or mitigate a finding, for example, such as a lack of two-factor authentication. Leadership can leverage better training for its employees about password protection (people) and company policies that provide for stern punishment if an employee does not change their password regularly (process).
This post focuses on the process improvements between the technology and people components as part of the overall PPT Triad. While Halladay (2013) ignores process improvement, Lee (2015) emphasizes a “systematic approach needs to be taken for projects [and solutions]…” (p. 13) that implicitly calls for process as a means to solving project development where Halladay does not. Improvements in process may be beneficial in refining the state of CPA. The answer for improved CPA may be found simply in better policies, guidance, and best practices that have yet to be the focus of the data analytics community.
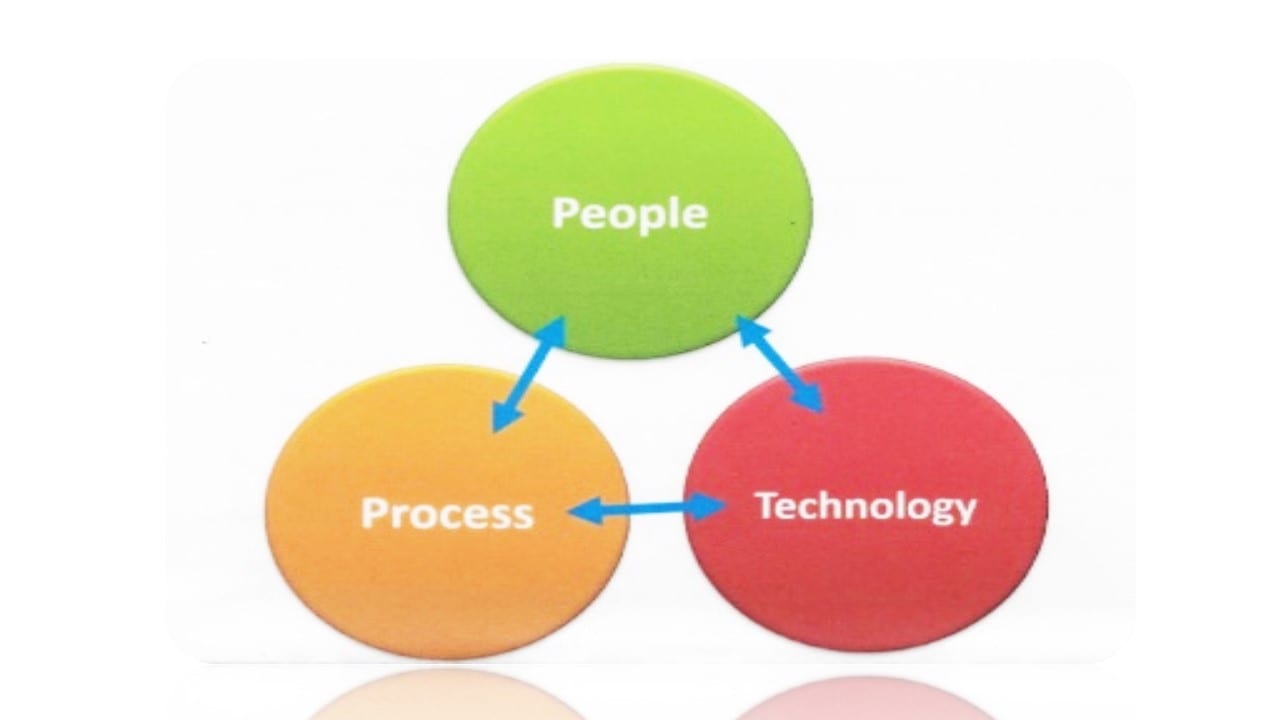
Brief History
The improvements in our mathematics’ can be seen in the current growth and demand for Artificial Intelligence (AI), data science, and data analytics as an outcome of the Big Data collection, analysis, and reporting revolution. However, all of this has not just been a technological occurrence, but a change in how these fields perceive and better accept the human element in improving their ability to predict the future. It was not until the work by Hubbard (Hubbard & Seiersen, 2016) in training SMEs to become “calibrated” experts which was one of the most recent improvements in predictive forecasting.
Hubbard’s work in this area is significant. While he recognizes that most individuals, including SMEs are initially bad at determining probabilities to a future event, they can be trained to predict better within the confines of the probability and their own unique expertise (Hubbard & Seiersen, 2016). It is reminiscent of the work of Sir Galton’s earlier described efforts, and it is taken to the next level. Hubbard (2016) and his team take SMEs through a calibration exercise that refines both the individual’s probabilistic predictive skills and refines it through the introduction of a 90% confidence interval. (Hubbard & Seiersen, 2016). As the exercise progresses more information is provided, and the overall quality of the forecast improves.
