ESSAY: Can the Human “Poet” Bring Value to Predictive Analysis
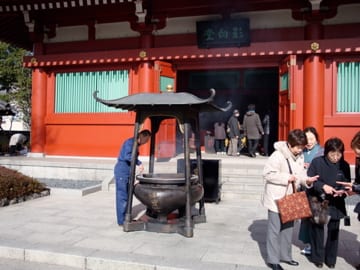
An essay on the role of how HUMAN beings play a vital role in the future of data analytics
From the time we are children through adulthood we are reminded that that which cannot be measured cannot be properly managed. The United States Congress, for example, repeatedly calls for better measurements and metrics, however, they do not appear to subsequently predict or at least foresee better the next financial crisis, political coup or cybersecurity attack with these numbers alone. The predictive forecasting and modeling community recognize the value that humans bring to the field of data analytics. As Hubbard and Seiersen (2016) state: “…if the primary concern about using probabilistic methods is the lack of data, then you also lack the data to use non-quantitative methods”, (Hubbard & Seiersen, 2016, p. 38). The “human element” is that needed non-quantitative component.
There are several reasons the often biased, irrational, and poetic human will continue to contribute to the quality of the quantitative. This includes the value of the individual subject matter expert, the collective synergy of a larger sample of experts, and the innate innovativeness of man attempting to solve problems and bypass the status quo.
Discussion
Hubbard & Seiersen (2016) and Tetlock & Gardner (2016) recognized the importance of the “calibrated” subject matter expert to improve the state of predictive and quantitative analysis. Such an individual is not only proficient in their field, but also has been trained to understand a significant facet of quantitative measurement: uncertainty. Silver (2012) recognized that forecasting is not about absolute mathemetical precision, but the recognition of the importance of knowing that “[w]e must become more comfortable with probability and uncertainty,” (Silver, 2012, p. 15); the individual understands the role of uncertainty where the mathematical equation or model does not.
It is also the collective nature of human beings attempting to solve problems that will continue to improve the outcomes of predictive analysis. In 1906, the British Scientist, Sir Francis Galton, conducted an experiment where he had several hundred individuals attempt to determine the final weight of a slaughtered cow. The result was greater accuracy from the collective crowd. With remarkable precision, the average weight guessed by the participants was 1197 pounds, and in fact, the actual weight was 1198 pounds, (Tetlock & Gardner, 2016, pp. 72-73).
It was this type of “crowd sourcing” effect that demonstrated the informed and knowledgeable average of all guesses (or, predictions) culminated into a final and near accurate answer. It was this collective “hive mind” that showed how the accumulation of human knowledge brought precision to the result.
Christakis and Fowler (2009) knew that individually humans are an important component of quantified and predictive outcomes, but even greater outcomes are possible collectively. “…[C]hallenges require us to recognize that although human beings are individually powerful, we must act together to achieve what we could not accomplish on our own,” (Christakis & Fowler, 2009, p. 304). The more knowledgeable experts contributing to an estimate the greater the likelihood of accuracy less any uncertainty.
In the book, Moneyball, Billy Beane, the coach of the Oakland A’s baseball team, successfully employed the rigors of data analysis and statistics to propel his team to its historical twenty game winning streak where it was one of the worst ranked teams at the time, (Thum, 2012). The challenges were many, but he recognized that following the old models of human instinct, hunches, and guess work in selecting the best players was not going to solve his biggest problem—not having the larger payrolls that other big-league teams had to recruit these “best” players.
He also identified that baseball scouting “…was at roughly the same stage of development in the twenty-first century as professional medicine was in the eighteenth,” (Lewis, 2004, p. 17). His recruiting of Paul DePodesta, a Harvard graduate with a love of math, statistics, and baseball was a recognition that there was a need for innovation; an innovation that riled his own internal scouting staff, (Lewis, 2004, p. 17). It was this revolutionary acknowledgment of the mathematical approach brought by DePodesta that was so effective for the improvement of the Oakland A’s success on the playing field.
Conclusion
The human element will always be a vital part of the balance between the “people” and the “technology” of data analytics, and they will both contribute to improvements in predictive analysis. With the exponential growth and access to data and the power of the data science community it will bring needed value to the improvement of such analysis. The sum of the parts between man and mathematics will contribute to the growing capabilities of data analysis to lead to better predictions in the modern world.
References
(Reference Images will take you to Amazon).
Christakis, N., & Fowler, J. (2009). Connected; The surprising power of our social networks and how they shape our lives. New York: Little, Brown & Company.
Hubbard, D., & Seiersen, R. (2016). How to measure anything in cybersecurity risk. Hoboken, NJ: John wiley & sons.
Lewis, M. (2004). Moneyball: The art of winning an unfair game. New York: WW Norton & Company.
Silver, N. (2012). The signal and the noise: Why so many predictions fail–but some don’t. New York: Penguin.
Tetlock, P., & Gardner, D. (2016). Superforecasting: The art and science of prediction. New York: Random House.