Recommended similar articles:
These progressive forecasts are monitored and measured by Hubbard’s team even after the initial one to two-day exercises. The SMEs are found to improve based upon these scientifically and psychologically developed calibration exercises. As pointed out by Hubbard, for example, common elements that interfere with CPA are reduced to include, underconfidence, overconfidence, and especially biases of the SME estimators that would have an otherwise adverse effect on such predictions (Hubbard & Seiersen, 2016).
The Three Branches
There are three distinct branches defined in the field of business analytics. They are descriptive, predictive, and prescriptive; this is where predictive analysis exists. This post focuses on the predictive but recognizes the vital importance of the others. Descriptive addresses the past, and the data of a like or similar occurrence that can contribute to the prediction portion of CPA. The predictive focuses on the “what if,” or the unknowns to be answered by either the statistical mathematics, the forecasts of the SME, or both (Praseeda & Shivakumar, 2014). The prescriptive helps in determining the follow-on actions to solve or reduce the negative impacts of a problem or issue. None are any better than the other and are just different (Praseeda & Shivakumar, 2014).Without the prior or the latter, this process flow would not provide any real or actionable insights to the business or organization.
CPA relies upon the “priors” of the descriptive data collected by the data scientist. Any advanced “…learning process is initiated by incorporating past errors in the evaluation of incoming or new data and changes…” (Lee, 2015, p. 16). The prescriptive is the result or suggested direction that occurs due to the outputs of CPA. Without the past, applied to the present, the future desire of actionable results would be meaningless and useless.
It Must Be Actionable
CPA is about creating actionable information that the business or individual can benefit (Halladay, 2013; Praseeda & Shivakumar, 2014). Halladay describes that business intelligence relies upon CPA to provide a holistic view to the company for it to make better decisions. A process improvement must move the state of CPA to a next and better point that enhances current decision-making demands of businesses or organizations (Halladay, 2013).
Halladay’s (2013) research focused on equipment leasing and the finance industry. Specifically, the objective of CPA is to support corporate leadership to make better future commercial decisions about risk and profitability. His work only calls out the technology and people. However, it does highlight the lack of explicit research in process improvement to enhance the PPT Triad, and suggests a new area requiring more academic and applied research.
In Plain Sight
The answer to improving the ability to make better predictions has been within our grasp, but we have failed to recognize it because it may have just appeared too obvious. But, why? The PPT Triad suggests that a solution to a problem may be found with the application of a technological, people, or process solution either used individually or in combination. It can be suggested that process and procedure are already an endemic part of the statistical mathematics used today in CPA. It would include, for example, the processes such as the transitive, substitution, and reflexive properties of mathematics that are already procedures embedded in the very nature of the applied mathematics and statistics. This may be why we have not explored that path. Maybe Occam’s razor is ideal; the simplest answer may be the most correct. Process improvement may help to refine the predictive ability of data scientists, statisticians, and corporate leadership and we just did not notice it as a next step.
But even if this lost variable holds the solution it is important to be mindful of the dangers of the absolute. We can never be categorically 100% able to predict a highly variant and fluid future. Before exploring potential improvements in process, there is a need to understand “process outcome paradoxes” (Tetlock & Gardner, 2016). Tetlock and Gardner (2016) describes in their book, Superforecasting, that “[n]othing is one hundred percent” (p. 134). Both the current state of technology and people components of the PPT Triad already recognize this through the measurement of probability and the inclusion of uncertainty (Hubbard & Seiersen, 2016). We can get better, but we will never reach “God-like” predictive capabilities no matter how good the three components of the Triad are improved upon or progress.
Potential Process Improvements
A suggested initial qualitative improvement should begin with the leadership’s understanding, execution, and accountability for implementing an effective CPA effort. One year after the 2015 Office of Personnel Management (OPM) data breach, the then-Acting Director Beth Cobert stated that: “[t]here’s a whole series of things around technology, around people, and process that are different today than a year ago” (Naylor, 2016). While Ms. Cobert recognized the importance of the PPT Triad to address this major data breach, the information already existed that should have stopped or at least vastly reduced the effectiveness of this major historical cybersecurity breach. This included the best practice implementation of two-factor authentication which was an already well-known best practice by the government. Did it really require a major exfiltration of millions of personnel data records to cause this?
The first process improvement should begin with accountability by leaders to provide the needed resources. Halladay (2013) describes that corporate leadership must both include CPA into the decision-making process and further accept those outputs without “second guessing or ignoring the predictive analytics” (p. 5). Before the process can improve, the strategic understanding and employment should be ingrained as part of the business or organizational culture by leadership.
Secondarily, Bayesian mathematics relies on the “priors.” Past information is applied especially in Bayesian mathematics to help the technology and the people component improve the quality of the prediction. This is not an unrealistic best practice and is based upon logic. As newer and verified information is provided to the predictive model it will improve the quality and fidelity of the output.
The same can be described for the SMEs. They retain a large body of knowledge as experts as they learn and grow within their respective area of study. SMEs can and will refine their predictions. As Lee discusses that CPA is an “iterative process” that combines sampling, estimation, and predictiveness into a single cohesive model (Lee, 2015). His conceptual model for a Predictive Analytics System (PAS) affords the best example of creating a refined process that can ensure repeatability of CPA efforts (p. 13).

Predictive analytics system. Reprinted from The Journal of Government Financial Management, “Predictive analytics: The new tool to combat fraud, waste and abuse” by A.J. Lee, p. 13.
Another key concern of any process improvement is prediction perishability. If a SME with any associated models predicts, for example, “the Democrat will win,” the value of the prediction diminishes as the day of the election approaches. To improve any measure of actionability, it is suggested that threshold dates, and in some cases times, should also be added to any prediction. Much like the addition of probability and uncertainty, a new factor of time- perishability should be added to improve the prediction and provide a means to better benchmark the SME and any predictive models; this process improvement would add a much-needed dimension to help company’s make better qualitative and quantitative decisions.
The final recommended process improvement may appear basic for a data scientist, but as noted in the 2015 OPM breach while it was already recognized that two-factor authentication was important, OPM (and much of the US government) had not implemented it. The suggested removal of predictions outside the norm, not their elimination from consideration, should occur. The common term is “outlier.” Any prediction, either mathematical or SME-based that appears outside of the general estimate should be set aside because such estimates would most likely skew the overall prediction.
However, we need to be reminded that outlier estimates should be removed to improve the process, but it must also be recognized that these outliers sometimes come true. Examples such as the 1941 surprise attack on Pearl Harbor or the September 11, 2001 attack would have been an extreme outlier or “black swan” event (Taleb, 2007). While not an absolute, it is suggested for the purposes of process outcome and prediction, and not a total answer to the challenges of better predictions.
Conclusion
CPA will most likely improve if the processes between the technology and the people are better melded. While both two separate pillars of the PPT Triad have afforded great improvements in the predictive capabilities of humans, maybe the next step is with the internal and external processes that can provide that next great revolutionary advance. It may seem too obvious an answer, but the works of Hubbard and Seiersen (2016) and Tetlock and Gardner (2016) recognize the connections needed between the two earlier PPT components. The next phase of improvements in the process are the most likely evolutionary step needed to improve the value of PA for better decision-making; this would likely be both of a quantitative nature, improving the mathematics, and the qualitative nature, improving upon the SME. The SME, cybersecurity expert, data scientist, or corporate officer who can only get near to, but never reach a “perfect” prediction.
References
(Recommended references link to Amazon)
Bravo, M. A. (2013, September 8). Who created the People – Process – Technology framework? Retrieved from Quora: https://www.quora.com/Who-created-the-People-Process-Technology-framework
Halladay, S. D. (2013). Using predictive analytics to improve decisionmaking. The Journal of Equipment Lease Financing (Online), 31(2), B1-B6. Retrieved from https://search.proquest.com/docview/1413251757?accountid=44888
Hubbard, D., & Seiersen, R. (2016). How to measure anything in cybersecurity risk. Hoboken, NJ: John wiley & sons.
Lee, A. J. (2015). Predictive analytics: The new tool to combat fraud, waste and abuse. The Journal of Government Financial Management, 64(2), 12-16. Retrieved from https://search.proquest.com/docview/1711620017?accountid=44888
Naylor, B. (2016, June 6). One year after OPM data breach, what has the government learned? Retrieved from National Public Radio: https://www.npr.org/sections/alltechconsidered/2016/06/06/480968999/one-year-after-opm-data-breach-what-has-the-government-learned
Praseeda, C., & Shivakumar, B. (2014). A review of trends and technologies in business analytics. International Journal of Advanced Research in Computer Science, 5(8). Retrieved from https://search.proquest.com/docview/1658426584?accountid=44888
Siegel, E. (2013). Predictive analytics: The power to predict who will click, buy, lie, or die. Hoboken: Wiley.
Silver, N. (2012). The signal and the noise: Why so many predictions fail–but some don’t. New York: Penguin.
Taleb, N. N. (2007). The black swan: The impact of the highly improbable (Vol. 2). New York: Random house.
Tetlock, P., & Gardner, D. (2016). Superforecasting: The art and science of prediction. New York: Random house.