Thum, W. (2012, August 19). Ten years later: The A’s 20-game winning streak, Scott Hatteberg, and Moneyball. Retrieved from SBNATION.com: https://www.sbnation.com/2012/8/19/3250200/ten-year-anniversary-athletics-20-game-winning-streak-hatteberg-moneyball
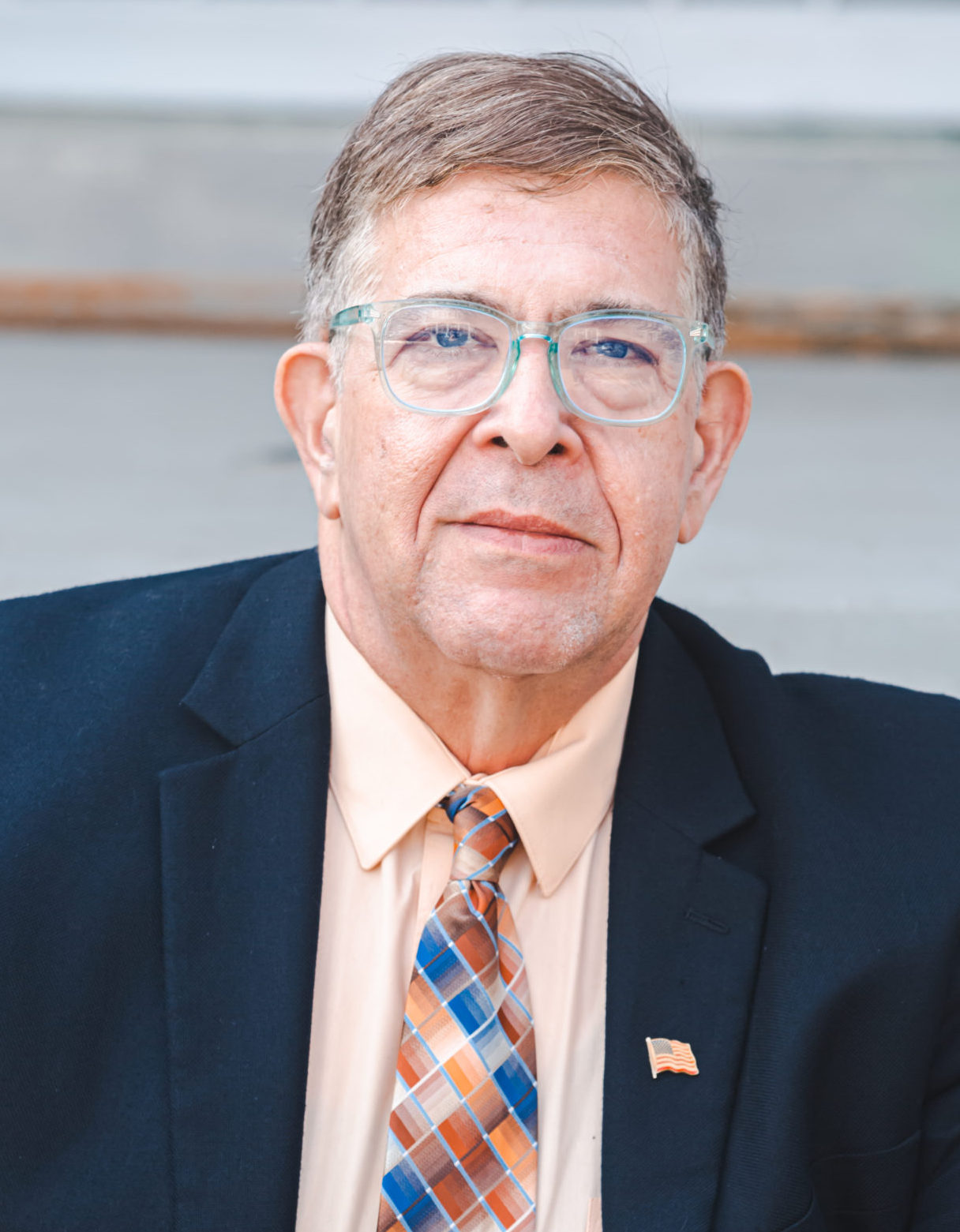
Dr. Russo is currently the Senior Data Scientist with Cybersenetinel AI in Washington, DC. He is a former Senior Information Security Engineer within the Department of Defense’s (DOD) F-35 Joint Strike Fighter program. He has an extensive background in cybersecurity and is an expert in the Risk Management Framework (RMF) and DOD Instruction 8510, which implement RMF throughout the DOD and the federal government. He holds a Certified Information Systems Security Professional (CISSP) certification and a CISSP in information security architecture (ISSAP). He has a 2017 Chief Information Security Officer (CISO) certification from the National Defense University, Washington, DC. Dr. Russo retired from the US Army Reserves in 2012 as a Senior Intelligence Officer.