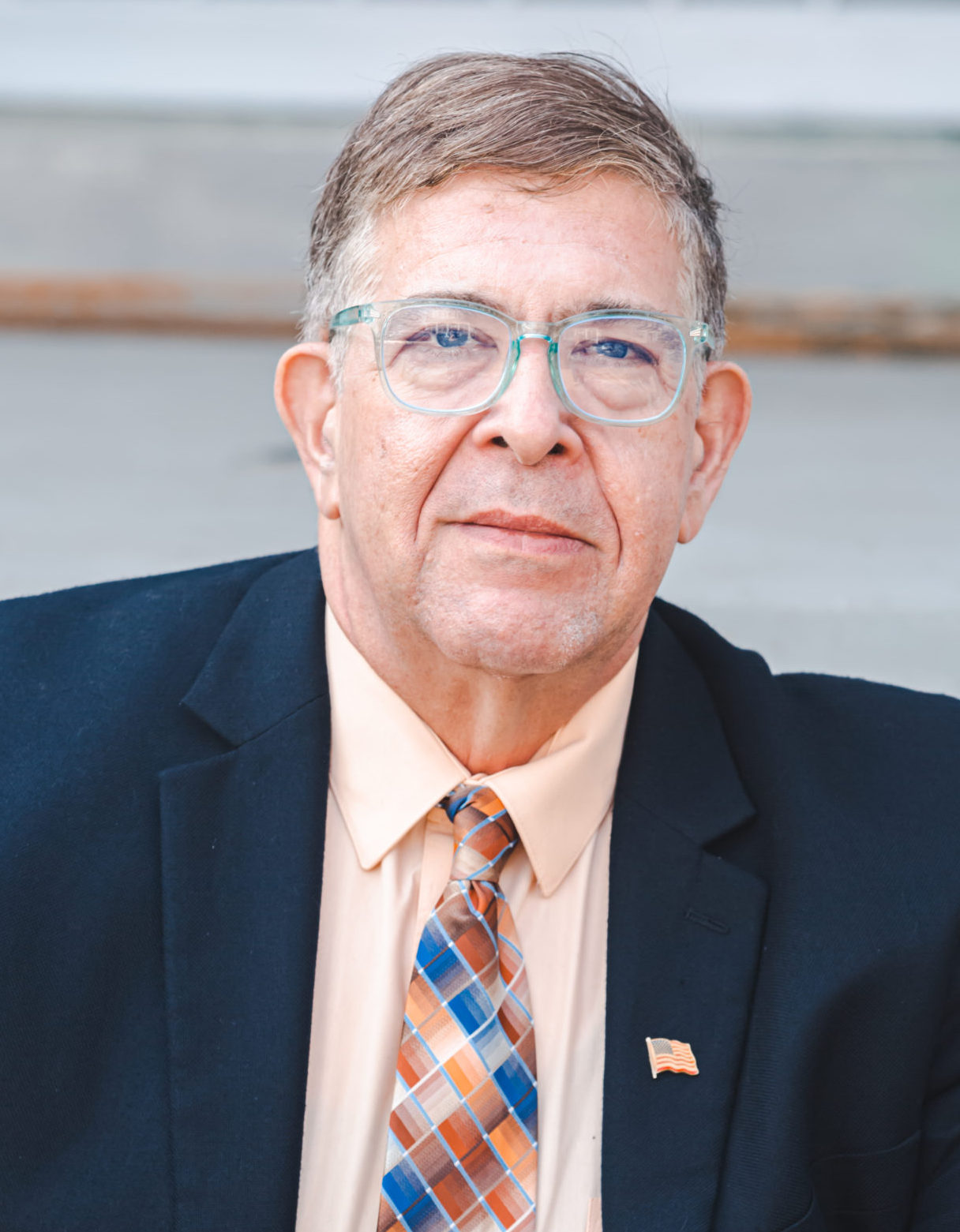
Dr. Russo is currently the Senior Data Scientist with Cybersenetinel AI in Washington, DC. He is a former Senior Information Security Engineer within the Department of Defense’s (DOD) F-35 Joint Strike Fighter program. He has an extensive background in cybersecurity and is an expert in the Risk Management Framework (RMF) and DOD Instruction 8510, which implement RMF throughout the DOD and the federal government. He holds a Certified Information Systems Security Professional (CISSP) certification and a CISSP in information security architecture (ISSAP). He has a 2017 Chief Information Security Officer (CISO) certification from the National Defense University, Washington, DC. Dr. Russo retired from the US Army Reserves in 2012 as a Senior Intelligence Officer.
1 thought on “What’s Next for Better Cyber Predictive Analytics?”
Comments are closed.